Lungenkrebs
Lungenkrebs ist weltweit die häufigste krebsbedingte Todesursache und nach Brustkrebs die zweithäufigste Krebsart (Sung et al., 2021). Rauchen bleibt der primäre Risikofaktor für die Krankheit, da etwa drei Viertel der Patienten Raucher oder Ex-Raucher sind (Siegel et al., 2021). Zu den weiteren Risikofaktoren zählen die Belastung durch Passivrauchen, Umweltschadstoffe, Radon und Berufsbelastungen wie Asbest (Malhotra et al., 2016).
Geschätzte Krebstodesfälle in den USA 2022
Frauen
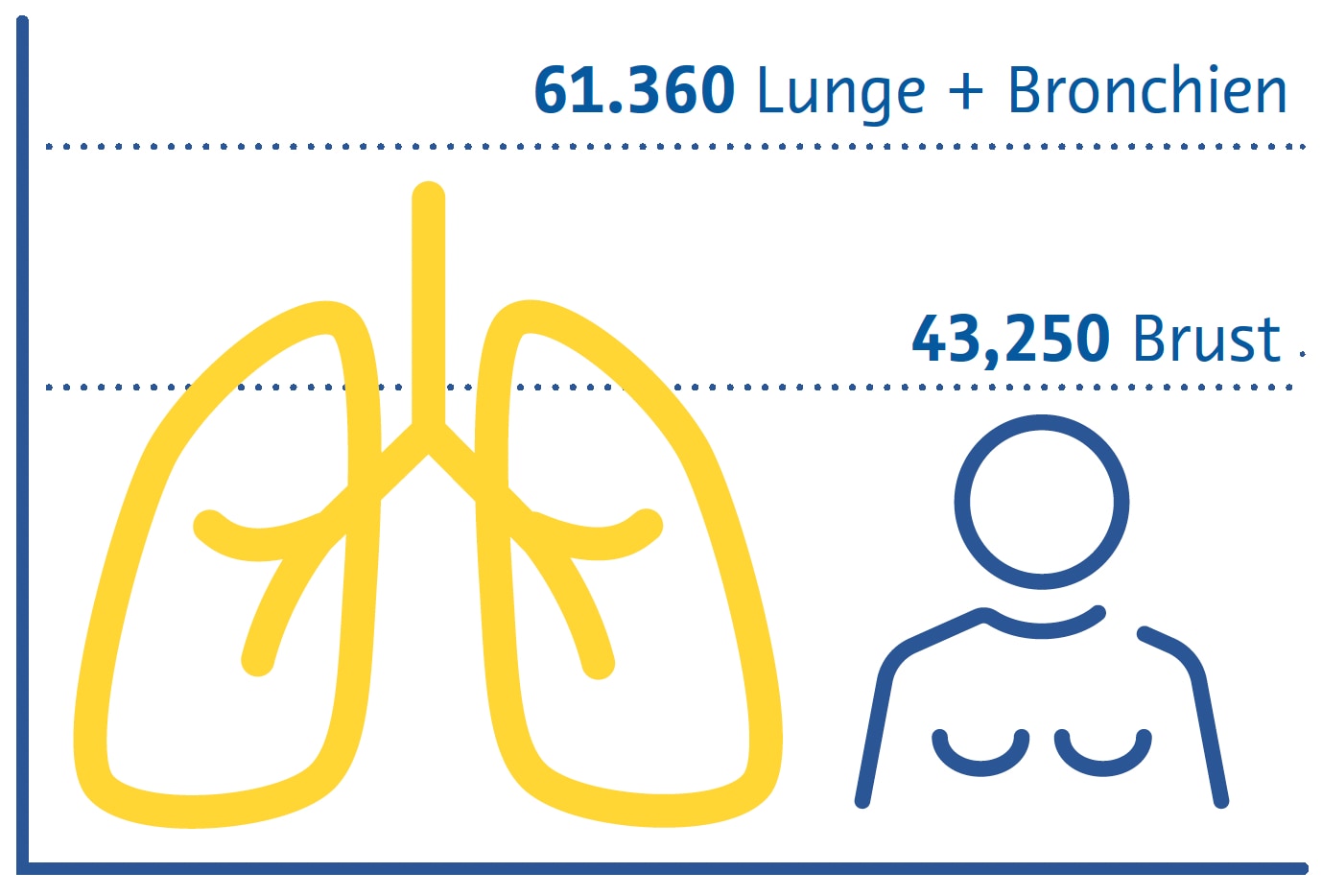
Es gibt zwei Hauptsubtypen von Lungenkrebs: nichtkleinzelligen Lungenkrebs (NSCLC) und kleinzelligen Lungenkrebs (SCLC) (Thai et al., 2021). NSCLC ist mit etwa 85 % aller Fälle am weitesten verbreitet und umfasst Adenokarzinome, Plattenepithelkarzinome und großzellige Karzinome (Thai et al., 2021). SCLC ist die aggressivere Form, die oft mit schnellem Tumorwachstum und früher Metastasierung einhergeht (Thai et al., 2021).
Die klinischen Merkmale von Lungenkrebs sind in den frühen Stadien oft unspezifisch, was zu Verzögerungen bei der Diagnose beiträgt (Hamilton et al., 2005). Zu den häufigsten Symptomen gehören anhaltender Husten, Brustschmerzen, Kurzatmigkeit und Bluthusten (Hamilton et al., 2005). Zur Diagnose von Lungenkrebs sind bildgebende Untersuchungen und eine offene „bronchoskopische“ oder radiologisch gesteuerte Gewebebiopsie erforderlich, um die Diagnose und den histologischen Subtyp zu bestätigen und Behandlungsentscheidungen zu treffen (Detterbeck et al., 2013).
Die Behandlung von Lungenkrebs hängt vom Stadium der Diagnose und den histologischen Subtypen ab (Detterbeck et al., 2013). Die primären Therapieformen sind Chirurgie, Strahlentherapie und Chemotherapie (Detterbeck et al., 2013; Thai et al., 2021). NSCLC im Frühstadium kann mit einer chirurgischen Resektion des Tumors behandelt werden, während fortgeschrittene Fälle oft eine Kombination aus Chemotherapie und Strahlentherapie erfordern (Detterbeck et al., 2013; Thai et al., 2021). Aufgrund seines aggressiven Charakters wird SCLC häufig mit Chemotherapie behandelt, manchmal in Verbindung mit Strahlentherapie (Detterbeck et al., 2013; Thai et al., 2021).
Die Immuntherapie hat sich als vielversprechende Option zur Behandlung von Lungenkrebs herausgestellt, insbesondere bei NSCLC (Thai et al., 2021; C. Wang et al., 2021). Medikamente, die auf Immun-Checkpoints abzielen, haben bei bestimmten Patienten das Überleben verbessern können (Thai et al., 2021; C. Wang et al., 2021).
Für Untergruppen von Lungenkrebspatienten wurden außerdem zielgerichtete Therapien entwickelt, die sich auf bestimmte genetische Mutationen oder Veränderungen konzentrieren und individualisierte wie auch wirksamere Behandlungsmöglichkeiten bieten (Thai et al., 2021). Um die Behandlungspläne an die individuellen Bedürfnisse der Patienten anzupassen, ist ein multidisziplinärer Ansatz unter Einbeziehung von Onkologen, Chirurgen, Radiologen und anderen medizinischen Fachkräften unerlässlich (Detterbeck et al., 2013).
Screening-Strategien
Bei den ersten klinischen Studien zum Lungenkrebs- Screening wurden Sputumanalysen und konventionelle Thorax-Röntgenaufnahmen eingesetzt. Dabei konnte kein Zusammenhang zwischen Screening und geringerer Sterblichkeit festgestellt werden (Marcus et al., 2000). Das Screening mit der Niedrigdosis-Computertomografie (CT) ist besser in der Lage, nicht verkalkte Lungenrundherde zu erkennen, die möglicherweise ein frühes Krebsstadium darstellen (Henschke et al., 1999). In nachfolgenden Studien wurde festgestellt, dass diese Form des Screenings die lungenkrebsbedingte Sterblichkeit um 20 % (National Lung Screening TriaResearch Team et. al., 2011; Aberle et. al.; 2011) und 24 % (de Koning et. al., 2020) reduziert. Obwohl die Niedrigdosis-CT teurer ist und mit einer höheren Strahlendosis als die konventionelle Radiographie verbunden ist, sind die mit der Strahlenbelastung verbundenen Risiken sehr gering (Sands et al., 2021) und große Studien haben ergeben, dass solche Screening-Strategien kosteneffektiv sind (Black et al., 2014; Toumazis et al., 2021).
Die Lungenkrebsvorsorge ist am effektivsten, wenn sie sich an Personen richtet, die ein hohes Risiko haben, an der Krebsform zu erkranken. In den aktuellen nationalen Früherkennungsrichtlinien vieler Länder werden diese Personen größtenteils anhand von Kriterien identifiziert, die aus den frühen klinischen Studien zur Lungenkrebsfrüherkennung abgeleitet wurden, z. B. Alter und Rauchverhalten in Packungsjahren. Forscher in anderen Ländern haben mathematische Modelle zur Schätzung des Lungenkrebsrisikos entwickelt, um die Eignung für die Vorsorgeuntersuchung zu bestimmen, indem sie zusätzliche Variablen wie ethnische Herkunft, persönliche und familiäre Lungenkrebsanamnese und den Body-Mass-Index einbeziehen (Cassidy et al., 2008; Field et al., 2016; Tammemägi et al., 2013).
