Breast Cancer
Breast cancer is the most common specific type cancer among women globally (Sung et. al., 2021). Among women, breast cancer accounts for 1 in 4 cancer cases and for 1 in 6 cancer deaths, ranking first for incidence in the vast majority of countries (159 of 185 countries) and for mortality in 110 countries (Sung et. al., 2021). Most cases occur in women over the age of 50 years, but it can affect younger women as well. Other risk factors include a genetic predisposition, family history, early onset of menstruation, hormone replacement therapy, alcohol consumption, and obesity (Łukasiewicz et al., 2021).
The breast is composed of milk-producing lobules, a system of transport ducts, and fatty tissue (Bazira et al., 2021). All breast cancers originate in the cells lining the terminal duct lobular units (the functional unit of the breast) of the collecting ducts. The most common type for male breast cancer is invasive ductal carcinoma, which starts in the milk ducts and invades nearby tissues (Harbeck et al., 2019). Breast cancer development involves genetic mutations that cause uncontrolled cell proliferation as well as the BRCA1 and BRCA2 genes, which are involved in DNA repair (Harbeck et al., 2019). Estrogen and progesterone receptors play important roles in the pathophysiology, all patients with tumors that express these receptors should receive hormonal therapy to block estrogen receptor activity (Harbeck et al., 2019).
Breast cancer can manifest in several ways. The most common clinical feature is a lump in the breast, changes in the nipple’s size, nipple discharge and skin changes as well as infection and/or inflammation of the breast (Koo et al., 2017). Early-stage breast cancer is often asymptomatic, underscoring the importance of routine screening (Alkabban et al., 2022).
Breast cancer is generally diagnosed through screening or a symptom (pain or palpable lump) that prompts a diagnostic exam (McDonald et al., 2016). These are supplemented by imaging techniques to look for abnormalities and characterize them in more detail (McDonald et al., 2016). A breast biopsy is usually performed to confirm the presence of cancer if suspected which can also determine its specific type if the lesion is cancerous (McDonald et al., 2016). Breast cancer is staged based on the extent of the tumor, the spread to nearby lymph nodes, the spread to distant sites, estrogen receptor status, progesterone receptor status, HER2status and grade of the cancer (McDonald et al., 2016).
There are different types of breast cancer and treatment can vary based on the molecular characteristics of a patient’s disease, stage, cancer type, receptor status (Hong & Xu, 2022). Treatment usually involves a combination of different modalities and a multidisciplinary team of healthcare professionals (Hong & Xu, 2022). Surgical options range from breast-conserving procedures to mastectomy, where the entire breast is removed (Hong & Xu, 2022). Lymph node removal may also be necessary to assess the extent of cancer spread (Hong & Xu, 2022). Radiation therapy is often used after breast-conserving therapy or mastectomy (with risk factors) (Hong & Xu, 2022). Systemic chemotherapy can be administered before or after surgery, depending on the specific situation (Hong & Xu, 2022). Hormone receptor-positive breast cancers can be treated with drugs that block the effects of estrogen and progesterone. Immunotherapy is an emerging treatment option for certain breast cancers, helping the immune system recognize and attack cancer cells (Hong & Xu, 2022).
Imaging Techniques
Digital Mammography
Digital mammography is the most commonly used technique for breast cancer screening. It is a two-dimensional summation technique whereby X-rays emitted by an X-ray tube are absorbed to various degrees by tissues and measured by a detector on the other end. Denser tissues appear brighter on the resulting images than less dense tissue. The breasts are compressed while the image is acquired to spread the breast tissue over a larger surface area (Ikeda, 2011a). This reduces the overlap between different components of breast tissue, decreases the scatter of the passing X-rays, and improves contrast. Two views of each breast are usually acquired - craniocaudal (CC) and mediolateral (MLO) (Ikeda, 2011a).
