Lung Cancer
Lung cancer is the leading cause of cancer-related deaths worldwide and the second most common cancer after breast cancer (Sung et al., 2021). Smoking remains the primary risk factor for the disease, with about three-quarters of patients being smokers or ex-smokers (Maholtra et al., 2016). Other risk factors include exposure to secondhand smoke, environmental pollutants, radon gas, and occupational hazards such as asbestos (Malhotra et al., 2016).
Estimated Cancer Deaths US 2022
Females
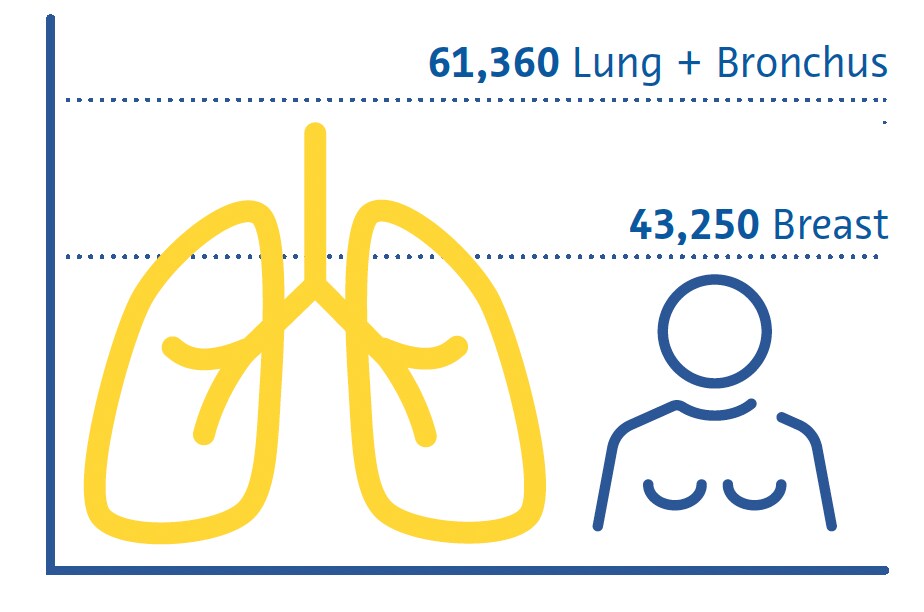
There are two main subtypes of lung cancer: non-small cell lung cancer (NSCLC) and small cell lung cancer (SCLC) (Thai et al., 2021). NSCLC is the most prevalent, representing about 85 % of all cases, and includes adenocarcinoma, squamous cell carcinoma, and large cell carcinoma (Thai et al., 2021). SCLC is the more aggressive form, often associated with rapid tumor growth and early metastasis (Thai et al., 2021).
Clinical features of lung cancer are often nonspecific in the early stages, contributing to delays in its diagnosis (Hamilton et al., 2005). Common symptoms include persistent cough, chest pain, shortness of breath, and coughing up blood (Hamilton et al., 2005). Lung cancer diagnosis involves imaging studies and open “bronchoscopic” or radiological-guided tissue biopsy essential to confirm the diagnosis and the histological subtype and guide treatment decisions (Detterbeck et al., 2013).
The treatment of lung cancer is dependent on the stage at which it is diagnosed and the histological subtypes (Detterbeck et al., 2013). Surgery, radiation therapy, and chemotherapy are the primary therapeutic modalities (Detterbeck et al., 2013; Thai et al., 2021). Early-stage NSCLC may be treated with surgical resection of the tumor, while advanced cases often require a combination of chemotherapy and radiation therapy (Detterbeck et al., 2013; Thai et al., 2021). Due to its aggressive nature, SCLC is often treated with chemotherapy, sometimes in conjunction with radiation therapy (Detterbeck et al., 2013; Thai et al., 2021).
Immunotherapy has emerged as a promising option for lung cancer treatment, particularly in NSCLC (Thai et al., 2021; C. Wang et al., 2021). Drugs that target immune checkpoints have shown efficacy in improving survival in certain patients (Thai et al., 2021; C. Wang et al., 2021).
Targeted therapies, focusing on specific genetic mutations or alterations, have also been developed for subsets of lung cancer patients, providing more personalized and effective treatment options (Thai et al., 2021). A multidisciplinary approach involving oncologists, surgeons, radiologists, and other healthcare professionals is essential to tailor treatment plans to patients’ individual needs (Detterbeck et al., 2013).
Screening strategies
The earliest lung cancer screening clinical trials used sputum analysis and conventional chest radiography and found no association between screening and lower mortality (Marcus et al., 2000). With its superior ability to detect non-calcified nodules that potentially represent early-stage cancer (Henschke et al., 1999), subsequent trials found that screening using low-dose computed tomography (CT) reduces lung cancer-related mortality by 20 % (National Lung Screening TriaResearch Team et. al., 2011; Aberle et. al.; 2011) and 24 % (de Koning et. al., 2020). Despite low-dose CT being more expensive and involving a higher radiation dose than conventional radiography, the risks associated with radiation exposure are very low (Sands et al., 2021) and large studies have found such screening strategies to be cost-effective (Black et al., 2014; Toumazis et al., 2021).
Lung cancer screening is most effective when targeted at individuals at high risk of developing the disease. Current national screening guidelines in many countries largely identify these individuals using criteria derived from the early clinical trials on lung cancer screening, including age and smoking history in pack-years. Researchers in other countries have developed mathematical models to estimate the risk of lung cancer to determine screening eligibility by incorporating additional variables such as race, personal and family history of lung cancer, and body mass index (Cassidy et al., 2008; Field et al., 2016; Tammemägi et al., 2013).