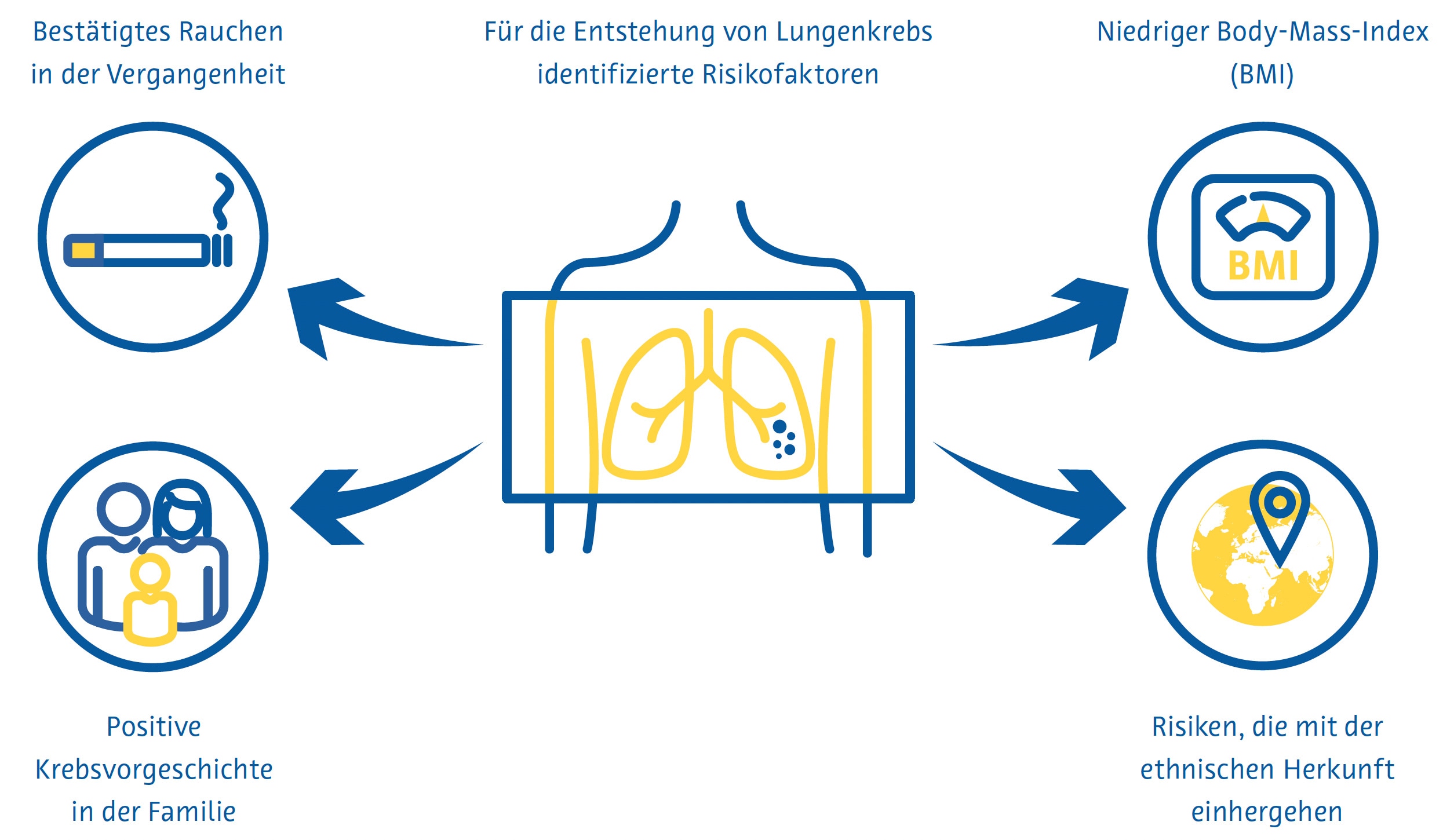
Herausforderungen beim Screening
Trotz eindeutiger Belege für die Wirksamkeit des Lungenkrebs-Screenings birgt die praktische Umsetzung mehrere Herausforderungen. Die Teilnahme an Lungenkrebs-Screeningprogrammen und die kontinuierliche Einhaltung dieser Programme sind gering. In den USA beträgt die Teilnahmequote nur 7–14 % (J. Li et al., 2018; Zahnd & Eberth, 2019). Auch in Großbritannien sind die Quoten niedrig, insbesondere unter denjenigen, die am stärksten von Lungenkrebs bedroht sind (Ali et al., 2015). Da immer mehr Länder nationale Lungenkrebs-Screeningprogramme einführen, könnte der bereits jetzt gravierende weltweite Mangel an Radiologen (AAMC-Bericht bestätigt zunehmenden Ärztemangel, 2021; Smieliauskas et al., 2014; The Royal College of Radiologists, 2022) das Lungenkrebs- Screening weiter erschweren, da es weniger qualifizierte Radiologen geben wird, die eine steigende Zahl von CT-Untersuchungen auswerten können.
Dies ist insbesondere deshalb problematisch, weil die Berichterstellung von Lungenkrebs- Vorsorgeuntersuchungen besondere Fachkenntnisse und Schulungen erfordert (LCS-Projekt, o. J.). Darüber hinaus geht ein höherer Berichterstellungsaufwand im Allgemeinen mit mehr Fehlern seitens der Radiologen einher (Hanna et al., 2018), was zu schlechteren Screeningergebnissen führen kann. Auch über den Umgang mit Zufallsbefunden, die im Rahmen einer Lungenkrebsvorsorge festgestellt werden, besteht keine Einigkeit. Diese Befunde führen bei bis zu 15 % der gescreenten Patienten zu einer weiterführenden Diagnostik (Morgan et al., 2017) und sind mit erhöhter Angst der Patienten und Kosten für das Gesundheitssystem verbunden (Adams et al., 2016).
Rolle der künstlichen Intelligenz
Identifizierung von Personen mit hohem Risiko
Die von den Centers for Medicare and Medicaid Services (CMS) in den USA festgesetzten Zulassungskriterien für das Lungenkrebs-Screening berücksichtigen die Hälfte aller Lungenkrebsfälle (Y. Wang et al., 2015) nicht. Diese Kriterien, die nur die Raucheranamnese und das Alter berücksichtigen, liefern eine suboptimale Risikovorhersage, da sie andere wichtige Risikofaktoren ignorieren (Burzic et al., 2022) und oft auf ungenauen oder nicht verfügbaren Daten basieren (Kinsinger et al., 2017). Dies ist insbesondere deshalb wichtig, weil etwa ein Viertel aller Lungenkrebsfälle nicht auf das Rauchen zurückzuführen sind (Sun et al., 2007).
Aus diesem Grund wurde in Studien der Einsatz künstlicher Intelligenz (KI) mit dem Ziel untersucht, die Vorhersage des Lungenkrebsrisikos zu verbessern und mehr Menschen in Screening-Programme einzubeziehen. Die Kombination von Informationen aus elektronischen Krankenakten und konventionellen Röntgenaufnahmen des Brustkorbs mithilfe eines Convolutional Neural Network (CNN) zeigte, dass Lungenkrebs über einen Zeitraum von 12 Jahren besser vorhergesagt werden konnte als bestehende Screening-Kriterien. Zudem wurde eine um 31 % niedrigere Zahl an übersehenen Lungenkrebsfällen erreicht (Lu et al., 2020). Eine andere Studie mit einem ähnlichen Ansatz kam zu dem Ergebnis, dass Patienten, die sowohl anhand konventioneller Thorax-Röntgenbilder als auch anhand der CMS-Kriterien als Hochrisikopatienten für Lungenkrebs eingestuft wurden, ohne jedoch ausschließlich die CMS-Kriterien anzuwenden, eine 6-Jahres-Inzidenz von Lungenkrebs von 3,3 % aufwiesen (Raghu et al., 2022). Dies liegt weit über dem 6-Jahres-Risikoschwellenwert für das Lungenkrebs-Screening von 1,3 %, der dem von der USPSTF (United States Preventative Services Task Force) verwendeten Schwellenwert ähnelt (Wood et al., 2018).
Reduzierung der Strahlendosis und Verbesserung der Bildqualität
Zur Bildglättung können Deep-Learning-Techniken eingesetzt werden, wobei einige Lösungen für die Thorax-CT bereits auf dem Markt erhältlich sind (Nam et al., 2021). Die auf Deep Learning basierende Bildrekonstruktion der Ultra-Low-Dose-CT erhöhte die Lungenrundherd-Erkennungsrate und verbesserte die Lungenrundherd-Messgenauigkeit im Vergleich zu herkömmlichen Rekonstruktionsalgorithmen (Jiang et al., 2022). Die auf Deep Learning basierende Rauschunterdrückung der Ultra-Low-Dose-CT zeigte auch eine bessere subjektive Bildqualität als die Ultra-Low- Dose-CT ohne Rauschunterdrückung (Hata et al., 2020).
Erkennung von Lungenrundherden
Lungenrundherde sind definiert als eine kleine (normalerweise weniger als 3 Zentimeter), runde oder unregelmäßige Masse im Lungengewebe, die infektiös, entzündlich, angeboren oder neoplastisch sein kann (Wyker & Henderson, 2022). Die Erkennung von Lungenrundherde durch Radiologen ist zeitaufwändig und fehleranfällig, wie etwa die Nichtidentifizierung oder falsche Identifizierung potenziell bösartiger Lungenrundherde (Al Mohammad et al., 2019; Armato et al., 2009; Gierada et al., 2017; Leader et al., 2005).