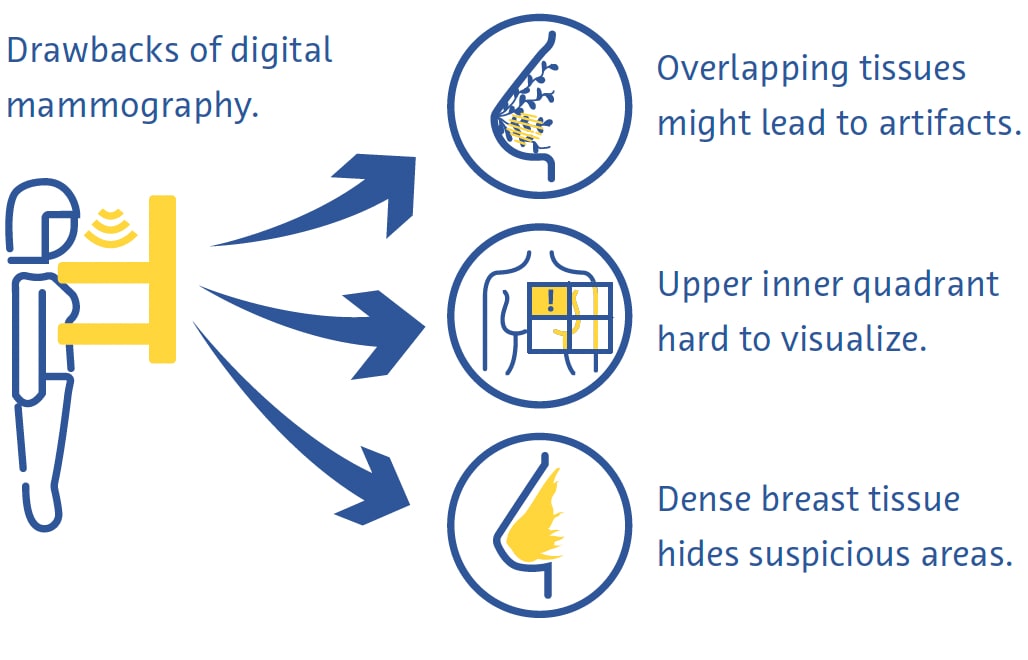
Digital mammography is a fast and useful technique for breast cancer screening, but it has its drawbacks (Ikeda, 2011a). Breast compression can be painful and overlapping of different tissues despite compression often leads to artifacts (Ikeda, 2011a). The upper inner quadrant of the breast, which is less mobile as it is fixed to the chest wall, is particularly hard to visualize on mammography (Ikeda, 2011a). Cancer can also be very hard to see on mammography in breasts with a large proportion of dense tissue (Ikeda, 2011a).
Digital Breast Tomosynthesis
Digital breast tomosynthesis (DBT) involves image acquisition utilizing an x-ray source which moves along an arc of excursion. Thin slices are reconstructed allowing for 3D imaging capabilities which are meant to minimize the influence of overlapping breast tissue and is particularly useful for imaging breast lesions located in heterogeneously dense breast parenchyma. One study found that DBT is more sensitive for the detection of breast cancer than digital mammography (DM). DBT can be combined with DM and one study found that using a combination of these techniques improves detection of breast cancer (Alabousi et al., 2020; Lei et al., 2014; Skaane et al., 2019), and can be combined with mammography. However, DBT takes longer to acquire than mammography and suffers from motion and other artifacts (Tirada et al., 2019).
Ultrasound
In diagnostic ultrasound, a transducer emits high-frequency sound waves that travel through tissues, bouncing off them and creating “echoes” that are reflected back onto and detected by the transducer. These echoes are then processed to create real-time images on a monitor based on the time it takes for the echoes to travel to the tissues and back. It is a safe and relatively low-cost technique that is often used as an adjunct to mammography (Ikeda, 2011b), especially for further evaluating a palpable or mammographic finding.
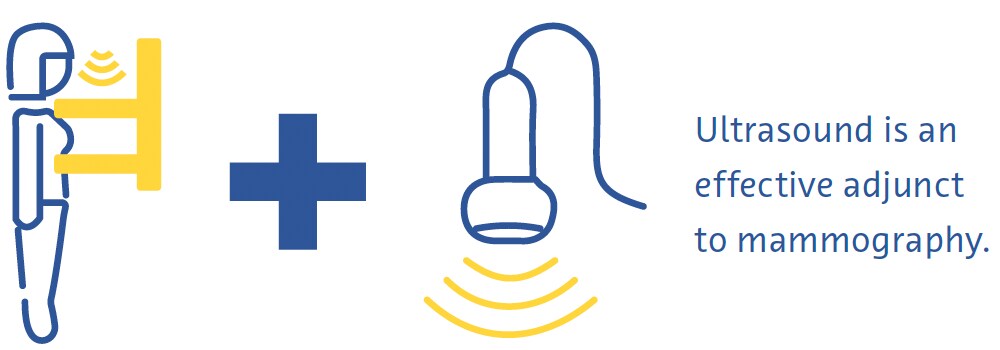
It can even be used as a primary screening modality in women under the age of 30 years or in pregnant or lactating women (Dixon, 2008; Ikeda, 2011b). Ultrasound is very useful in clarifying if a mass is cystic or solid, what kinds of margins it has, and its vascularity (Dixon, 2008; Ikeda, 2011b). It also helps detect other masses and suspicious axillary lymph nodes (Dixon, 2008; Ikeda, 2011b). Its main drawback is that the quality of the examination is highly operator-dependent (Dixon, 2008; Ikeda, 2011b).