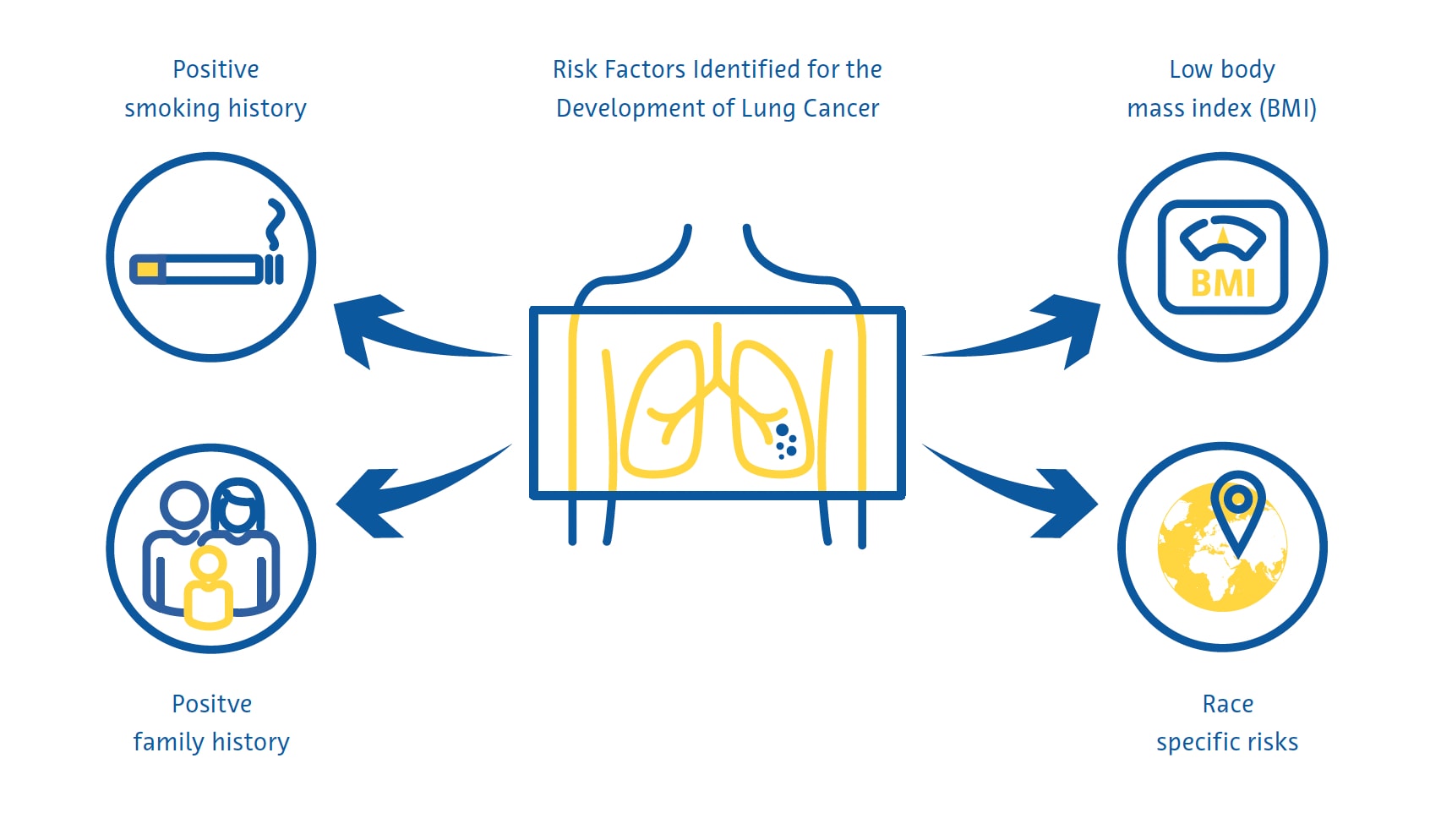
Screening challenges
Despite the strong evidence for the effectiveness of lung cancer screening, its practical implementation suffers from several challenges. Engagement in, and adherence to, lung cancer screening programs is low, with an uptake rate of just 6-14% in the United States (J. Li et al., 2018; Zahnd & Eberth, 2019) and as well as low rates in the UK, particularly amongst those at highest risk of lung cancer (Ali et al., 2015). As more countries roll out national lung cancer screening programs, the already severe worldwide shortage of radiologists (AAMC Report Reinforces Mounting Physician Shortage, 2021; Smieliauskas et al., 2014; The Royal College of Radiologists, 2022) may further complicate lung cancer screening as there will be less qualified radiologists to read an increased number of CT studies.
This is especially problematic as reporting lung cancer screening examinations requires specific expertise and training (LCS Project, n.d.). In addition, higher reporting loads are generally associated with more errors by radiologists (Hanna et al., 2018), which may lead to worse screening outcomes. There is also no consensus on how to deal with incidental findings that are identified during lung cancer screening. These findings result in a further diagnostic workup in up to 15 % of screened patients (Morgan et al., 2017) and are associated with increased patient anxiety and costs for the healthcare system (Adams et al., 2016).
Role of artificial intelligence
Identifying high-risk individuals
The eligibility criteria for lung cancer screening in the United States, adopted by the Centers for Medicare and Medicaid Services (CMS), miss over half of lung cancer cases (Y. Wang et al., 2015). These criteria, which only include smoking history and age, provide suboptimal risk prediction because they ignore other important risk factors (Burzic et al., 2022) and are often based on inaccurate or unavailable data (Kinsinger et al., 2017). This is especially important considering that about a quarter of all lung cancer cases are not attributed to smoking (Sun et al., 2007).
For this reason, studies have investigated the use of artificial intelligence (AI) to improve lung cancer risk prediction and include more people in screening programs. Combining information from electronic medical records and conventional chest radiographs using a convolutional neural network (CNN) was found to predict lung cancer over a 12-year period better than existing screening criteria and was associated with 31 % fewer lung cancer cases being missed (Lu et al., 2020). Another study using a similar approach found that patients classified as being at high risk for lung cancer using both conventional chest radiographs and the CMS criteria but not using the CMS criteria alone had a 6-year incidence of lung cancer of 3.3 % (Raghu et al., 2022), far higher than the 1.3 % 6-year risk threshold for lung cancer screening, which is similar to the threshold used by the USPSTF (United States Preventative Services Task Force) (Wood et al., 2018).
Radiation dose reduction & image quality improvement
Deep learning techniques can be utilized for image denoising, with some solutions for chest CT being commercially available (Nam et al., 2021). Deep-learning-based image reconstruction of ultra-low-dose CT increased nodule detection rate and improved nodule measurement accuracy compared to conventional reconstruction algorithms (Jiang et al., 2022). Deep-learning-based denoising of ultra-low-dose CT also showed better subjective image quality than ultra-low-dose CT without denoising (Hata et al., 2020).
Lung nodule detection
Pulmonary nodules are defined as a small (typically less than 3 centimeters), rounded or irregular mass in the lung tissue that can be infectious, inflammatory, congenital, or neoplastic (Wyker & Henderson, 2022). Lung nodule detection by radiologists is time-consuming and prone to errors such as non-identification or misidentification of potentially malignant nodules (Al Mohammad et al., 2019; Armato et al., 2009; Gierada et al., 2017; Leader et al., 2005).
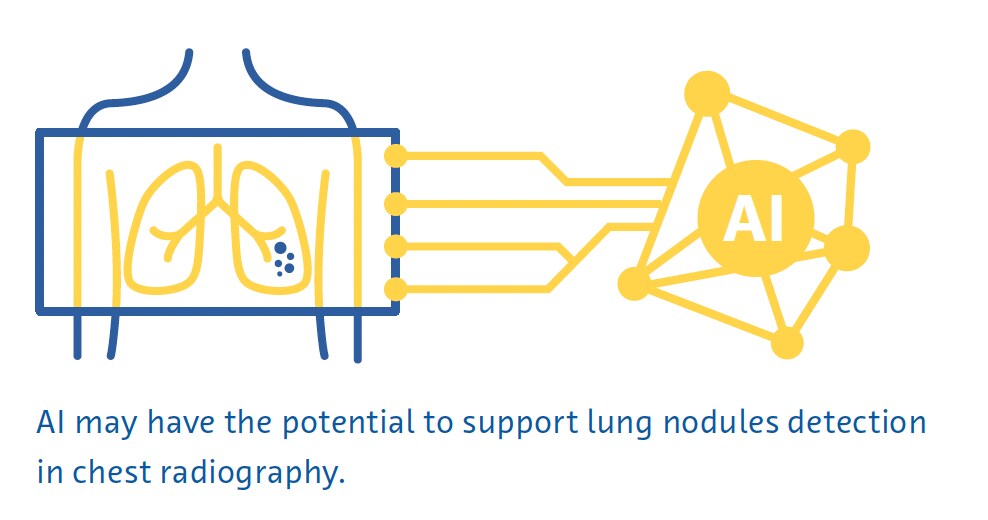
Small lung nodules are often invisible on conventional chest radiographs, and even large nodules can be missed by radiologists on radiographs (Austin et al., 1992). Despite this, the potential for AI to detect lung nodules and cancers on chest radiography is an area of active research (Cha et al., 2019; Homayounieh et al., 2021; Jones et al., 2021; X. Li et al., 2020; Mendoza & Pedrini, 2020; Nam et al., 2019; Yoo et al., 2021) because of its role as a first-line imaging examination in respiratory and cardiac diseases and its widespread availability and low cost (Ravin & Chotas, 1997).