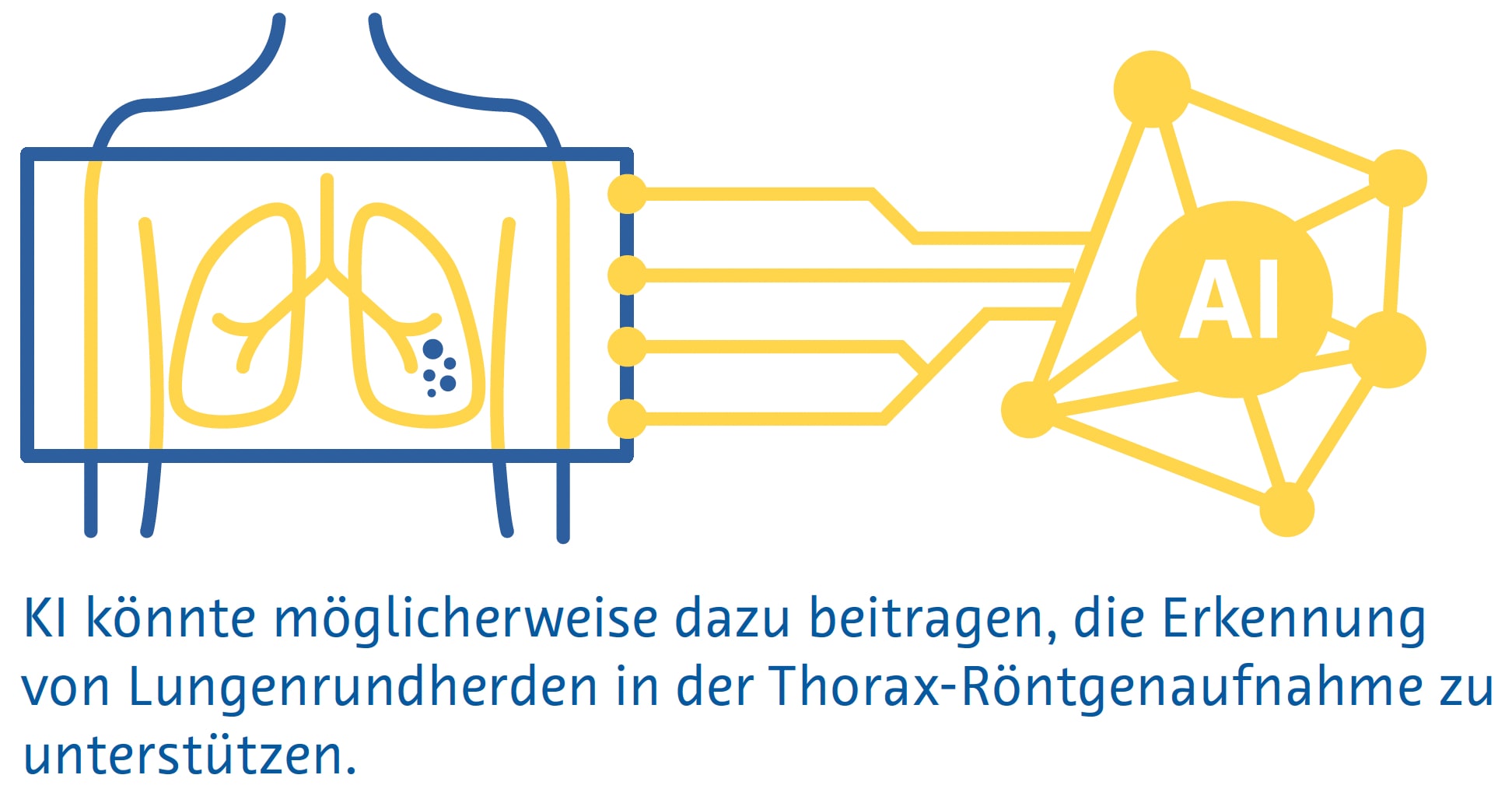
Kleine Lungenrundherde sind auf herkömmlichen Röntgenbildern des Brustbereichs häufig nicht sichtbar, und selbst große Lungenrundherde können von Radiologen auf Röntgenbildern übersehen werden (Austin et al., 1992). Trotzdem wird das Potenzial der KI zur Erkennung von Lungenrundherden und Lungenkrebs auf Röntgenaufnahmen des Brustkorbs aktiv erforscht (Cha et al., 2019; Homayounieh et al., 2021; Jones et al., 2021; X. Li et al., 2020; Mendoza & Pedrini, 2020; Nam et al., 2019; Yoo et al., 2021), da die Methode als bildgebende Erstuntersuchung bei Atemwegs- und Herzerkrankungen eingesetzt wird und weit verbreitet und kostengünstig ist (Ravin & Chotas, 1997).
Eine Metaanalyse von neun Studien ergab eine AUC von 0,884 für die Erkennung von Lungenrundherden auf konventionellen Thorax-Röntgenbildern mittels KI (Aggarwal et al., 2021).
Eine Metaanalyse von 41 Studien ergab eine Sensitivität von 55–99 % für traditionelles maschinelles Lernen und 80–97 % für Deep-Learning-Algorithmen zur Identifizierung von Lungenrundherden in der Niedrigdosis-CT (Pehrson et al., 2019). Eine der ersten Studien, die Deep Learning zur Erkennung von Lungenrundherden bei der Niedrigdosis-CT einsetzte, ergab eine Sensitivität von 98,3 % und ein falschpositives Ergebnis pro Untersuchung unter Verwendung einer Kombination verschiedener Algorithmen (Setio et al., 2017). Falsch positive Ergebnisse betreffen in der Regel Blutgefäße, Narbengewebe oder Abschnitte der Brustwand, der Wirbel oder des Mediastinalgewebes (Cui et al., 2022; L. Li et al., 2019; Setio et al., 2017).
Eine Metaanalyse, die 56 Studien umfasste, ergab eine AUC von 0,94 für die Lungenrundherderkennung im CT mittels Deep Learning (Aggarwal et al., 2021). Allerdings verwendeten nur sehr wenige dieser Studien prospektiv erhobene Daten oder validierten die Algorithmen anhand eines unabhängigen externen Datensatzes (Aggarwal et al., 2021). Ein Deep-Learning-Algorithmus, der anhand von über 10.000 Niedrigdosis-Thorax-CTs trainiert wurde, erreichte bei Tests anhand von drei externen Validierungsdatensätzen nach einem Jahr unter Verwendung der Histopathologie als Referenzstandard eine AUC von 0,86–0,94 für die Lungenkrebsvorhersage (Mikhael et al., 2023).
Eine Studie mit 346 Personen, die an einem Lungenkrebs- Screening-Programm teilnahmen, ergab, dass ein Deep- Learning-Algorithmus eine höhere Sensitivität für Lungenrundherde aufwies als eine Doppelbefundung durch zwei auf Thoraxbildgebung spezialisierte Radiologen (86 % gegenüber 79 %), aber eine viel höhere Falscherkennungsrate (1,53 gegenüber 0,13 pro Scan) (L. Li et al., 2019). Eine ähnliche Studie mit 360 Personen ergab, dass ein Deep-Learning-Algorithmus Lungenrundherde bei einer Niedrigdosis-CT mit einer Sensitivität von 90 % und einer Falscherkennungsrate von 1 pro Scan erkannte, im Vergleich zu einer Sensitivität von 76 % und eine Falscherkennungsrate von 0,04 pro Scan, wenn die Untersuchungen von Paaren, bestehend aus einem Junior- und einem Senior-Radiologen doppelt gelesen wurden (Cui et al., 2022). Der Unterschied in der Sensitivität zwischen dem Algorithmus und den Radiologen war besonders groß bei Lungenrundherden mit einem Durchmesser zwischen 4 und 6 mm (86 % gegenüber 59 %) (Cui et al., 2022).
Lungenrundherdsegmentierung
Die genaue Messung von Lungenrundherden ist wichtig, um das Lungenrundherdwachstum im Laufe der Zeit zu überwachen und die Behandlung dieser Läsionen zu steuern (Bankier et al., 2017). Allerdings beträgt der geschätzte Fehler bei der manuellen Messung des Lungenrundherd-Durchmesser etwa 1,5 mm, was bei kleinen Lungenrundherden eine erhebliche Fehleinschätzung der Größe darstellt (Bankier et al., 2017; Revel et al., 2004). Darüber hinaus spiegelt der Lungenrundherd-Durchmesser das Lungenrundherdwachstum unter Umständen nicht genau wider, weil man davon ausgeht, dass alle Lungenrundherde perfekte Kugeln sind (Devaraj et al., 2017). In diesem Zusammenhang beinhaltete die neueste Version von LungRADS 2022 die Möglichkeit, eine volumetrische ( mm3 ) Bewertung durchzuführen.