Magnetic Resonance Imaging
Using a powerful magnetic field and a series of radiofrequency waves, magnetic resonance imaging (MRI) perturbs hydrogen nuclei in tissues to create detailed cross-sectional images of the body (Daniel & Ikeda, 2011; Mann et al., 2019). Because tissues with different compositions respond to this perturbation in different ways, MRI can detect even subtle differences between types of soft tissue very well and is considered the most sensitive modality to diagnose breast cancer (Daniel & Ikeda, 2011; Mann et al., 2019). It is mostly used for screening high-risk patients based on genetic or acquired risk factors (Daniel & Ikeda, 2011).
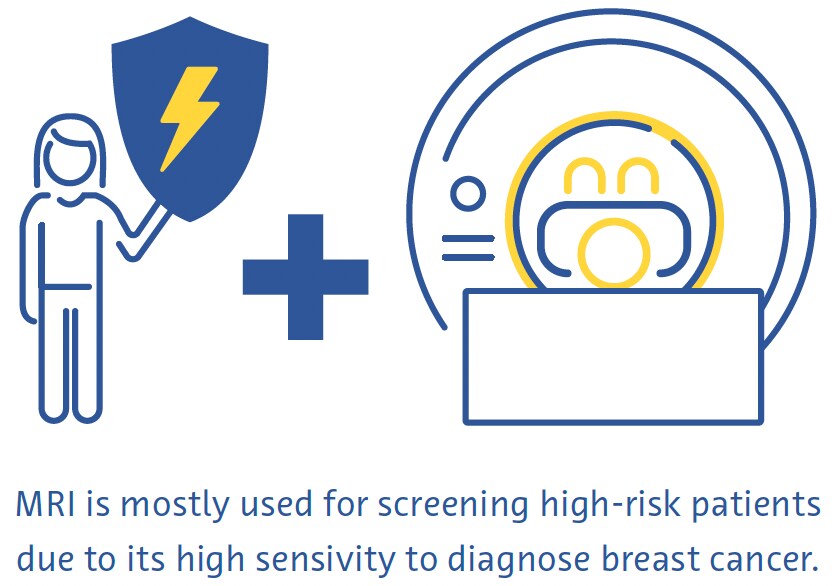
Breast MRI requires dedicated breast coils that transmit the radiofrequency waves and receive the generated signal. Images are often acquired with an in-plane spatial resolution of 1 mm, a slice thickness of under 3 mm, and suppression of signal from fat. Commonly used sequences include T2-weighted images, diffusion-weighted imaging, and dynamic contrast-enhanced MRI. To reduce false positives due to non-specific breast parenchymal changes, it is best performed between days 7 and 13 of the menstrual cycle (Daniel & Ikeda, 2011).
Unlike mammography, MRI does not involve the use of ionizing radiation and produces 3-dimensional images that facilitate the detection of very small lesions (DeMartini & Lehman, 2008; Shahid et al., 2016). MRI also allows a more detailed assessment of the chest wall than mammography and ultrasound (DeMartini & Lehman, 2008). Drawbacks of breast MRI include a low sensitivity to microcalcifications, high cost, and the fact it is contraindicated in people with certain metallic implants (Daniel & Ikeda, 2011).
Screening and Diagnostic Challenges
Despite evidence for the overall benefit of breast cancer screening (Dibden et al., 2020; Kalager et al., 2010; Tabár et al., 2019), it suffers from several technical and logistical challenges. More than half of women screened annually for 10 years will have a false positive test (Hubbard et al., 2011). This has wide-ranging and significant consequences including the physical and emotional burden of unnecessary biopsies and increased healthcare costs (Nelson, Pappas, et al., 2016; Ong & Mandl, 2015). Screening also often misses breast cancer, particularly in women with dense breasts (Banks et al., 2006).