A meta-analysis of nine studies found an AUC of 0.884 for the detection of lung nodules on conventional chest radiographs using AI (Aggarwal et al., 2021).
A meta-analysis of 41 studies found a 55–99 % sensitivity for traditional machine learning and 80–97 % for deep learning algorithms for identifying lung nodules on low-dose CT (Pehrson et al., 2019). One of the first studies to use deep learning to detect lung nodules on low-dose CT found a sensitivity of 98.3 % and one false positive per examination using a combination of different algorithms (Setio et al., 2017). False positives tend to be blood vessels, scar tissue, or sections of the thoracic wall, vertebrae or mediastinal tissue (Cui et al., 2022; L. Li et al., 2019; Setio et al., 2017).
A meta-analysis that included 56 studies found an AUC of 0.94 for lung nodule detection on CT using deep learning (Aggarwal et al., 2021). However, very few of these studies used prospectively collected data or validated the algorithms on an independent external dataset (Aggarwal et al., 2021). A deep learning algorithm trained on over 10,000 low-dose chest CTs achieved an AUC of 0.86–0.94 for lung cancer prediction at 1 year using histopathology as a reference standard when tested on three external validation datasets (Mikhael et al., 2023).
A study of 346 individuals who participated in a lung cancer screening program found that a deep learning algorithm had higher sensitivity for lung nodules than double-reading by two radiologists subspecialized in thoracic imaging (86 % vs 79 %) but a much higher false detection rate (1.53 vs 0.13 per scan) (L. Li et al., 2019). A similar study of 360 individuals found that a deep learning algorithm detected lung nodules on low-dose CT with a sensitivity of 90 % and a false detection rate of 1 per scan compared to a sensitivity of 76 % and a false detection rate of 0.04 per scan when the examinations were double-read by pairs consisting of one junior and one senior radiologist (Cui et al., 2022). The difference in sensitivity between the algorithm and the radiologists was particularly large for nodules with a diameter between 4 and 6 mm (86 % vs 59 %) (Cui et al., 2022).
Lung nodule segmentation
Precise measurement of lung nodules is important for monitoring nodule growth over time, as well as to guide management of these lesions (Bankier et al., 2017). However, the estimated error in the manual measurement of lung nodule diameter is about 1.5 mm, which represents a significant misestimation of size for small nodules (Bankier et al., 2017; Revel et al., 2004). Moreover, relying on nodule diameter may not accurately reflect nodule growth because it assumes that all nodules are perfect spheres (Devaraj et al., 2017). In this regard, the latest version of LungRADS 2022 included the possibility of performing a volumetric (mm3) assessment.
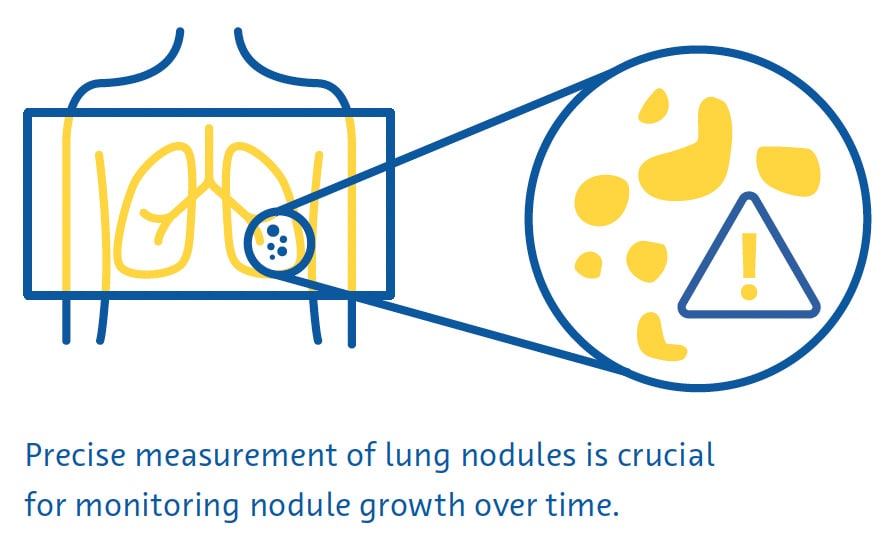
Segmentation of lung nodules allows accurate estimation of their volume and is a multi-step process that includes nodule detection, a “region-growing” process by which the boundaries of the nodules are identified by exploiting differences in tissue attenuation between the nodule and the surrounding lung parenchyma, and removal of surrounding structures with similar attenuation such as blood vessels (Devaraj et al., 2017).
Simple segmentation approaches that rely heavily on differences in attenuation between nodules and the surrounding lung parenchyma perform poorly with juxtavascular and subsolid nodules (Devaraj et al., 2017). CNNs, particularly encoder-decoder algorithms, on the other hand, achieve much better segmentation performance with Dice similarity coefficients (a measure of spatial overlap) of 0.79 to 0.93 compared to ground truth segmentations by radiologists (Dong et al., 2020; Gu et al., 2021). Several AI-based lung nodule segmentation algorithms that perform automated nodule volumetry and longitudinal volume tracking are commercially available (Hwang et al., 2021; Jacobs et al., 2021; Murchison et al., 2022; Park et al., 2019; Röhrich et al., 2023; Singh et al., 2021).
Lung nodule classification
Factors that are taken into account when determining the likelihood of a lung nodule being cancerous include its size, shape, composition, location, and if and how it changes over time (Callister et al., 2015; Lung Rads, n.d.). Studies have shown moderate inter- and intraobserver agreement between radiologists for characteristics used to estimate the probability of a lung nodule being malignant with discordant classification in over a third of nodules (van Riel et al., 2015). Several commercially available AI-based solutions for lung nodule classification, many of which include an assessment of malignancy risk, are currently available (Adams et al., 2023; Hwang et al., 2021; Murchison et al., 2022; Park et al., 2019; Röhrich et al., 2023).
A deep learning algorithm trained on data from 943 patients and validated on an independent dataset of 468 patients showed an overall accuracy of 78–80 % for classifying nodules into one of 6 categories (solid, calcified, part-solid, non-solid, perifissural, or spiculated) (Ciompi et al., 2017). The accuracy was lowest for part-solid, spiculated, and perifissural nodules (Ciompi et al., 2017).
A deep learning algorithm tested on 6716 low-dose CTs and validated on an independent dataset of 1139 low-dose CTs showed an AUC of 0.94 for predicting the risk of lung cancer using histopathology as a reference standard (Ardila et al., 2019). When available, the algorithm incorporates information from prior CTs of the same patient, and in these cases, its performance was similar to that of six radiologists (Ardila et al., 2019). When prior imaging was unavailable, the algorithm had an 11 % lower false positive rate and a 5 % lower false negative rate than the radiologists (Ardila et al., 2019).
Another study used a multitask 3-dimensional CNN to extract nodule characteristics such as calcification, lobulation, sphericity, spiculation, margins, and texture with an “off-by-one” accuracy of 91.3 % (Hussein et al., 2017).
The study used transfer learning of an algorithm trained on a million videos and tested it on a dataset of over 1000 chest CT scans. The reference standard consisted of malignancy scores and nodule characteristic scores assessed by at least three radiologists (Hussein et al., 2017). Using a multi-task interpretable 3D CNN tested on the same dataset without transfer learning, another study simultaneously segmented lung nodules, predicted the likelihood of malignancy, and outputted descriptive nodule attributes, achieving a Dice similarity coefficient of 0.74 and an “off-by-one” accuracy of 97.6 % (Wu et al., 2018).