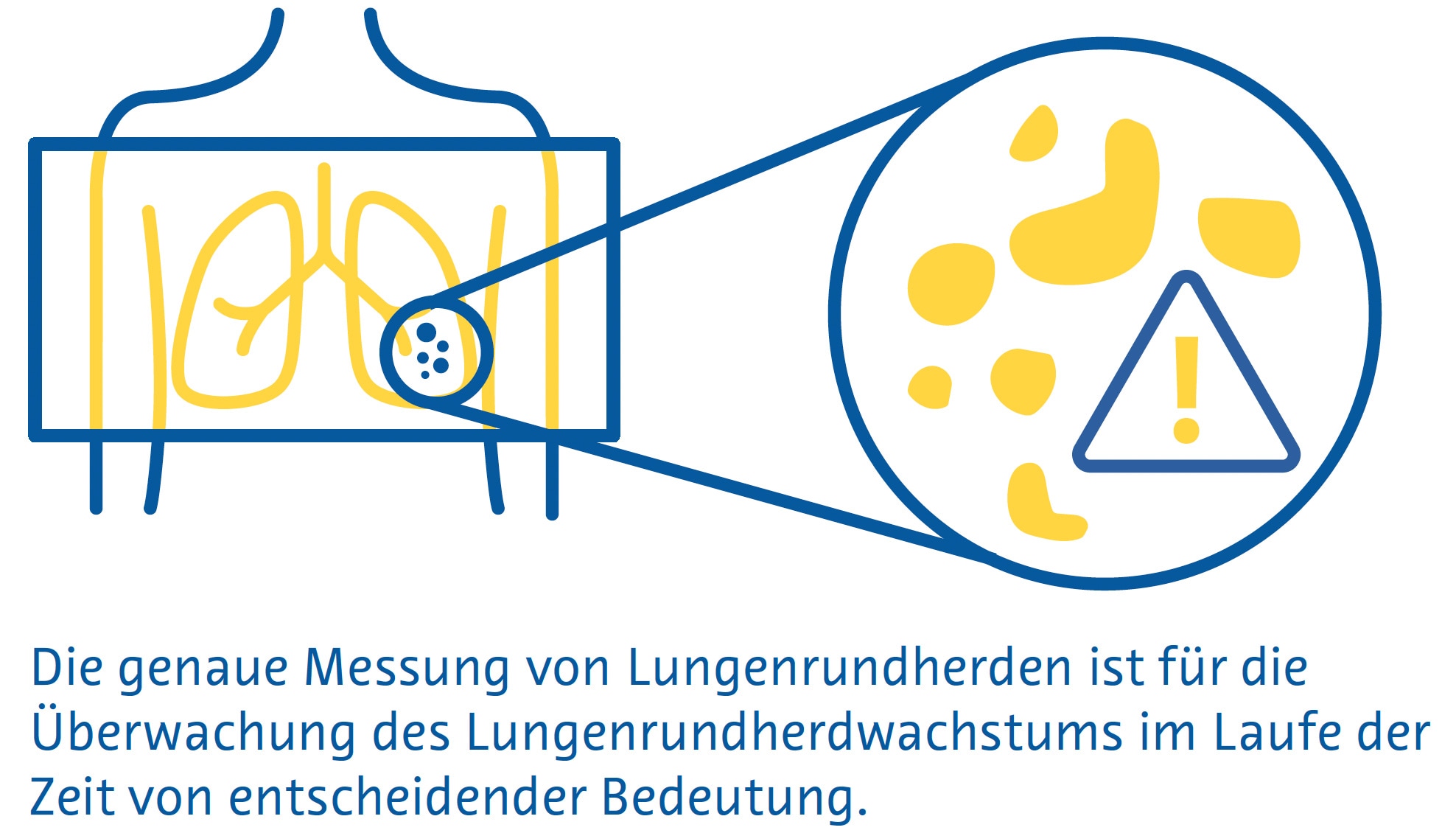
Die Segmentierung von Lungenrundherden ermöglicht eine genaue Schätzung ihres Volumens und ist ein mehrstufiger Prozess, der die Lungenrundherderkennung, einen „Region-Growing“-Prozess, bei dem die Grenzen der Lungenrundherde durch Ausnutzung von Unterschieden in der Gewebeschwächung zwischen dem Lungenrundherd und dem umgebenden Lungenparenchym identifiziert werden, und die Entfernung umgebender Strukturen mit ähnlicher Schwächung, wie etwa Blutgefäße, umfasst (Devaraj et al., 2017).
Einfache Segmentierungsansätze, die stark auf den Unterschieden in der Abschwächung zwischen Lungenrundherd und dem umgebenden Lungenparenchym beruhen, weisen bei juxtavaskulären und subsoliden Lungenrundherden eine schlechte Leistung auf (Devaraj et al., 2017). CNNs, insbesondere Encoder-Decoder-Algorithmen, erzielen dagegen mit Dice-Ähnlichkeitskoeffizienten (ein Maß für räumliche Überlappung) von 0,79 bis 0,93 eine deutlich bessere Segmentierungsleistung im Vergleich zu Ground-Truth- Segmentierungen durch Radiologen (Dong et al., 2020; Gu et al., 2021). Es sind mehrere KI-basierte Algorithmen zur Lungenrundherdsegmentierung auf dem Markt, die eine automatisierte Lungenrundherd-volumenmessung und Längsvolumenverfolgung durchführen (Hwang et al., 2021; Jacobs et al., 2021; Murchison et al., 2022; Park et al., 2019; Röhrich et al., 2023; Singh et al., 2021).
Klassifizierung von Lungenrundherden
Bei der Bestimmung der Wahrscheinlichkeit, dass es sich bei einem Lungenrundherd um Krebs handelt, werden folgende Faktoren berücksichtigt: Größe, Form, Zusammensetzung, Lage sowie ob und wie es sich im Laufe der Zeit verändert (Callister et al., 2015; Lung Rads, nd). Studien haben eine mäßige Inter- und Intraobserver-Übereinstimmung zwischen Radiologen hinsichtlich der Merkmale gezeigt, die zur Schätzung der Wahrscheinlichkeit verwendet werden, dass ein Lungenrundherd bösartig ist, wobei bei über einem Drittel der Lungenrundherde eine abweichende Klassifizierung vorliegt (van Riel et al., 2015). Derzeit sind mehrere auf dem Markt erhältliche KI-basierte Lösungen zur Klassifizierung von Lungenrundherden verfügbar, von denen viele eine Bewertung des Malignitätsrisikos beinhalten (Adams et al., 2023; Hwang et al., 2021; Murchison et al., 2022; Park et al., 2019; Röhrich et al., 2023).
Ein Deep-Learning-Algorithmus, der mit Daten von 943 Patienten trainiert und an einem unabhängigen Datensatz von 468 Patienten validiert wurde, zeigte eine Gesamtgenauigkeit von 78–80 % für die Klassifizierung von Lungenrundherde in eine von 6 Kategorien (solid, kalzifiziert, teilsolid, nicht solid, perifissural oder spikuliert). (Ciompi et al., 2017). Die Genauigkeit war bei teilsoliden, spikulierten und perifissuralen Lungenrundherden am geringsten (Ciompi et al., 2017).
Ein Deep-Learning-Algorithmus, der an 6716 Niedrigdosis- CTs getestet und an einem unabhängigen Datensatz von 1139 Niedrigdosis-CTs validiert wurde, zeigte eine AUC von 0,94 für die Vorhersage des Lungenkrebsrisikos unter Verwendung der Histopathologie als Referenzstandard (Ardila et al., 2019). Sofern verfügbar, bezieht der Algorithmus Informationen aus früheren CTs desselben Patienten mit ein. In diesen Fällen war seine Leistung mit der von sechs Radiologen vergleichbar (Ardila et al., 2019). Wenn keine vorherige Bildgebung verfügbar war, hatte der Algorithmus eine um 11 % niedrigere Falsch-Positiv-Rate und eine um 5 % niedrigere Falsch Negativ-Rate als die Radiologen (Ardila et al., 2019).
In einer anderen Studie wurde ein multitaskingfähiges dreidimensionales CNN verwendet, um Lungenrundherd- Merkmale wie Verkalkung, Lobulation, Sphärizität, Spikulation, Ränder und Textur mit einer Genauigkeit von 91,3 % zu extrahieren (Hussein et al., 2017).
Die Studie nutzte das Transferlernen eines Algorithmus, der anhand von einer Million Videos trainiert wurde, und testete ihn anhand eines Datensatzes von über 1.000 CT-Scans des Brustbereichs. Der Referenzstandard bestand aus Malignitäts-Scores und Lungenrundherdcharakterisitk-Scores, die von mindestens drei Radiologen ermittelt wurden (Hussein et al., 2017). In einer anderen Studie wurden mithilfe eines multitaskingfähigen 3D-CNN, das auf demselben Datensatz ohne Transferlernen getestet wurde, gleichzeitig Lungenrundherde segmentiert, die Wahrscheinlichkeit einer bösartigen Erkrankung vorhergesagt und beschreibende Lungenrundherdattribute ausgegeben. Dabei wurde ein Dice-Ähnlichkeitskoeffizient von 0,74 und eine „Off-byone“- Genauigkeit von 97,6 % erreicht. (Wu et al., 2018).
Herausforderungen und zukünftige Wege
Auf der Grundlage aller verfügbaren Belege haben einige Länder damit begonnen, KI in ihre nationalen Screening-Programme zu integrieren. Erwähnenswert ist in diesem Zusammenhang das kürzlich vom deutschen Bundesjustizministerium veröffentlichte Bundesgesetz, das den Einsatz einer Software zur computergestützten Lungenrundherderkennung im neuen Lungenkrebs-Screening-Programm, zur Erkennung und Volumetrie von Lungenrundherden, zur Ermittlung der Volumenverdopplungszeit und zur Speicherung der Auswertung für eine strukturierte Befundung vorsieht ( https://www.recht.bund.de/bgbl/1/2024/162/VO.html ).