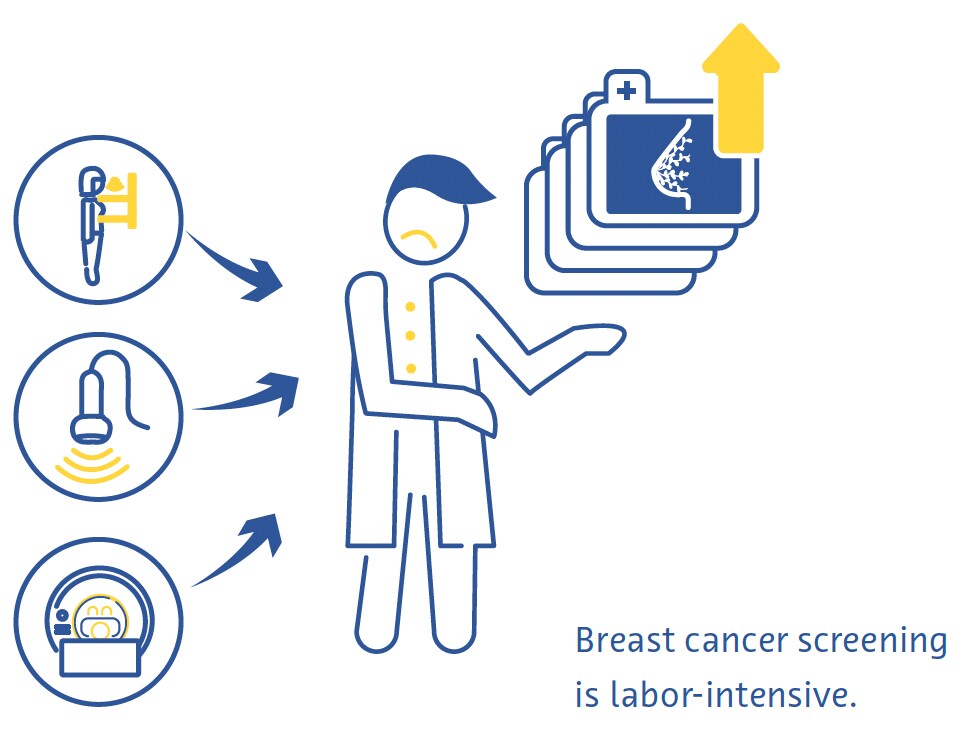
Breast cancer screening requires highly skilled workers, including radiologists and radiographers, of whom there is currently a global shortage (Moran & Warren-Forward, 2012; Rimmer, 2017; Wing & Langelier, 2009). This problem is compounded by the fact that the standard of care in breast screening in many European countries is that each examination is read by two radiologists independently (Giordano et al., 2012) and that, in certain countries, such as the United States, the barriers for qualifying to interpret mammograms are high because of stringent professional certification standards (Food and Drug Administration, 2001).
There are also substantial barriers to the uptake of breast cancer screening worldwide. These include lack of or difficult access to screening programs, lack of knowledge or misunderstanding of the benefits of these programs, and social and cultural barriers (Mascara & Constantinou, 2021).
Role of Artificial Intelligence
Technical Improvements
Few published studies have thus far directly investigated the use of AI to make technical improvements to breast examinations. One commercially available application provides real-time feedback to radiographers on the adequacy of patient positioning on mammograms. (Volpara Health, 2022). Other AI applications have focused on reducing radiation doses (J. Liu et al., 2018), improving image reconstruction (Kim et al., 2016), and reducing noise and artifacts on DBT (Garrett et al., 2018).
DBT is frequently combined with digital mammography for breast cancer screening, which doubles the radiation dose received by the patient (Svahn et al., 2015). To avoid this, there has been increasing interest in generating synthetic mammograms from DBT data (Chikarmane et al., 2023). In a large prospective Norwegian study, the accuracies of DBT combined with either digital mammography or synthetic mammography for breast cancer detection were very similar (Skaane et al., 2019). Recent studies have investigated improving the quality of synthetic mammography using AI, with promising results (Balleyguier et al., 2017; James et al., 2018).
Diagnostic Improvements
Breast Density Assessment
Dense breast tissue that is seen on mammography represents fibroglandular tissue. Women with dense breasts have a 2-to-4-fold higher risk of breast cancer than women with breasts with more fatty breast tissue (Byrne et al., 1995; Duffy et al., 2018; Torres-Mejía et al., 2005). In addition, the sensitivity of mammography for breast cancer is 20–30 % lower in dense breasts than in less dense breasts (Lynge et al., 2019). The standard-of- care in breast density assessment uses the BI-RADS classification (Berg et al., 2000).
Several large studies have investigated the potential for automatic assessment of breast density on mammograms using AI-based tools. A convolutional neural network (CNN) trained on 14,000 mammograms and tested on almost 2000 mammograms classified breast density into either “scattered density” or “heterogeneously dense” with an area-under-the-curve (AUC) of 0.93 (Mohamed et al., 2018). Another study used a CNN capable of both binary and four-way BI-RADS classification and trained on more than 40,000 mammograms (Lehman et al., 2019). In a test dataset of more than 8,000 mammograms, they found good agreement on breast density between the algorithm and individual radiologists (kappa = 0.67) as well as the consensus of five radiologists (kappa = 0.78) (Lehman et al., 2019).
Breast Cancer Detection
In a systematic review that included 82 studies using AI for breast cancer detection with various reference standards, the authors found an AUC of 0.87 for studies using mammography, 0.91 using ultrasound, 0.91 using DBT, and 0.87 using MRI (Aggarwal et al., 2021). These are promising results, however, head-to-head comparisons between AI-based algorithms and radiologists reveal room for improvement. In another systematic review of studies using either histopathology or follow-up (for screen negative women) as a reference, 94 % of the 36 CNNs identified were less accurate than a single radiologist, and all were less accurate than the consensus of 2 or more radiologists when used as a standalone system (Freeman et al., 2021). Current evidence, therefore, does not support the use of AI as a standalone strategy for breast cancer detection.