Challenges and Future Directions
Based on all the available evidence, some countries have begun to incorporate the use of AI into their national screening programs. In this vein, it is worth mentioning the recent publication of a federal law by the German Federal Ministry of Justice, which includes the use of software for computer-assisted detection of lung nodules in the new lung cancer screening program, for the detection and volumetry of pulmonary nodules, determining the volume doubling time, and the storage of the evaluation for structured reporting Federal Ministry for the Environment, Nature Conservation, Nuclear Safety and Consumer Protection. (2024). https://www.recht.bund.de/bgbl/1/2024/162/VO.html accessed on 26.09.2024
Despite the very encouraging results achieved in recent years in the improvement of lung cancer screening using artificial intelligence, several important methodological challenges remain.
The sheer heterogeneity of the studies conducted so far makes evidence synthesis through meta-analysis difficult (Aggarwal et al., 2021). Moreover, it is unclear how generalizable these algorithms are as the majority of studies lack robust external validation (Aggarwal et al., 2021). There is also a need to investigate the additional value of some of these algorithms beyond improvements in diagnostic performance, such as in terms of increased efficiency or reduced costs (National Institute for Health and Care Excellence (NICE), n.d.).
Subsolid nodules, including pure ground glass nodules, are more likely to be malignant than solid nodules (Henschke et al., 2002). However, because attenuation differences between these nodules and the surrounding lung parenchyma are very subtle, they are particularly challenging to detect (de Margerie-Mellon & Chassagnon, 2023; L. Li et al., 2019; Setio et al., 2017). Some automated algorithms have shown promise for detecting subsolid nodules but have yet to be extensively validated (Qi et al., 2020, 2021).
Conclusion
The use of artificial intelligence may help identify more individuals at high risk of lung cancer and improve the quality of screening examinations. AI has proven particularly useful in identifying and segmenting potentially malignant lung nodules, often exceeding the sensitivity of radiologists (Cui et al., 2022; L. Li et al., 2019). It has also shown promise in estimating the likelihood of lung nodule malignancy, particularly when prior imaging is available.
Future research should aim at addressing the drawbacks of past studies, including the lack of robust external validation and neglect of efficiency- and cost-related outcomes, paving the way for earlier diagnosis and better treatment of lung cancer.
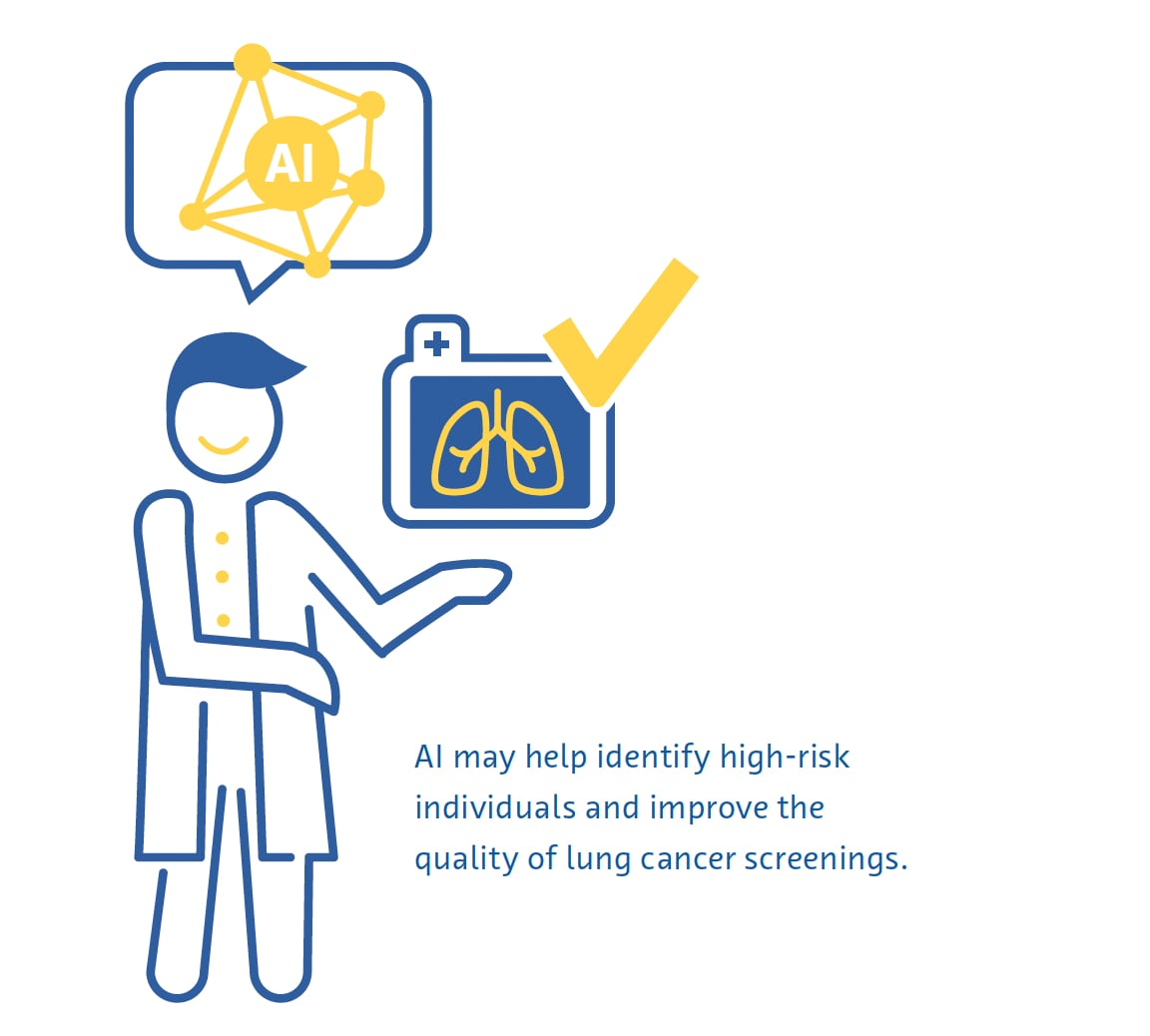
References
AAMC Report Reinforces Mounting Physician Shortage. (2021). AAMC. https://www.aamc.org/news-insights/press-releases/aamcreport- reinforces-mounting-physician-shortage, accessed on 26.09.2024
Adams, S. J., Babyn, P. S., & Danilkewich, A. (2016). Toward a comprehensive management strategy for incidental findings in imaging. Canadian Family Physician Medecin de Famille Canadien, 62(7), 541–543.