Trotz der sehr ermutigenden Ergebnisse, die in den letzten Jahren bei der Verbesserung des Lungenkrebs- Screenings durch künstliche Intelligenz erzielt wurden, bleiben einige wichtige methodische Herausforderungen bestehen.
Die schiere Heterogenität der bisher durchgeführten Studien erschwert eine Evidenzsynthese durch Metaanalyse (Aggarwal et al., 2021). Darüber hinaus ist unklar, wie generalisierbar diese Algorithmen sind, da es der Mehrzahl der Studien an einer soliden externen Validierung mangelt (Aggarwal et al., 2021). Darüber hinaus muss der Zusatznutzen einiger dieser Algorithmen über die Verbesserung der Diagnoseleistung hinaus untersucht werden, etwa im Hinblick auf eine höhere Effizienz oder geringere Kosten (National Institute for Health and Care Excellence (NICE), o. J.).
Subsolide Lungenrundherde, einschließlich milchglasartiger Lungenrundherde, sind mit höherer Wahrscheinlichkeit bösartig als solide Lungenrundherde (Henschke et al., 2002). Da die Unterschiede in der Abschwächung zwischen diesen Lungenrundherden und dem umgebenden Lungenparenchym jedoch sehr gering sind, ist ihre Erkennung besonders schwierig (de Margerie-Mellon & Chassagnon, 2023; L. Li et al., 2019; Setio et al., 2017). Einige automatisierte Algorithmen haben sich bei der Erkennung subsolider Lungenrundherde als vielversprechend erwiesen, müssen jedoch noch umfassend validiert werden (Qi et al., 2020, 2021).
Fazit
Der Einsatz künstlicher Intelligenz könnte dazu beitragen, mehr Personen mit hohem Lungenkrebsrisiko zu identifizieren und die Qualität der Vorsorgeuntersuchungen zu verbessern. KI hat sich als besonders nützlich erwiesen, um potenziell bösartige Lungenrundherde zu identifizieren und zu segmentieren, wobei ihre Sensitivität oft die von Radiologen übertrifft (Cui et al., 2022; L. Li et al., 2019). Auch bei der Einschätzung der Wahrscheinlichkeit einer malignen Lungenrundherderkrankung hat sich die Methode als vielversprechend erwiesen, insbesondere wenn vorherige bildgebende Verfahren verfügbar sind.
Zukünftige Forschung sollte sich mit den Nachteilen früherer Studien befassen, zu denen unter anderem das Fehlen einer soliden externen Validierung und die Vernachlässigung von Effizienz- und Kostenergebnissen gehören. So soll der Weg für eine frühere Diagnose und bessere Behandlung von Lungenkrebs geebnet werden.
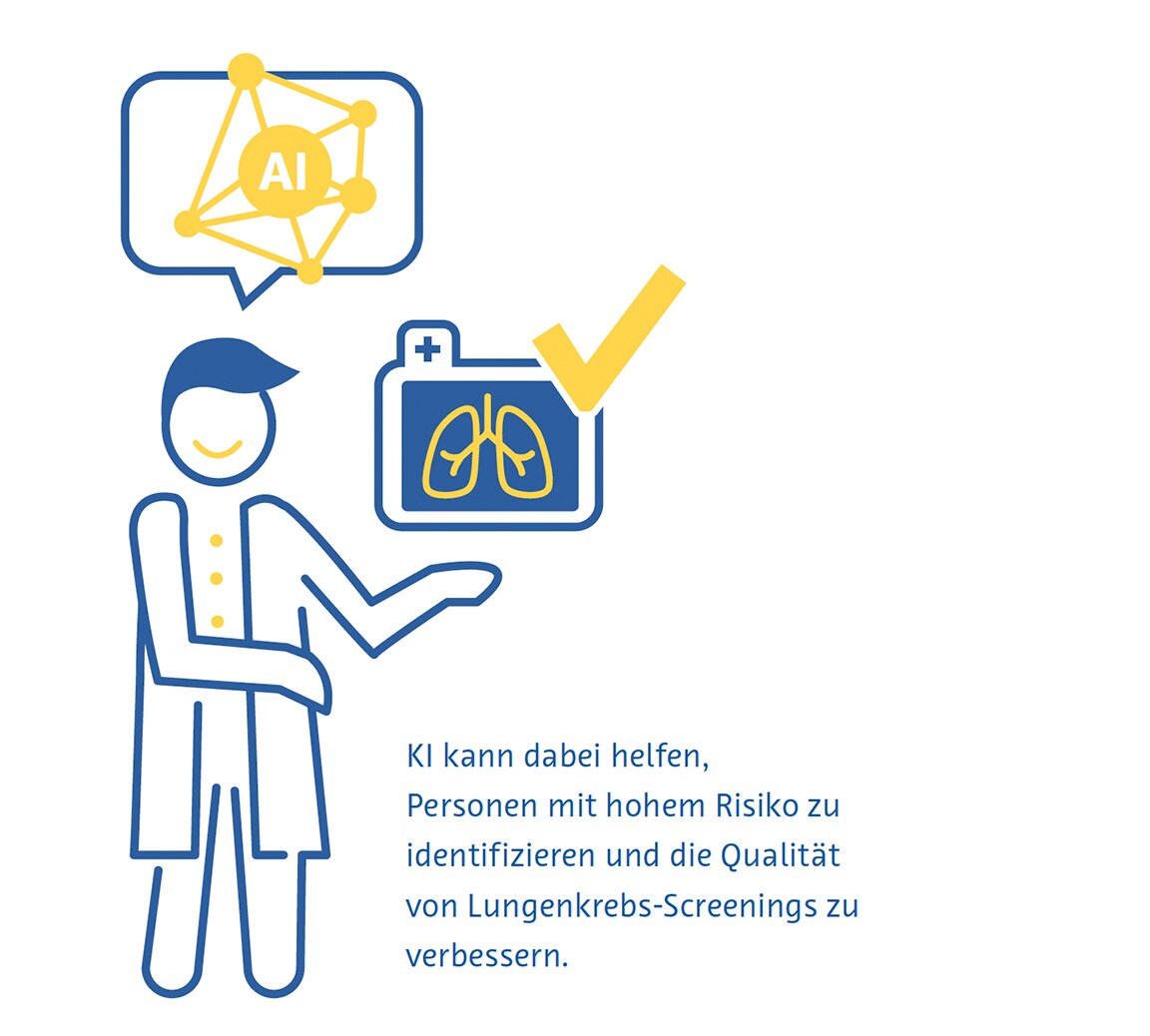
Literaturverzeichnis
AAMC Report Reinforces Mounting Physician Shortage. (2021). AAMC. https://www.aamc.org/news-insights/press-releases/aamcreport- reinforces-mounting-physician-shortage, accessed on 26.09.2024
Adams, S. J., Babyn, P. S., & Danilkewich, A. (2016). Toward a comprehensive management strategy for incidental findings in imaging. Canadian Family Physician Medecin de Famille Canadien, 62(7), 541–543.