Breast Cancer Prediction
AI has shown promise for predicting the risk of developing breast cancer based on screening mammograms, either by providing a better assessment of breast density, an established risk factor for breast cancer (Duffy et al., 2018), or detecting subtle imaging features that are harbingers of cancer (Batchu et al., 2021). Several studies have used AI-based models to predict the risk of developing breast cancer in the future based on mammograms (Batchu et al., 2021; Geras et al., 2019).
A CNN trained on almost 1,000,000 mammographic images showed an AUC of 0.65 for predicting future development of breast cancer breast cancer compared to 0.57–0.60 for conventional mammography-based breast density scores (Dembrower, Liu, et al., 2020). A smaller study found an AUC of 0.73 for a CNN-based method to predict breast cancer from normal mammographic images. (Arefan et al., 2020). Another deep learning algorithm showed an AUC of 0.82 for predicting interval cancers (cancers detected within 12 months after a negative mammogram) compared to 0.65 for BI-RADS visual assessment of breast density (Hinton et al., 2019). Another deep-learning-based model that incorporated both risk factors and mammographic findings for predicting breast cancer risk had an AUC of up to 0.7, surpassing the accuracy of predictive models based on risk factors or mammographic findings alone. (Yala, Lehman, et al., 2019).
Efficiency Improvements
The sheer volume of mammographic examinations and the shortage of trained radiologists have made efficiency improvements one of the most interesting areas of research into the use of AI in breast cancer.
In one study, the authors simulated a workflow in which mammograms were interpreted by a radiologist and a deep learning model, with the decision being considered final if both agreed (McKinney et al., 2020). A second radiologist was only consulted in case of disagreement, and this was associated with an 88 % reduction in workload for the second radiologist with a negative predictive value of over 99.9 % (McKinney et al., 2020).
In a first-of-kind large randomized clinical trial from Sweden, approximately 80,000 women were assigned to have their screening mammograms either pre-read by a CNN or not (Lång et al., 2023). In the intervention arm, only mammograms assigned a high likelihood-of-malignancy score were double-read (the rest were read by one radiologist) and the results were compared to conventional double-reading without the help of the algorithm. In an interim analysis of data from the 80,000 women, both study arms showed an identical false-positive rate of 1.5 %. The positive predictive value of recall was 28.3 % in the intervention group and 24.8 % in the control group and the strategy reduced workload by 44.3 % (Lång et al., 2023).
Other studies have used AI to prescreen mammograms, triaging out those with a low likelihood of cancer, and showing only those with a high probability of cancer to a radiologist. One study from the US used a simulated workflow involving a CNN trained on more than 212,000 mammograms and tested on over 26,000 for this purpose (Yala, Schuster, et al., 2019). The workflow using the algorithm had a non-inferior sensitivity to breast cancer (90.1 % vs 90.6 %) and a slightly higher specificity compared to radiologists working alone (94.2 % vs 93.5 %) and was associated with a 19.3 % lower workload (Yala, Schuster, et al., 2019). A smaller study from Spain found a 72.5 % decrease in workload using AI to triage only high risk DBT cases for a second radiologist read and 29.7 % using AI to triage only high risk DBT studies to a second radiologist to read when compared to traditional double read mammography workflows (Raya-Povedano et al., 2021). They also found a non-inferior sensitivity of this strategy of using AI to triage high risk mammography and DBT cases for a second read in comparison to standard double reading mammography and DBT workflows (Raya-Povedano et al., 2021). In a Swedish study, a similar strategy using a commercially available AI algorithm yielded a false-negative rate of no higher than 4 % and the algorithm demonstrated the ability to possibly detect an additional 71 cancers per 1000 examinations more than a negative double-reading by human radiologists in patients noted to be at a very high risk by the AI algorithm (Dembrower, Wåhlin, et al., 2020).
In a study of over one million mammograms across eight screening sites and three device manufacturers, a commercially available deep learning algorithm triaged 63 % to no further workup based on high-confidence assessments of the examinations (Leibig et al., 2022). The rest of the examinations, in which the algorithm’s confidence was low, were shown to radiologists. This strategy improved the sensitivity of the radiologists (compared to unaided reading) by 2.6–4 % and the specificity by 0.5–1.0 % (Leibig et al., 2022).
Challenges and Future Directions
Several ethical, technical, and methodological challenges associated with the use of AI in breast cancer screening provide a framework for guiding future research on this topic (Hickman et al., 2021).
Most AI-based tools have thus far focused on digital mammography (Aggarwal et al., 2021), but other exam techniques such as DBT and MRI have unique advantages (Alsheik et al., 2019; Mann et al., 2019) and are likely to play larger roles in breast cancer screening in the future. However, because DBT and MRI are tomographic techniques producing 3-dimensional outputs, processing them using AI-based tools will require more storage space and computing power (Prevedello et al., 2019).