Adams, S. J., Madtes, D. K., Burbridge, B., Johnston, J., Goldberg, I. G., Siegel, E. L., Babyn, P., Nair, V. S., & Calhoun, M. E. (2023). Clinical Impact and Generalizability of a Computer-Assisted Diagnostic Tool to Risk-Stratify Lung Nodules With CT. Journal of the American College of Radiology: JACR, 20(2), 232–242. https:// doi.org/10.1016/j.jacr.2022.08.006
Aggarwal, R., Farag, S., Martin, G., Ashrafian, H., & Darzi, A. (2021). Patient Perceptions on Data Sharing and Applying Artificial Intelligence to Health Care Data: Cross-sectional Survey. Journal of Medical Internet Research, 23(8), e26162. https://doi.org/10.2196/26162
Aggarwal, R., Sounderajah, V., Martin, G., Ting, D. S. W., Karthikesalingam, A., King, D., Ashrafian, H., & Darzi, A. (2021). Diagnostic accuracy of deep learning in medical imaging: a systematic review and meta-analysis. NPJ Digital Medicine, 4(1), 65. https://doi.org/10.1038/s41746-021-00438-z
Ali, N., Lifford, K. J., Carter, B., McRonald, F., Yadegarfar, G., Baldwin, D. R., Weller, D., Hansell, D. M., Duffy, S. W., Field, J. K., & Brain, K. (2015). Barriers to uptake among high-risk individuals declining participation in lung cancer screening: a mixed methods analysis of the UK Lung Cancer Screening (UKLS) trial. BMJ Open, 5(7), e008254. https://doi.org/10.1136/ bmjopen-2015-008254
Al Mohammad, B., Hillis, S. L., Reed, W., Alakhras, M., & Brennan, P. C. (2019). Radiologist performance in the detection of lung cancer using CT. Clinical Radiology, 74(1), 67–75. https://doi.org/10.1016/j.crad.2018.10.008
Ardila, D., Kiraly, A. P., Bharadwaj, S., Choi, B., Reicher, J. J., Peng, L., Tse, D., Etemadi, M., Ye, W., Corrado, G., Naidich, D. P., & Shetty, S. (2019). End-to-end lung cancer screening with three-dimensional deep learning on low-dose chest computed tomography. Nature Medicine, 25(6), 954–961. https://doi.org/10.1038/s41591-019-0447-x
Armato, S. G., 3rd, Roberts, R. Y., Kocherginsky, M., Aberle, D. R., Kazerooni, E. A., Macmahon, H., van Beek, E. J. R., Yankelevitz, D., McLennan, G., McNitt-Gray, M. F., Meyer, C. R., Reeves, A. P., Caligiuri, P., Quint, L. E., Sundaram, B., Croft, B. Y., & Clarke, L. P. (2009). Assessment of radiologist performance in the detection of lung nodules: dependence on the definition of “truth.” Academic Radiology, 16(1), 28–38. https://doi.org/10.1016/j.acra.2008.05.022
Austin, J. H., Romney, B. M., & Goldsmith, L. S. (1992). Missed bronchogenic carcinoma: radiographic findings in 27 patients with a potentially resectable lesion evident in retrospect. Radiology, 182(1), 115–122. https://doi.org/10.1148/radiology.182.1.1727272
Bankier, A. A., MacMahon, H., Goo, J. M., Rubin, G. D., Schaefer- Prokop, C. M., & Naidich, D. P. (2017). Recommendations for Measuring Pulmonary Nodules at CT: A Statement from the Fleischner Society. Radiology, 285(2), 584–600. https://doi. org/10.1148/radiol.2017162894
Black, W. C., Gareen, I. F., Soneji, S. S., Sicks, J. D., Keeler, E. B., Aberle, D. R., Naeim, A., Church, T. R., Silvestri, G. A., Gorelick, J., Gatsonis, C., & National Lung Screening Trial Research Team. (2014). Cost-effectiveness of CT screening in the National Lung Screening Trial. The New England Journal of Medicine, 371(19), 1793–1802. https://doi.org/10.1056/NEJMoa1312547
Burzic, A., O’Dowd, E. L., & Baldwin, D. R. (2022). The Future of Lung Cancer Screening: Current Challenges and Research Priorities. Cancer Management and Research, 14, 637–645. https://doi.org/10.2147/CMAR.S293877
Callister, M. E. J., Baldwin, D. R., Akram, A. R., Barnard, S., Cane, P., Draffan, J., Franks, K., Gleeson, F., Graham, R., Malhotra, P., Prokop, M., Rodger, K., Subesinghe, M., Waller, D., Woolhouse, I., British Thoracic Society Pulmonary Nodule Guideline Development Group, & British Thoracic Society Standards of Care Committee. (2015). British Thoracic Society guidelines for the investigation and management of pulmonary nodules. Thorax, 70 Suppl 2, ii1–ii54. https://doi.org/10.1136/thoraxjnl-2015-207168
Cassidy, A., Myles, J. P., van Tongeren, M., Page, R. D., Liloglou, T., Duffy, S. W., & Field, J. K. (2008). The LLP risk model: an individual risk prediction model for lung cancer. British Journal of Cancer, 98(2), 270–276. https://doi.org/10.1038/sj.bjc.6604158
Cha, M. J., Chung, M. J., Lee, J. H., & Lee, K. S. (2019). Performance of Deep Learning Model in Detecting Operable Lung Cancer With Chest Radiographs. Journal of Thoracic Imaging, 34(2), 86–91. https://doi.org/10.1097/RTI.0000000000000388
Ciompi, F., Chung, K., van Riel, S. J., Setio, A. A. A., Gerke, P. K., Jacobs, C., Scholten, E. T., Schaefer-Prokop, C., Wille, M. M. W., Marchianò, A., Pastorino, U., Prokop, M., & van Ginneken, B. (2017). Towards automatic pulmonary nodule management in lung cancer screening with deep learning. Scientific Reports, 7, 46479. https://doi.org/10.1038/srep46479
Cui, X., Zheng, S., Heuvelmans, M. A., Du, Y., Sidorenkov, G., Fan, S., Li, Y., Xie, Y., Zhu, Z., Dorrius, M. D., Zhao, Y., Veldhuis, R. N. J., de Bock, G. H., Oudkerk, M., van Ooijen, P. M. A., Vliegenthart, R., & Ye, Z. (2022). Performance of a deep learning-based lung nodule detection system as an alternative reader in a Chinese lung cancer screening program. European Journal of Radiology, 146, 110068. https://doi.org/10.1016/j. ejrad.2021.110068
de Koning, H. J., van der Aalst, C. M., de Jong, P. A., Scholten, E. T., Nackaerts, K., Heuvelmans, M. A., Lammers, J.-W. J., Weenink, C., Yousaf-Khan, U., Horeweg, N., van ’t Westeinde, S., Prokop, M., Mali, W. P., Mohamed Hoesein, F. A. A., van Ooijen, P. M. A., Aerts, J. G. J. V., den Bakker, M. A., Thunnissen, E., Verschakelen, J., … Oudkerk, M. (2020). Reduced Lung-Cancer Mortality with Volume CT Screening in a Randomized Trial. The New England Journal of Medicine, 382(6), 503–513. https://doi. org/10.1056/NEJMoa1911793
de Margerie-Mellon, C., & Chassagnon, G. (2023). Artificial intelligence: A critical review of applications for lung nodule and lung cancer. Diagnostic and Interventional Imaging, 104(1), 11–17. https://doi.org/10.1016/j.diii.2022.11.007
Detterbeck, F. C., Lewis, S. Z., Diekemper, R., Addrizzo-Harris, D., & Alberts, W. M. (2013). Executive Summary: Diagnosis and management of lung cancer, 3rd ed: American College of Chest Physicians evidence-based clinical practice guidelines. Chest, 143(5 Suppl), 7S – 37S. https://doi.org/10.1378/chest.12-2377