Adams, S. J., Madtes, D. K., Burbridge, B., Johnston, J., Goldberg, I. G., Siegel, E. L., Babyn, P., Nair, V. S., & Calhoun, M. E. (2023). Clinical Impact and Generalizability of a Computer-Assisted Diagnostic Tool to Risk-Stratify Lung Nodules With CT. Journal of the American College of Radiology: JACR, 20(2), 232–242. https:// doi.org/10.1016/j.jacr.2022.08.006
Aggarwal, R., Farag, S., Martin, G., Ashrafian, H., & Darzi, A. (2021). Patient Perceptions on Data Sharing and Applying Artificial Intelligence to Health Care Data: Cross-sectional Survey. Journal of Medical Internet Research, 23(8), e26162. https://doi.org/10.2196/26162
Aggarwal, R., Sounderajah, V., Martin, G., Ting, D. S. W., Karthikesalingam, A., King, D., Ashrafian, H., & Darzi, A. (2021). Diagnostic accuracy of deep learning in medical imaging: a systematic review and meta-analysis. NPJ Digital Medicine, 4(1), 65. https://doi.org/10.1038/s41746-021-00438-z
Ali, N., Lifford, K. J., Carter, B., McRonald, F., Yadegarfar, G., Baldwin, D. R., Weller, D., Hansell, D. M., Duffy, S. W., Field, J. K., & Brain, K. (2015). Barriers to uptake among high-risk individuals declining participation in lung cancer screening: a mixed methods analysis of the UK Lung Cancer Screening (UKLS) trial. BMJ Open, 5(7), e008254. https://doi.org/10.1136/ bmjopen-2015-008254
Al Mohammad, B., Hillis, S. L., Reed, W., Alakhras, M., & Brennan, P. C. (2019). Radiologist performance in the detection of lung cancer using CT. Clinical Radiology, 74(1), 67–75. https://doi.org/10.1016/j.crad.2018.10.008
Ardila, D., Kiraly, A. P., Bharadwaj, S., Choi, B., Reicher, J. J., Peng, L., Tse, D., Etemadi, M., Ye, W., Corrado, G., Naidich, D. P., & Shetty, S. (2019). End-to-end lung cancer screening with three-dimensional deep learning on low-dose chest computed tomography. Nature Medicine, 25(6), 954–961. https://doi.org/10.1038/s41591-019-0447-x
Armato, S. G., 3rd, Roberts, R. Y., Kocherginsky, M., Aberle, D. R., Kazerooni, E. A., Macmahon, H., van Beek, E. J. R., Yankelevitz, D., McLennan, G., McNitt-Gray, M. F., Meyer, C. R., Reeves, A. P., Caligiuri, P., Quint, L. E., Sundaram, B., Croft, B. Y., & Clarke, L. P. (2009). Assessment of radiologist performance in the detection of lung nodules: dependence on the definition of “truth.” Academic Radiology, 16(1), 28–38. https://doi.org/10.1016/j.acra.2008.05.022
Austin, J. H., Romney, B. M., & Goldsmith, L. S. (1992). Missed bronchogenic carcinoma: radiographic findings in 27 patients with a potentially resectable lesion evident in retrospect. Radiology, 182(1), 115–122. https://doi.org/10.1148/radiology.182.1.1727272
Bankier, A. A., MacMahon, H., Goo, J. M., Rubin, G. D., Schaefer- Prokop, C. M., & Naidich, D. P. (2017). Recommendations for Measuring Pulmonary Nodules at CT: A Statement from the Fleischner Society. Radiology, 285(2), 584–600. https://doi. org/10.1148/radiol.2017162894
Black, W. C., Gareen, I. F., Soneji, S. S., Sicks, J. D., Keeler, E. B., Aberle, D. R., Naeim, A., Church, T. R., Silvestri, G. A., Gorelick, J., Gatsonis, C., & National Lung Screening Trial Research Team. (2014). Cost-effectiveness of CT screening in the National Lung Screening Trial. The New England Journal of Medicine, 371(19), 1793–1802. https://doi.org/10.1056/NEJMoa1312547
Burzic, A., O’Dowd, E. L., & Baldwin, D. R. (2022). The Future of Lung Cancer Screening: Current Challenges and Research Priorities. Cancer Management and Research, 14, 637–645. https://doi.org/10.2147/CMAR.S293877
Callister, M. E. J., Baldwin, D. R., Akram, A. R., Barnard, S., Cane, P., Draffan, J., Franks, K., Gleeson, F., Graham, R., Malhotra, P., Prokop, M., Rodger, K., Subesinghe, M., Waller, D., Woolhouse, I., British Thoracic Society Pulmonary Nodule Guideline Development Group, & British Thoracic Society Standards of Care Committee. (2015). British Thoracic Society guidelines for the investigation and management of pulmonary nodules. Thorax, 70 Suppl 2, ii1–ii54. https://doi.org/10.1136/thoraxjnl-2015-207168
Cassidy, A., Myles, J. P., van Tongeren, M., Page, R. D., Liloglou, T., Duffy, S. W., & Field, J. K. (2008). The LLP risk model: an individual risk prediction model for lung cancer. British Journal of Cancer, 98(2), 270–276. https://doi.org/10.1038/sj.bjc.6604158
Cha, M. J., Chung, M. J., Lee, J. H., & Lee, K. S. (2019). Performance of Deep Learning Model in Detecting Operable Lung Cancer With Chest Radiographs. Journal of Thoracic Imaging, 34(2), 86–91. https://doi.org/10.1097/RTI.0000000000000388
Ciompi, F., Chung, K., van Riel, S. J., Setio, A. A. A., Gerke, P. K., Jacobs, C., Scholten, E. T., Schaefer-Prokop, C., Wille, M. M. W., Marchianò, A., Pastorino, U., Prokop, M., & van Ginneken, B. (2017). Towards automatic pulmonary nodule management in lung cancer screening with deep learning. Scientific Reports, 7, 46479. https://doi.org/10.1038/srep46479
Cui, X., Zheng, S., Heuvelmans, M. A., Du, Y., Sidorenkov, G., Fan, S., Li, Y., Xie, Y., Zhu, Z., Dorrius, M. D., Zhao, Y., Veldhuis, R. N. J., de Bock, G. H., Oudkerk, M., van Ooijen, P. M. A., Vliegenthart, R., & Ye, Z. (2022). Performance of a deep learning-based lung nodule detection system as an alternative reader in a Chinese lung cancer screening program. European Journal of Radiology, 146, 110068. https://doi.org/10.1016/j. ejrad.2021.110068
de Koning, H. J., van der Aalst, C. M., de Jong, P. A., Scholten, E. T., Nackaerts, K., Heuvelmans, M. A., Lammers, J.-W. J., Weenink, C., Yousaf-Khan, U., Horeweg, N., van ’t Westeinde, S., Prokop, M., Mali, W. P., Mohamed Hoesein, F. A. A., van Ooijen, P. M. A., Aerts, J. G. J. V., den Bakker, M. A., Thunnissen, E., Verschakelen, J., … Oudkerk, M. (2020). Reduced Lung-Cancer Mortality with Volume CT Screening in a Randomized Trial. The New England Journal of Medicine, 382(6), 503–513. https://doi. org/10.1056/NEJMoa1911793
de Margerie-Mellon, C., & Chassagnon, G. (2023). Artificial intelligence: A critical review of applications for lung nodule and lung cancer. Diagnostic and Interventional Imaging, 104(1), 11–17. https://doi.org/10.1016/j.diii.2022.11.007
Detterbeck, F. C., Lewis, S. Z., Diekemper, R., Addrizzo-Harris, D., & Alberts, W. M. (2013). Executive Summary: Diagnosis and management of lung cancer, 3rd ed: American College of Chest Physicians evidence-based clinical practice guidelines. Chest, 143(5 Suppl), 7S – 37S. https://doi.org/10.1378/chest.12-2377
Devaraj, A., van Ginneken, B., Nair, A., & Baldwin, D. (2017). Use of Volumetry for Lung Nodule Management: Theory and Practice. Radiology, 284(3), 630–644. https://doi.org/10.1148/ radiol.2017151022
Dong, X., Xu, S., Liu, Y., Wang, A., Saripan, M. I., Li, L., Zhang, X., & Lu, L. (2020). Multi-view secondary input collaborative deep learning for lung nodule 3D segmentation. Cancer Imaging: The Official Publication of the International Cancer Imaging Society, 20(1), 53. https://doi.org/10.1186/s40644-020-00331-0
Field, J. K., Duffy, S. W., Baldwin, D. R., Whynes, D. K., Devaraj, A., Brain, K. E., Eisen, T., Gosney, J., Green, B. A., Holemans, J. A., Kavanagh, T., Kerr, K. M., Ledson, M., Lifford, K. J., McRonald, F. E., Nair, A., Page, R. D., Parmar, M. K. B., Rassl, D. M., … Hansell, D. M. (2016). UK Lung Cancer RCT Pilot Screening Trial: baseline findings from the screening arm provide evidence for the potential implementation of lung cancer screening. Thorax, 71(2), 161–170. https://doi.org/10.1136/thoraxjnl-2015-207140
Gierada, D. S., Pinsky, P. F., Duan, F., Garg, K., Hart, E. M., Kazerooni, E. A., Nath, H., Watts, J. R., Jr, & Aberle, D. R. (2017). Interval lung cancer after a negative CT screening examination: CT findings and outcomes in National Lung Screening Trial participants. European Radiology, 27(8), 3249–3256. https://doi. org/10.1007/s00330-016-4705-8
Gu, D., Liu, G., & Xue, Z. (2021). On the performance of lung nodule detection, segmentation and classification. Computerized Medical Imaging and Graphics: The Official Journal of the Computerized Medical Imaging Society, 89, 101886. https://doi. org/10.1016/j.compmedimag.2021.101886
Hamilton, W., Peters, T. J., Round, A., & Sharp, D. (2005). What are the clinical features of lung cancer before the diagnosis is made? A population based case-control study. Thorax, 60(12), 1059–1065. https://doi.org/10.1136/thx.2005.045880