The incidence, presentation, and outcome of breast cancer are related to several sociodemographic factors, including race and ethnicity (Hirko et al., 2022; Hu et al., 2019; Martini et al., 2022). Training AI-based tools on datasets that represent a diverse population is key to ensuring that they can generalize and benefit as many people as possible.
The overall performance of AI for breast cancer detection has been impressive. However, it is noteworthy that noninferior sensitivity of the AI to that of radiologists for detecting breast cancer could not be proven in one study (Lauritzen et al., 2022). In addition, the quality of evidence behind many studies on this topic is concerning. A systematic review investigating the accuracy of AI-based tools for breast cancer detection identified several areas of potential improvement (Freeman et al., 2021). The review found no prospective studies and the identified studies were of poor methodological quality.
In particular, the authors observed that smaller studies showed more positive results that were not replicated in larger studies. In another systematic review, only about one-tenth of studies used an external dataset for validation, no studies provided a prespecified sample size calculation, and serious issues with selection bias and inappropriate reference standards were identified (Aggarwal et al., 2021). These methodological issues can potentially be mitigated in the future with the introduction of large open data repositories (Nguyen et al., 2023) and increased adherence to guidelines for conducting AI-based medical research (Lekadir et al., 2021; X. Liu et al., 2020).
Conclusion
Integrating artificial intelligence in breast screening programs holds promise for enhancing image quality, improving efficiency, and predicting future breast cancer risk. For detecting breast cancer on screening examinations, evidence suggests that Artificial Intelligence works best when working synergistically with radiologists. Ongoing research is crucial to address challenges associated with the use of AI in breast cancer screening, including expanding its applications beyond mammography and ensuring its ethical and responsible use. With the continuous evolution of AI applications, the future of breast cancer screening holds immense potential for increased accessibility, early intervention, and ultimately, improved outcomes for patients.
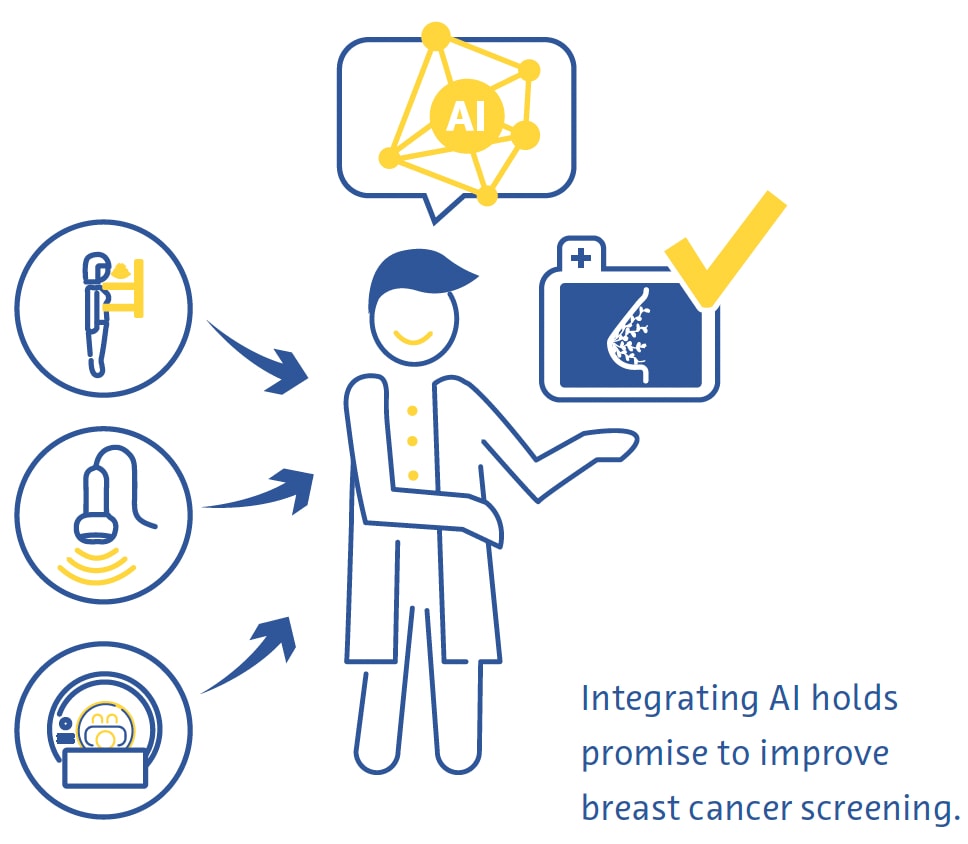
References
Aggarwal, R., Sounderajah, V., Martin, G., Ting, D. S. W., Karthikesalingam, A., King, D., Ashrafian, H., & Darzi, A. (2021). Diagnostic accuracy of deep learning in medical imaging: a systematic review and meta-analysis. NPJ Digital Medicine, 4(1), 65.