Devaraj, A., van Ginneken, B., Nair, A., & Baldwin, D. (2017). Use of Volumetry for Lung Nodule Management: Theory and Practice. Radiology, 284(3), 630–644. https://doi.org/10.1148/ radiol.2017151022
Dong, X., Xu, S., Liu, Y., Wang, A., Saripan, M. I., Li, L., Zhang, X., & Lu, L. (2020). Multi-view secondary input collaborative deep learning for lung nodule 3D segmentation. Cancer Imaging: The Official Publication of the International Cancer Imaging Society, 20(1), 53. https://doi.org/10.1186/s40644-020-00331-0
Field, J. K., Duffy, S. W., Baldwin, D. R., Whynes, D. K., Devaraj, A., Brain, K. E., Eisen, T., Gosney, J., Green, B. A., Holemans, J. A., Kavanagh, T., Kerr, K. M., Ledson, M., Lifford, K. J., McRonald, F. E., Nair, A., Page, R. D., Parmar, M. K. B., Rassl, D. M., … Hansell, D. M. (2016). UK Lung Cancer RCT Pilot Screening Trial: baseline findings from the screening arm provide evidence for the potential implementation of lung cancer screening. Thorax, 71(2), 161–170. https://doi.org/10.1136/thoraxjnl-2015-207140
Gierada, D. S., Pinsky, P. F., Duan, F., Garg, K., Hart, E. M., Kazerooni, E. A., Nath, H., Watts, J. R., Jr, & Aberle, D. R. (2017). Interval lung cancer after a negative CT screening examination: CT findings and outcomes in National Lung Screening Trial participants. European Radiology, 27(8), 3249–3256. https://doi. org/10.1007/s00330-016-4705-8
Gu, D., Liu, G., & Xue, Z. (2021). On the performance of lung nodule detection, segmentation and classification. Computerized Medical Imaging and Graphics: The Official Journal of the Computerized Medical Imaging Society, 89, 101886. https://doi. org/10.1016/j.compmedimag.2021.101886
Hamilton, W., Peters, T. J., Round, A., & Sharp, D. (2005). What are the clinical features of lung cancer before the diagnosis is made? A population based case-control study. Thorax, 60(12), 1059–1065. https://doi.org/10.1136/thx.2005.045880
Hanna, T. N., Lamoureux, C., Krupinski, E. A., Weber, S., & Johnson, J.-O. (2018). Effect of Shift, Schedule, and Volume on Interpretive Accuracy: A Retrospective Analysis of 2.9 Million Radiologic Examinations. Radiology, 287(1), 205–212. https://doi. org/10.1148/radiol.2017170555
Hata, A., Yanagawa, M., Yoshida, Y., Miyata, T., Tsubamoto, M., Honda, O., & Tomiyama, N. (2020). Combination of Deep Learning-Based Denoising and Iterative Reconstruction for Ultra-Low-Dose CT of the Chest: Image Quality and Lung-RADS Evaluation. AJR. American Journal of Roentgenology, 215(6), 1321–1328. https://doi.org/10.2214/AJR.19.22680
Henschke, C. I., McCauley, D. I., Yankelevitz, D. F., Naidich, D. P., McGuinness, G., Miettinen, O. S., Libby, D. M., Pasmantier, M. W., Koizumi, J., Altorki, N. K., & Smith, J. P. (1999). Early Lung Cancer Action Project: overall design and findings from baseline screening. The Lancet, 354(9173), 99–105. https://doi.org/10.1016/S0140-6736(99)06093-6
Henschke, C. I., Yankelevitz, D. F., Mirtcheva, R., McGuinness, G., McCauley, D., & Miettinen, O. S. (2002). CT Screening for Lung Cancer. American Journal of Roentgenology, 178(5), 1053–1057. https://doi.org/10.2214/ajr.178.5.1781053
Homayounieh, F., Digumarthy, S., Ebrahimian, S., Rueckel, J., Hoppe, B. F., Sabel, B. O., Conjeti, S., Ridder, K., Sistermanns, M., Wang, L., Preuhs, A., Ghesu, F., Mansoor, A., Moghbel, M., Botwin, A., Singh, R., Cartmell, S., Patti, J., Huemmer, C., … Kalra, M. (2021). An Artificial Intelligence-Based Chest X-ray Model on Human Nodule Detection Accuracy From a Multicenter Study. JAMA Network Open, 4(12), e2141096. https://doi.org/10.1001/ jamanetworkopen.2021.41096
Hussein, S., Cao, K., Song, Q., & Bagci, U. (2017). Risk Stratification of Lung Nodules Using 3D CNN-Based Multi-task Learning. Information Processing in Medical Imaging, 249–260. https://doi.org/10.1007/978-3-319-59050-9_20
Hwang, E. J., Goo, J. M., Kim, H. Y., Yi, J., Yoon, S. H., & Kim, Y. (2021). Implementation of the cloud-based computerized interpretation system in a nationwide lung cancer screening with low-dose CT: comparison with the conventional reading system. European Radiology, 31(1), 475–485. https://doi.org/10.1007/ s00330-020-07151-7
Jacobs, C., Schreuder, A., van Riel, S. J., Scholten, E. T., Wittenberg, R., Wille, M. M. W., de Hoop, B., Sprengers, R., Mets, O. M., Geurts, B., Prokop, M., Schaefer-Prokop, C., & van Ginneken, B. (2021). Assisted versus Manual Interpretation of Low- Dose CT Scans for Lung Cancer Screening: Impact on Lung-RADS Agreement. Radiology. Imaging Cancer, 3(5), e200160. https://doi. org/10.1148/rycan.2021200160
Jiang, B., Li, N., Shi, X., Zhang, S., Li, J., de Bock, G. H., Vliegenthart, R., & Xie, X. (2022). Deep Learning Reconstruction Shows Better Lung Nodule Detection for Ultra-Low-Dose Chest CT. Radiology, 303(1), 202–212. https://doi.org/10.1148/radiol.210551
Jones, C. M., Buchlak, Q. D., Oakden-Rayner, L., Milne, M., Seah, J., Esmaili, N., & Hachey, B. (2021). Chest radiographs and machine learning - Past, present and future. Journal of Medical Imaging and Radiation Oncology, 65(5), 538–544. https://doi. org/10.1111/1754-9485.13274
Kinsinger, L. S., Anderson, C., Kim, J., Larson, M., Chan, S. H., King, H. A., Rice, K. L., Slatore, C. G., Tanner, N. T., Pittman, K., Monte, R. J., McNeil, R. B., Grubber, J. M., Kelley, M. J., Provenzale, D., Datta, S. K., Sperber, N. S., Barnes, L. K., Abbott, D. H., … Jackson, G. L. (2017). Implementation of Lung Cancer Screening in the Veterans Health Administration. JAMA Internal Medicine, 177(3), 399–406. https://doi.org/10.1001/ jamainternmed.2016.9022
LCS Project. (n.d.). https://www.myesti.org/lungcancerscreeningcertificationproject/, accessed on 26.09.2024
Leader, J. K., Warfel, T. E., Fuhrman, C. R., Golla, S. K., Weissfeld, J. L., Avila, R. S., Turner, W. D., & Zheng, B. (2005). Pulmonary nodule detection with low-dose CT of the lung: agreement among radiologists. AJR. American Journal of Roentgenology, 185(4), 973–978. https://doi.org/10.2214/AJR.04.1225
Li, J., Chung, S., Wei, E. K., & Luft, H. S. (2018). New recommendation and coverage of low-dose computed tomography for lung cancer screening: uptake has increased but is still low. BMC Health Services Research, 18(1), 525. https://doi.org/10.1186/ s12913-018-3338-9