Hanna, T. N., Lamoureux, C., Krupinski, E. A., Weber, S., & Johnson, J.-O. (2018). Effect of Shift, Schedule, and Volume on Interpretive Accuracy: A Retrospective Analysis of 2.9 Million Radiologic Examinations. Radiology, 287(1), 205–212. https://doi. org/10.1148/radiol.2017170555
Hata, A., Yanagawa, M., Yoshida, Y., Miyata, T., Tsubamoto, M., Honda, O., & Tomiyama, N. (2020). Combination of Deep Learning-Based Denoising and Iterative Reconstruction for Ultra-Low-Dose CT of the Chest: Image Quality and Lung-RADS Evaluation. AJR. American Journal of Roentgenology, 215(6), 1321–1328. https://doi.org/10.2214/AJR.19.22680
Henschke, C. I., McCauley, D. I., Yankelevitz, D. F., Naidich, D. P., McGuinness, G., Miettinen, O. S., Libby, D. M., Pasmantier, M. W., Koizumi, J., Altorki, N. K., & Smith, J. P. (1999). Early Lung Cancer Action Project: overall design and findings from baseline screening. The Lancet, 354(9173), 99–105. https://doi.org/10.1016/S0140-6736(99)06093-6
Henschke, C. I., Yankelevitz, D. F., Mirtcheva, R., McGuinness, G., McCauley, D., & Miettinen, O. S. (2002). CT Screening for Lung Cancer. American Journal of Roentgenology, 178(5), 1053–1057. https://doi.org/10.2214/ajr.178.5.1781053
Homayounieh, F., Digumarthy, S., Ebrahimian, S., Rueckel, J., Hoppe, B. F., Sabel, B. O., Conjeti, S., Ridder, K., Sistermanns, M., Wang, L., Preuhs, A., Ghesu, F., Mansoor, A., Moghbel, M., Botwin, A., Singh, R., Cartmell, S., Patti, J., Huemmer, C., … Kalra, M. (2021). An Artificial Intelligence-Based Chest X-ray Model on Human Nodule Detection Accuracy From a Multicenter Study. JAMA Network Open, 4(12), e2141096. https://doi.org/10.1001/ jamanetworkopen.2021.41096
Hussein, S., Cao, K., Song, Q., & Bagci, U. (2017). Risk Stratification of Lung Nodules Using 3D CNN-Based Multi-task Learning. Information Processing in Medical Imaging, 249–260. https://doi.org/10.1007/978-3-319-59050-9_20
Hwang, E. J., Goo, J. M., Kim, H. Y., Yi, J., Yoon, S. H., & Kim, Y. (2021). Implementation of the cloud-based computerized interpretation system in a nationwide lung cancer screening with low-dose CT: comparison with the conventional reading system. European Radiology, 31(1), 475–485. https://doi.org/10.1007/ s00330-020-07151-7
Jacobs, C., Schreuder, A., van Riel, S. J., Scholten, E. T., Wittenberg, R., Wille, M. M. W., de Hoop, B., Sprengers, R., Mets, O. M., Geurts, B., Prokop, M., Schaefer-Prokop, C., & van Ginneken, B. (2021). Assisted versus Manual Interpretation of Low- Dose CT Scans for Lung Cancer Screening: Impact on Lung-RADS Agreement. Radiology. Imaging Cancer, 3(5), e200160. https://doi. org/10.1148/rycan.2021200160
Jiang, B., Li, N., Shi, X., Zhang, S., Li, J., de Bock, G. H., Vliegenthart, R., & Xie, X. (2022). Deep Learning Reconstruction Shows Better Lung Nodule Detection for Ultra-Low-Dose Chest CT. Radiology, 303(1), 202–212. https://doi.org/10.1148/radiol.210551
Jones, C. M., Buchlak, Q. D., Oakden-Rayner, L., Milne, M., Seah, J., Esmaili, N., & Hachey, B. (2021). Chest radiographs and machine learning - Past, present and future. Journal of Medical Imaging and Radiation Oncology, 65(5), 538–544. https://doi. org/10.1111/1754-9485.13274
Kinsinger, L. S., Anderson, C., Kim, J., Larson, M., Chan, S. H., King, H. A., Rice, K. L., Slatore, C. G., Tanner, N. T., Pittman, K., Monte, R. J., McNeil, R. B., Grubber, J. M., Kelley, M. J., Provenzale, D., Datta, S. K., Sperber, N. S., Barnes, L. K., Abbott, D. H., … Jackson, G. L. (2017). Implementation of Lung Cancer Screening in the Veterans Health Administration. JAMA Internal Medicine, 177(3), 399–406. https://doi.org/10.1001/ jamainternmed.2016.9022
LCS Project. (n.d.). https://www.myesti.org/lungcancerscreeningcertificationproject/, accessed on 26.09.2024
Leader, J. K., Warfel, T. E., Fuhrman, C. R., Golla, S. K., Weissfeld, J. L., Avila, R. S., Turner, W. D., & Zheng, B. (2005). Pulmonary nodule detection with low-dose CT of the lung: agreement among radiologists. AJR. American Journal of Roentgenology, 185(4), 973–978. https://doi.org/10.2214/AJR.04.1225
Li, J., Chung, S., Wei, E. K., & Luft, H. S. (2018). New recommendation and coverage of low-dose computed tomography for lung cancer screening: uptake has increased but is still low. BMC Health Services Research, 18(1), 525. https://doi.org/10.1186/ s12913-018-3338-9
Li, L., Liu, Z., Huang, H., Lin, M., & Luo, D. (2019). Evaluating the performance of a deep learning-based computer-aided diagnosis (DL-CAD) system for detecting and characterizing lung nodules: Comparison with the performance of double reading by radiologists. Thoracic Cancer, 10(2), 183–192. https://doi. org/10.1111/1759-7714.12931
Li, X., Shen, L., Xie, X., Huang, S., Xie, Z., Hong, X., & Yu, J. (2020). Multi-resolution convolutional networks for chest X-ray radiograph based lung nodule detection. Artificial Intelligence in Medicine, 103, 101744. https://doi.org/10.1016/j. artmed.2019.101744
Lu, M. T., Raghu, V. K., Mayrhofer, T., Aerts, H. J. W. L., & Hoffmann, U. (2020). Deep Learning Using Chest Radiographs to Identify High-Risk Smokers for Lung Cancer Screening Computed Tomography: Development and Validation of a Prediction Model. Annals of Internal Medicine, 173(9), 704–713. https://doi. org/10.7326/M20-1868
Lung Rads. (n.d.). https://www.acr.org/Clinical-Resources/Reporting-and-Data-Systems/Lung-Rads, accessed on 26.09.2024
Malhotra, J., Malvezzi, M., Negri, E., La Vecchia, C., & Boffetta, P. (2016). Risk factors for lung cancer worldwide. The European Respiratory Journal: Official Journal of the European Society for Clinical Respiratory Physiology, 48(3), 889–902. https://doi. org/10.1183/13993003.00359-2016
Marcus, P. M., Bergstralh, E. J., Fagerstrom, R. M., Williams, D. E., Fontana, R., Taylor, W. F., & Prorok, P. C. (2000). Lung cancer mortality in the Mayo Lung Project: impact of extended followup. Journal of the National Cancer Institute, 92(16), 1308–1316. https://doi.org/10.1093/jnci/92.16.1308
Mendoza, J., & Pedrini, H. (2020). Detection and classification of lung nodules in chest X‐ray images using deep convolutional neural networks. Computational Intelligence. An International Journal, 36(2), 370–401. https://doi.org/10.1111/coin.12241
Mikhael, P. G., Wohlwend, J., Yala, A., Karstens, L., Xiang, J., Takigami, A. K., Bourgouin, P. P., Chan, P., Mrah, S., Amayri, W., Juan, Y.-H., Yang, C.-T., Wan, Y.-L., Lin, G., Sequist, L. V., Fintelmann, F. J., & Barzilay, R. (2023). Sybil: A Validated Deep Learning Model to Predict Future Lung Cancer Risk From a Single Low-Dose Chest Computed Tomography. Journal of Clinical Oncology: Official Journal of the American Society of Clinical Oncology, 41(12), 2191–2200. https://doi.org/10.1200/ JCO.22.01345
Morgan, L., Choi, H., Reid, M., Khawaja, A., & Mazzone, P. J. (2017). Frequency of Incidental Findings and Subsequent Evaluation in Low-Dose Computed Tomographic Scans for Lung Cancer Screening. Annals of the American Thoracic Society, 14(9), 1450–1456. https://doi.org/10.1513/AnnalsATS.201612-1023OC
Murchison, J. T., Ritchie, G., Senyszak, D., Nijwening, J. H., van Veenendaal, G., Wakkie, J., & van Beek, E. J. R. (2022). Validation of a deep learning computer aided system for CT based lung nodule detection, classification, and growth rate estimation in a routine clinical population. PloS One, 17(5), e0266799. https://doi. org/10.1371/journal.pone.0266799
Nam, J. G., Ahn, C., Choi, H., Hong, W., Park, J., Kim, J. H., & Goo, J. M. (2021). Image quality of ultralow-dose chest CT using deep learning techniques: potential superiority of vendor-agnostic postprocessing over vendor-specific techniques. European Radiology, 31(7), 5139–5147. https://doi.org/10.1007/s00330-020-07537-7
Nam, J. G., Park, S., Hwang, E. J., Lee, J. H., Jin, K.-N., Lim, K. Y., Vu, T. H., Sohn, J. H., Hwang, S., Goo, J. M., & Park, C. M. (2019). Development and Validation of Deep Learning-based Automatic Detection Algorithm for Malignant Pulmonary Nodules on Chest Radiographs. Radiology, 290(1), 218–228. https://doi.org/10.1148/ radiol.2018180237
National Institute for Health and Care Excellence (NICE). (n.d.). Evidence standards framework for digital health technologies. https://www.nice.org.uk/corporate/ecd7, accessed on 26.09.2024
National Lung Screening Trial Research Team, Aberle, D. R., Adams, A. M., Berg, C. D., Black, W. C., Clapp, J. D., Fagerstrom, R. M., Gareen, I. F., Gatsonis, C., Marcus, P. M., & Sicks, J. D. (2011). Reduced lung-cancer mortality with low-dose computed tomographic screening. The New England Journal of Medicine, 365(5), 395–409. https://doi.org/10.1056/NEJMoa1102873