Alabousi, M., Zha, N., Salameh, J.-P., Samoilov, L., Sharifabadi, A. D., Pozdnyakov, A., Sadeghirad, B., Freitas, V., McInnes, M. D. F., & Alabousi, A. (2020). Digital breast tomosynthesis for breast cancer detection: a diagnostic test accuracy systematic review and meta-analysis. European Radiology, 30(4), 2058–2071.
Alkabban FM and Ferguson T. (2022). Breast Cancer, 2022. In: StatPearls [Internet]. Treasure Island (FL): StatPearls Publishing.
Alsheik, N. H., Dabbous, F., Pohlman, S. K., Troeger, K. M., Gliklich, R. E., Donadio, G. M., Su, Z., Menon, V., & Conant, E. F. (2019). Comparison of Resource Utilization and Clinical Outcomes Following Screening with Digital Breast Tomosynthesis Versus Digital Mammography: Findings From a Learning Health System. Academic Radiology, 26(5), 597–605.
Arefan, D., Mohamed, A. A., Berg, W. A., Zuley, M. L., Sumkin, J. H., & Wu, S. (2020). Deep learning modeling using normal mammograms for predicting breast cancer risk. Medical Physics, 47(1), 110–118.
Balleyguier, C., Arfi-Rouche, J., Levy, L., Toubiana, P. R., Cohen-Scali, F., Toledano, A. Y., & Boyer, B. (2017). Improving digital breast tomosynthesis reading time: A pilot multi-reader, multi-case study using concurrent Computer-Aided Detection (CAD). European Journal of Radiology, 97, 83–89.
Banks, E., Reeves, G., Beral, V., Bull, D., Crossley, B., Simmonds, M., Hilton, E., Bailey, S., Barrett, N., Briers, P., English, R., Jackson, A., Kutt, E., Lavelle, J., Rockall, L., Wallis, M. G., Wilson, M., & Patnick, J. (2006). Hormone replacement therapy and false positive recall in the Million Women Study: patterns of use, hormonal constituents and consistency of effect. Breast Cancer Research: BCR, 8(1), R8.
Batchu, S., Liu, F., Amireh, A., Waller, J., & Umair, M. (2021). A Review of Applications of Machine Learning in Mammography and Future Challenges. Oncology, 99(8), 483–490. https://doi.org/10.1159/000515698
Bazira, P. J., Ellis, H., & Mahadevan, V. (2022). Anatomy and physiology of the breast. Surgery, 40(2), 79–83. https://doi.org/10.1016/j.mpsur.2021.11.015
Berg, W. A., Campassi, C., Langenberg, P., & Sexton, M. J. (2000). Breast Imaging Reporting and Data System: interand intraobserver variability in feature analysis and final assessment. AJR. American Journal of Roentgenology, 174(6), 1769–1777.
Boyd, N. F., Guo, H., Martin, L. J., Sun, L., Stone, J., Fishell, E., Jong, R. A., Hislop, G., Chiarelli, A., Minkin, S., & Yaffe, M. J. (2007). Mammographic density and the risk and detection of breast cancer. The New England Journal of Medicine, 356(3), 227–236.
Bray, F., Ferlay, J., Soerjomataram, I., Siegel, R. L., Torre, L. A., & Jemal, A. (2018). Global cancer statistics 2018: GLOBOCAN estimates of incidence and mortality worldwide for 36 cancers in 185 countries. CA: A Cancer Journal for Clinicians, 68(6), 394–424.
Breast Cancer Statistics. (2020, October 27). Susan G. Komen®. https://www.komen.org/breast-cancer/facts-statistics/breastcancer- statistics/
Byrne, C., Schairer, C., Wolfe, J., Parekh, N., Salane, M., Brinton, L. A., Hoover, R., & Haile, R. (1995). Mammographic features and breast cancer risk: effects with time, age, and menopause status. Journal of the National Cancer Institute, 87(21), 1622–1629.
Chikarmane, S. A., Offit, L. R., & Giess, C. S. (2023). Synthetic Mammography: Benefits, Drawbacks, and Pitfalls. Radiographics: A Review Publication of the Radiological Society of North America, Inc, 43(10), e230018. https://doi.org/10.1148/rg.230018
Daniel, B. L., & Ikeda, D. M. (2011). Chapter 7 - Magnetic Resonance Imaging of Breast Cancer and MRI-Guided Breast Biopsy. In D. M. Ikeda (Ed.), Breast Imaging (Second Edition) (pp. 239–296). Mosby.
DeMartini W & Lehman C, (2008). Top Magn Reson Imaging, Jun;19(3):143-50.
Dembrower, K., Liu, Y., Azizpour, H., Eklund, M., Smith, K., Lindholm, P., & Strand, F. (2020). Comparison of a Deep Learning Risk Score and Standard Mammographic Density Score for Breast Cancer Risk Prediction. Radiology, 294(2), 265–272.