Li, L., Liu, Z., Huang, H., Lin, M., & Luo, D. (2019). Evaluating the performance of a deep learning-based computer-aided diagnosis (DL-CAD) system for detecting and characterizing lung nodules: Comparison with the performance of double reading by radiologists. Thoracic Cancer, 10(2), 183–192. https://doi. org/10.1111/1759-7714.12931
Li, X., Shen, L., Xie, X., Huang, S., Xie, Z., Hong, X., & Yu, J. (2020). Multi-resolution convolutional networks for chest X-ray radiograph based lung nodule detection. Artificial Intelligence in Medicine, 103, 101744. https://doi.org/10.1016/j. artmed.2019.101744
Lu, M. T., Raghu, V. K., Mayrhofer, T., Aerts, H. J. W. L., & Hoffmann, U. (2020). Deep Learning Using Chest Radiographs to Identify High-Risk Smokers for Lung Cancer Screening Computed Tomography: Development and Validation of a Prediction Model. Annals of Internal Medicine, 173(9), 704–713. https://doi. org/10.7326/M20-1868
Lung Rads. (n.d.). https://www.acr.org/Clinical-Resources/Reporting-and-Data-Systems/Lung-Rads, accessed on 26.09.2024
Malhotra, J., Malvezzi, M., Negri, E., La Vecchia, C., & Boffetta, P. (2016). Risk factors for lung cancer worldwide. The European Respiratory Journal: Official Journal of the European Society for Clinical Respiratory Physiology, 48(3), 889–902. https://doi. org/10.1183/13993003.00359-2016
Marcus, P. M., Bergstralh, E. J., Fagerstrom, R. M., Williams, D. E., Fontana, R., Taylor, W. F., & Prorok, P. C. (2000). Lung cancer mortality in the Mayo Lung Project: impact of extended followup. Journal of the National Cancer Institute, 92(16), 1308–1316. https://doi.org/10.1093/jnci/92.16.1308
Mendoza, J., & Pedrini, H. (2020). Detection and classification of lung nodules in chest X‐ray images using deep convolutional neural networks. Computational Intelligence. An International Journal, 36(2), 370–401. https://doi.org/10.1111/coin.12241
Mikhael, P. G., Wohlwend, J., Yala, A., Karstens, L., Xiang, J., Takigami, A. K., Bourgouin, P. P., Chan, P., Mrah, S., Amayri, W., Juan, Y.-H., Yang, C.-T., Wan, Y.-L., Lin, G., Sequist, L. V., Fintelmann, F. J., & Barzilay, R. (2023). Sybil: A Validated Deep Learning Model to Predict Future Lung Cancer Risk From a Single Low-Dose Chest Computed Tomography. Journal of Clinical Oncology: Official Journal of the American Society of Clinical Oncology, 41(12), 2191–2200. https://doi.org/10.1200/ JCO.22.01345
Morgan, L., Choi, H., Reid, M., Khawaja, A., & Mazzone, P. J. (2017). Frequency of Incidental Findings and Subsequent Evaluation in Low-Dose Computed Tomographic Scans for Lung Cancer Screening. Annals of the American Thoracic Society, 14(9), 1450–1456. https://doi.org/10.1513/AnnalsATS.201612-1023OC
Murchison, J. T., Ritchie, G., Senyszak, D., Nijwening, J. H., van Veenendaal, G., Wakkie, J., & van Beek, E. J. R. (2022). Validation of a deep learning computer aided system for CT based lung nodule detection, classification, and growth rate estimation in a routine clinical population. PloS One, 17(5), e0266799. https://doi. org/10.1371/journal.pone.0266799
Nam, J. G., Ahn, C., Choi, H., Hong, W., Park, J., Kim, J. H., & Goo, J. M. (2021). Image quality of ultralow-dose chest CT using deep learning techniques: potential superiority of vendor-agnostic postprocessing over vendor-specific techniques. European Radiology, 31(7), 5139–5147. https://doi.org/10.1007/s00330-020-07537-7
Nam, J. G., Park, S., Hwang, E. J., Lee, J. H., Jin, K.-N., Lim, K. Y., Vu, T. H., Sohn, J. H., Hwang, S., Goo, J. M., & Park, C. M. (2019). Development and Validation of Deep Learning-based Automatic Detection Algorithm for Malignant Pulmonary Nodules on Chest Radiographs. Radiology, 290(1), 218–228. https://doi.org/10.1148/ radiol.2018180237
National Institute for Health and Care Excellence (NICE). (n.d.). Evidence standards framework for digital health technologies. https://www.nice.org.uk/corporate/ecd7, accessed on 26.09.2024
National Lung Screening Trial Research Team, Aberle, D. R., Adams, A. M., Berg, C. D., Black, W. C., Clapp, J. D., Fagerstrom, R. M., Gareen, I. F., Gatsonis, C., Marcus, P. M., & Sicks, J. D. (2011). Reduced lung-cancer mortality with low-dose computed tomographic screening. The New England Journal of Medicine, 365(5), 395–409. https://doi.org/10.1056/NEJMoa1102873
Park, H., Ham, S.-Y., Kim, H.-Y., Kwag, H. J., Lee, S., Park, G., Kim, S., Park, M., Sung, J.-K., & Jung, K.-H. (2019). A deep learning-based CAD that can reduce false negative reports: A preliminary study in health screening center. RSNA 2019. RSNA 2019. https://archive.rsna.org/2019/19017034.html
Pehrson, L. M., Nielsen, M. B., & Ammitzbøl Lauridsen, C. (2019). Automatic Pulmonary Nodule Detection Applying Deep Learning or Machine Learning Algorithms to the LIDC-IDRI Database: A Systematic Review. Diagnostics (Basel, Switzerland), 9(1). https://doi.org/10.3390/diagnostics9010029
Qi, L.-L., Wang, J.-W., Yang, L., Huang, Y., Zhao, S.-J., Tang, W., Jin, Y.-J., Zhang, Z.-W., Zhou, Z., Yu, Y.-Z., Wang, Y.-Z., & Wu, N. (2021). Natural history of pathologically confirmed pulmonary subsolid nodules with deep learning-assisted nodule segmentation. European Radiology, 31(6), 3884–3897. https://doi. org/10.1007/s00330-020-07450-z
Qi, L.-L., Wu, B.-T., Tang, W., Zhou, L.-N., Huang, Y., Zhao, S.-J., Liu, L., Li, M., Zhang, L., Feng, S.-C., Hou, D.-H., Zhou, Z., Li, X.- L., Wang, Y.-Z., Wu, N., & Wang, J.-W. (2020). Long-term followup of persistent pulmonary pure ground-glass nodules with deep learning-assisted nodule segmentation. European Radiology, 30(2), 744–755. https://doi.org/10.1007/s00330-019-06344-z
Raghu, V. K., Walia, A. S., Zinzuwadia, A. N., Goiffon, R. J., Shepard, J.-A. O., Aerts, H. J. W. L., Lennes, I. T., & Lu, M. T. (2022). Validation of a Deep Learning-Based Model to Predict Lung Cancer Risk Using Chest Radiographs and Electronic Medical Record Data. JAMA Network Open, 5(12), e2248793. https://doi. org/10.1001/jamanetworkopen.2022.48793
Ravin, C. E., & Chotas, H. G. (1997). Chest radiography. Radiology, 204(3), 593–600. https://doi.org/10.1148/radiology.204.3.9280231
Revel, M.-P., Bissery, A., Bienvenu, M., Aycard, L., Lefort, C., & Frija, G. (2004). Are two-dimensional CT measurements of small noncalcified pulmonary nodules reliable? Radiology, 231(2), 453–458. https://doi.org/10.1148/radiol.2312030167