Park, H., Ham, S.-Y., Kim, H.-Y., Kwag, H. J., Lee, S., Park, G., Kim, S., Park, M., Sung, J.-K., & Jung, K.-H. (2019). A deep learning-based CAD that can reduce false negative reports: A preliminary study in health screening center. RSNA 2019. RSNA 2019. https://archive.rsna.org/2019/19017034.html
Pehrson, L. M., Nielsen, M. B., & Ammitzbøl Lauridsen, C. (2019). Automatic Pulmonary Nodule Detection Applying Deep Learning or Machine Learning Algorithms to the LIDC-IDRI Database: A Systematic Review. Diagnostics (Basel, Switzerland), 9(1). https://doi.org/10.3390/diagnostics9010029
Qi, L.-L., Wang, J.-W., Yang, L., Huang, Y., Zhao, S.-J., Tang, W., Jin, Y.-J., Zhang, Z.-W., Zhou, Z., Yu, Y.-Z., Wang, Y.-Z., & Wu, N. (2021). Natural history of pathologically confirmed pulmonary subsolid nodules with deep learning-assisted nodule segmentation. European Radiology, 31(6), 3884–3897. https://doi. org/10.1007/s00330-020-07450-z
Qi, L.-L., Wu, B.-T., Tang, W., Zhou, L.-N., Huang, Y., Zhao, S.-J., Liu, L., Li, M., Zhang, L., Feng, S.-C., Hou, D.-H., Zhou, Z., Li, X.- L., Wang, Y.-Z., Wu, N., & Wang, J.-W. (2020). Long-term followup of persistent pulmonary pure ground-glass nodules with deep learning-assisted nodule segmentation. European Radiology, 30(2), 744–755. https://doi.org/10.1007/s00330-019-06344-z
Raghu, V. K., Walia, A. S., Zinzuwadia, A. N., Goiffon, R. J., Shepard, J.-A. O., Aerts, H. J. W. L., Lennes, I. T., & Lu, M. T. (2022). Validation of a Deep Learning-Based Model to Predict Lung Cancer Risk Using Chest Radiographs and Electronic Medical Record Data. JAMA Network Open, 5(12), e2248793. https://doi. org/10.1001/jamanetworkopen.2022.48793
Ravin, C. E., & Chotas, H. G. (1997). Chest radiography. Radiology, 204(3), 593–600. https://doi.org/10.1148/radiology.204.3.9280231
Revel, M.-P., Bissery, A., Bienvenu, M., Aycard, L., Lefort, C., & Frija, G. (2004). Are two-dimensional CT measurements of small noncalcified pulmonary nodules reliable? Radiology, 231(2), 453–458. https://doi.org/10.1148/radiol.2312030167
Röhrich, S., Heidinger, B. H., Prayer, F., Weber, M., Krenn, M., Zhang, R., Sufana, J., Scheithe, J., Kanbur, I., Korajac, A., Pötsch, N., Raudner, M., Al-Mukhtar, A., Fueger, B. J., Milos, R.-I., Scharitzer, M., Langs, G., & Prosch, H. (2023). Impact of a content-based image retrieval system on the interpretation of chest CTs of patients with diffuse parenchymal lung disease. European Radiology, 33(1), 360–367. https://doi.org/10.1007/ s00330-022-08973-3
Sands, J., Tammemägi, M. C., Couraud, S., Baldwin, D. R., Borondy-Kitts, A., Yankelevitz, D., Lewis, J., Grannis, F., Kauczor, H.-U., von Stackelberg, O., Sequist, L., Pastorino, U., & McKee, B. (2021). Lung Screening Benefits and Challenges: A Review of The Data and Outline for Implementation. Journal of Thoracic Oncology: Official Publication of the International Association for the Study of Lung Cancer, 16(1), 37–53. https://doi. org/10.1016/j.jtho.2020.10.127
Setio, A. A. A., Traverso, A., de Bel, T., Berens, M. S. N., van den Bogaard, C., Cerello, P., Chen, H., Dou, Q., Fantacci, M. E., Geurts, B., Gugten, R. van der, Heng, P. A., Jansen, B., de Kaste, M. M. J., Kotov, V., Lin, J. Y.-H., Manders, J. T. M. C., Sóñora-Mengana, A., García-Naranjo, J. C., … Jacobs, C. (2017). Validation, comparison, and combination of algorithms for automatic detection of pulmonary nodules in computed tomography images: The LUNA16 challenge. Medical Image Analysis, 42, 1–13. https://doi.org/10.1016/j.media.2017.06.015
Siegel, D. A., Fedewa, S. A., Henley, S. J., Pollack, L. A., & Jemal, A. (2021). Proportion of Never Smokers Among Men and Women With Lung Cancer in 7 US States. JAMA Oncology, 7(2), 302–304. https://doi.org/10.1001/jamaoncol.2020.6362
Singh, R., Kalra, M. K., Homayounieh, F., Nitiwarangkul, C., McDermott, S., Little, B. P., Lennes, I. T., Shepard, J.-A. O., & Digumarthy, S. R. (2021). Artificial intelligence-based vessel suppression for detection of sub-solid nodules in lung cancer screening computed tomography. Quantitative Imaging in Medicine and Surgery, 11(4), 1134–1143. https://doi.org/10.21037/ qims-20-630
Smieliauskas, F., MacMahon, H., Salgia, R., & Shih, Y.- C. T. (2014). Geographic variation in radiologist capacity and widespread implementation of lung cancer CT screening. Journal of Medical Screening, 21(4), 207–215. https://doi. org/10.1177/0969141314548055
Sung, H., Ferlay, J., Siegel, R. L., Laversanne, M., Soerjomataram, I., Jemal, A., & Bray, F. (2021). Global Cancer Statistics 2020: GLOBOCAN Estimates of Incidence and Mortality Worldwide for 36 Cancers in 185 Countries. CA: A Cancer Journal for Clinicians, 71(3), 209–249. https://doi.org/10.3322/caac.21660
Sun, S., Schiller, J. H., & Gazdar, A. F. (2007). Lung cancer in never smokers--a different disease. Nature Reviews. Cancer, 7(10), 778–790. https://doi.org/10.1038/nrc2190
Tammemägi, M. C., Katki, H. A., Hocking, W. G., Church, T. R., Caporaso, N., Kvale, P. A., Chaturvedi, A. K., Silvestri, G. A., Riley, T. L., Commins, J., & Berg, C. D. (2013). Selection criteria for lung-cancer screening. The New England Journal of Medicine, 368(8), 728–736. https://doi.org/10.1056/NEJMoa1211776
Thai, A. A., Solomon, B. J., Sequist, L. V., Gainor, J. F., & Heist, R. S. (2021). Lung cancer. The Lancet, 398(10299), 535–554. https:// doi.org/10.1016/S0140-6736(21)00312-3
The Royal College of Radiologists. (2022). Clinical Radiology Workforce Census.
Toumazis, I., de Nijs, K., Cao, P., Bastani, M., Munshi, V., Ten Haaf, K., Jeon, J., Gazelle, G. S., Feuer, E. J., de Koning, H. J., Meza, R., Kong, C. Y., Han, S. S., & Plevritis, S. K. (2021). Costeffectiveness Evaluation of the 2021 US Preventive Services Task Force Recommendation for Lung Cancer Screening. JAMA Oncology, 7(12), 1833–1842. https://doi.org/10.1001/jamaoncol.2021.4942
van Riel, S. J., Sánchez, C. I., Bankier, A. A., Naidich, D. P., Verschakelen, J., Scholten, E. T., de Jong, P. A., Jacobs, C., van Rikxoort, E., Peters-Bax, L., Snoeren, M., Prokop, M., van Ginneken, B., & Schaefer-Prokop, C. (2015). Observer Variability for Classification of Pulmonary Nodules on Low-Dose CT Images and Its Effect on Nodule Management. Radiology, 277(3), 863–871. https://doi.org/10.1148/radiol.2015142700
Wang, C., Li, J., Zhang, Q., Wu, J., Xiao, Y., Song, L., Gong, H., & Li, Y. (2021). The landscape of immune checkpoint inhibitor therapy in advanced lung cancer. BMC Cancer, 21(1), 968. https:// doi.org/10.1186/s12885-021-08662-2
Wang, Y., Midthun, D. E., Wampfler, J. A., Deng, B., Stoddard, S. M., Zhang, S., & Yang, P. (2015). Trends in the proportion of patients with lung cancer meeting screening criteria. JAMA: The Journal of the American Medical Association, 313(8), 853–855. https://doi.org/10.1001/jama.2015.413
Wood, D. E., Kazerooni, E. A., Baum, S. L., Eapen, G. A., Ettinger, D. S., Hou, L., Jackman, D. M., Klippenstein, D., Kumar, R., Lackner, R. P., Leard, L. E., Lennes, I. T., Leung, A. N. C., Makani, S. S., Massion, P. P., Mazzone, P., Merritt, R. E., Meyers, B. F., Midthun, D. E., … Hughes, M. (2018). Lung Cancer Screening, Version 3.2018, NCCN Clinical Practice Guidelines in Oncology. Journal of the National Comprehensive Cancer Network: JNCCN, 16(4), 412–441. https://doi.org/10.6004/jnccn.2018.0020
Wu, B., Zhou, Z., Wang, J., & Wang, Y. (2018). Joint learning for pulmonary nodule segmentation, attributes and malignancy prediction. 2018 IEEE 15th International Symposium on Biomedical Imaging (ISBI 2018), 1109–1113. https://doi.org/10.1109/ ISBI.2018.8363765
Wyker, A., & Henderson, W. W. (2022). Solitary Pulmonary Nodule. StatPearls Publishing.
Yoo, H., Lee, S. H., Arru, C. D., Doda Khera, R., Singh, R., Siebert, S., Kim, D., Lee, Y., Park, J. H., Eom, H. J., Digumarthy, S. R., & Kalra, M. K. (2021). AI-based improvement in lung cancer detection on chest radiographs: results of a multi-reader study in NLST dataset. European Radiology, 31(12), 9664–9674. https://doi. org/10.1007/s00330-021-08074-7
Zahnd, W. E., & Eberth, J. M. (2019). Lung Cancer Screening Utilization: A Behavioral Risk Factor Surveillance System Analysis. American Journal of Preventive Medicine, 57(2), 250–255. https:// doi.org/10.1016/j.amepre.2019.03.015
PP-CALA-DE-0240