Röhrich, S., Heidinger, B. H., Prayer, F., Weber, M., Krenn, M., Zhang, R., Sufana, J., Scheithe, J., Kanbur, I., Korajac, A., Pötsch, N., Raudner, M., Al-Mukhtar, A., Fueger, B. J., Milos, R.-I., Scharitzer, M., Langs, G., & Prosch, H. (2023). Impact of a content-based image retrieval system on the interpretation of chest CTs of patients with diffuse parenchymal lung disease. European Radiology, 33(1), 360–367. https://doi.org/10.1007/ s00330-022-08973-3
Sands, J., Tammemägi, M. C., Couraud, S., Baldwin, D. R., Borondy-Kitts, A., Yankelevitz, D., Lewis, J., Grannis, F., Kauczor, H.-U., von Stackelberg, O., Sequist, L., Pastorino, U., & McKee, B. (2021). Lung Screening Benefits and Challenges: A Review of The Data and Outline for Implementation. Journal of Thoracic Oncology: Official Publication of the International Association for the Study of Lung Cancer, 16(1), 37–53. https://doi. org/10.1016/j.jtho.2020.10.127
Setio, A. A. A., Traverso, A., de Bel, T., Berens, M. S. N., van den Bogaard, C., Cerello, P., Chen, H., Dou, Q., Fantacci, M. E., Geurts, B., Gugten, R. van der, Heng, P. A., Jansen, B., de Kaste, M. M. J., Kotov, V., Lin, J. Y.-H., Manders, J. T. M. C., Sóñora-Mengana, A., García-Naranjo, J. C., … Jacobs, C. (2017). Validation, comparison, and combination of algorithms for automatic detection of pulmonary nodules in computed tomography images: The LUNA16 challenge. Medical Image Analysis, 42, 1–13. https://doi.org/10.1016/j.media.2017.06.015
Siegel, D. A., Fedewa, S. A., Henley, S. J., Pollack, L. A., & Jemal, A. (2021). Proportion of Never Smokers Among Men and Women With Lung Cancer in 7 US States. JAMA Oncology, 7(2), 302–304. https://doi.org/10.1001/jamaoncol.2020.6362
Singh, R., Kalra, M. K., Homayounieh, F., Nitiwarangkul, C., McDermott, S., Little, B. P., Lennes, I. T., Shepard, J.-A. O., & Digumarthy, S. R. (2021). Artificial intelligence-based vessel suppression for detection of sub-solid nodules in lung cancer screening computed tomography. Quantitative Imaging in Medicine and Surgery, 11(4), 1134–1143. https://doi.org/10.21037/ qims-20-630
Smieliauskas, F., MacMahon, H., Salgia, R., & Shih, Y.- C. T. (2014). Geographic variation in radiologist capacity and widespread implementation of lung cancer CT screening. Journal of Medical Screening, 21(4), 207–215. https://doi. org/10.1177/0969141314548055
Sung, H., Ferlay, J., Siegel, R. L., Laversanne, M., Soerjomataram, I., Jemal, A., & Bray, F. (2021). Global Cancer Statistics 2020: GLOBOCAN Estimates of Incidence and Mortality Worldwide for 36 Cancers in 185 Countries. CA: A Cancer Journal for Clinicians, 71(3), 209–249. https://doi.org/10.3322/caac.21660
Sun, S., Schiller, J. H., & Gazdar, A. F. (2007). Lung cancer in never smokers--a different disease. Nature Reviews. Cancer, 7(10), 778–790. https://doi.org/10.1038/nrc2190
Tammemägi, M. C., Katki, H. A., Hocking, W. G., Church, T. R., Caporaso, N., Kvale, P. A., Chaturvedi, A. K., Silvestri, G. A., Riley, T. L., Commins, J., & Berg, C. D. (2013). Selection criteria for lung-cancer screening. The New England Journal of Medicine, 368(8), 728–736. https://doi.org/10.1056/NEJMoa1211776
Thai, A. A., Solomon, B. J., Sequist, L. V., Gainor, J. F., & Heist, R. S. (2021). Lung cancer. The Lancet, 398(10299), 535–554. https:// doi.org/10.1016/S0140-6736(21)00312-3
The Royal College of Radiologists. (2022). Clinical Radiology Workforce Census.
Toumazis, I., de Nijs, K., Cao, P., Bastani, M., Munshi, V., Ten Haaf, K., Jeon, J., Gazelle, G. S., Feuer, E. J., de Koning, H. J., Meza, R., Kong, C. Y., Han, S. S., & Plevritis, S. K. (2021). Costeffectiveness Evaluation of the 2021 US Preventive Services Task Force Recommendation for Lung Cancer Screening. JAMA Oncology, 7(12), 1833–1842. https://doi.org/10.1001/jamaoncol.2021.4942
van Riel, S. J., Sánchez, C. I., Bankier, A. A., Naidich, D. P., Verschakelen, J., Scholten, E. T., de Jong, P. A., Jacobs, C., van Rikxoort, E., Peters-Bax, L., Snoeren, M., Prokop, M., van Ginneken, B., & Schaefer-Prokop, C. (2015). Observer Variability for Classification of Pulmonary Nodules on Low-Dose CT Images and Its Effect on Nodule Management. Radiology, 277(3), 863–871. https://doi.org/10.1148/radiol.2015142700
Wang, C., Li, J., Zhang, Q., Wu, J., Xiao, Y., Song, L., Gong, H., & Li, Y. (2021). The landscape of immune checkpoint inhibitor therapy in advanced lung cancer. BMC Cancer, 21(1), 968. https:// doi.org/10.1186/s12885-021-08662-2
Wang, Y., Midthun, D. E., Wampfler, J. A., Deng, B., Stoddard, S. M., Zhang, S., & Yang, P. (2015). Trends in the proportion of patients with lung cancer meeting screening criteria. JAMA: The Journal of the American Medical Association, 313(8), 853–855. https://doi.org/10.1001/jama.2015.413
Wood, D. E., Kazerooni, E. A., Baum, S. L., Eapen, G. A., Ettinger, D. S., Hou, L., Jackman, D. M., Klippenstein, D., Kumar, R., Lackner, R. P., Leard, L. E., Lennes, I. T., Leung, A. N. C., Makani, S. S., Massion, P. P., Mazzone, P., Merritt, R. E., Meyers, B. F., Midthun, D. E., … Hughes, M. (2018). Lung Cancer Screening, Version 3.2018, NCCN Clinical Practice Guidelines in Oncology. Journal of the National Comprehensive Cancer Network: JNCCN, 16(4), 412–441. https://doi.org/10.6004/jnccn.2018.0020
Wu, B., Zhou, Z., Wang, J., & Wang, Y. (2018). Joint learning for pulmonary nodule segmentation, attributes and malignancy prediction. 2018 IEEE 15th International Symposium on Biomedical Imaging (ISBI 2018), 1109–1113. https://doi.org/10.1109/ ISBI.2018.8363765
Wyker, A., & Henderson, W. W. (2022). Solitary Pulmonary Nodule. StatPearls Publishing.
Yoo, H., Lee, S. H., Arru, C. D., Doda Khera, R., Singh, R., Siebert, S., Kim, D., Lee, Y., Park, J. H., Eom, H. J., Digumarthy, S. R., & Kalra, M. K. (2021). AI-based improvement in lung cancer detection on chest radiographs: results of a multi-reader study in NLST dataset. European Radiology, 31(12), 9664–9674. https://doi. org/10.1007/s00330-021-08074-7
Zahnd, W. E., & Eberth, J. M. (2019). Lung Cancer Screening Utilization: A Behavioral Risk Factor Surveillance System Analysis. American Journal of Preventive Medicine, 57(2), 250–255. https:// doi.org/10.1016/j.amepre.2019.03.015