Dembrower, K., Wåhlin, E., Liu, Y., Salim, M., Smith, K., Lindholm, P., Eklund, M., & Strand, F. (2020). Effect of artificial intelligence-based triaging of breast cancer screening mammograms on cancer detection and radiologist workload: a retrospective simulation study. The Lancet. Digital Health, 2(9), e468–e474.
Dibden, A., Offman, J., Duffy, S. W., & Gabe, R. (2020). Worldwide Review and Meta-Analysis of Cohort Studies Measuring the Effect of Mammography Screening Programmes on Incidence-Based Breast Cancer Mortality. Cancers, 12(4). https://doi.org/10.3390/cancers12040976
Duffy, S. W., Morrish, O. W. E., Allgood, P. C., Black, R., Gillan, M. G. C., Willsher, P., Cooke, J., Duncan, K. A., Michell, M. J., Dobson, H. M., Maroni, R., Lim, Y. Y., Purushothaman, H. N., Suaris, T., Astley, S. M., Young, K. C., Tucker, L., & Gilbert, F. J. (2018). Mammographic density and breast cancer risk in breast screening assessment cases and women with a family history of breast cancer. European Journal of Cancer, 88, 48–56.
Food and Drug Administration. (2001). The Mammography Quality Standards Act Final Regulations: Preparing for MQSA Inspections; Final Guidance for Industry and FDA.
Freeman, K., Geppert, J., Stinton, C., Todkill, D., Johnson, S., Clarke, A., & Taylor-Phillips, S. (2021). Use of artificial intelligence for image analysis in breast cancer screening programmes: systematic review of test accuracy. BMJ, 374, n1872.
Freer, P. E. (2015). Mammographic breast density: impact on breast cancer risk and implications for screening. Radiographics: A Review Publication of the Radiological Society of North America, Inc, 35(2), 302–315.
Garrett, J. W., Li, Y., Li, K., & Chen, G.-H. (2018). Reduced anatomical clutter in digital breast tomosynthesis with statistical iterative reconstruction. Medical Physics, 45(5), 2009–2022.
Geras, K. J., Mann, R. M., & Moy, L. (2019). Artificial Intelligence for Mammography and Digital Breast Tomosynthesis: Current Concepts and Future Perspectives. Radiology, 293(2), 246–259. https://doi.org/10.1148/radiol.2019182627
Giordano, L., von Karsa, L., Tomatis, M., Majek, O., de Wolf, C., Lancucki, L., Hofvind, S., Nyström, L., Segnan, N., Ponti, A., Eunice Working Group, Van Hal, G., Martens, P., Májek, O., Danes, J., von Euler-Chelpin, M., Aasmaa, A., Anttila, A., Becker, N., … Suonio, E. (2012). Mammographic screening programmes in Europe: organization, coverage and participation. Journal of Medical Screening, 19 Suppl 1, 72–82.
Harbeck, N., Penault-Llorca, F., Cortes, J., Gnant, M., Houssami, N., Poortmans, P., Ruddy, K., Tsang, J., & Cardoso, F. (2019). Breast cancer. Nature Reviews. Disease Primers, 5(1), 66. https://doi.org/10.1038/s41572-019-0111-2
Hickman, S. E., Baxter, G. C., & Gilbert, F. J. (2021). Adoption of artificial intelligence in breast imaging: evaluation, ethical constraints and limitations. British Journal of Cancer, 125(1), 15–22.
Hinton, B., Ma, L., Mahmoudzadeh, A. P., Malkov, S., Fan, B., Greenwood, H., Joe, B., Lee, V., Kerlikowske, K., & Shepherd, J. (2019). Deep learning networks find unique mammographic differences in previous negative mammograms between interval and screen-detected cancers: a case-case study. Cancer Imaging: The Official Publication of the International Cancer Imaging Society, 19(1), 41.
Hirko, K. A., Rocque, G., Reasor, E., Taye, A., Daly, A., Cutress, R. I., Copson, E. R., Lee, D.-W., Lee, K.-H., Im, S.-A., & Park, Y. H. (2022). The impact of race and ethnicity in breast cancer-disparities and implications for precision oncology.BMC Medicine, 20(1), 72.
Hong, R., & Xu, B. (2022). Breast cancer: an up-to-date review and future perspectives. Cancer Communications, 42(10), 913–936. https://doi.org/10.1002/cac2.12358
Hubbard, R. A., Kerlikowske, K., Flowers, C. I., Yankaskas, B. C., Zhu, W., & Miglioretti, D. L. (2011). Cumulative probability of false-positive recall or biopsy recommendation after 10 years of screening mammography: a cohort study.Annals of Internal Medicine, 155(8), 481–492.
Hu, K., Ding, P., Wu, Y., Tian, W., Pan, T., & Zhang, S. (2019). Global patterns and trends in the breast cancer incidence and mortality according to sociodemographic indices: an observational study based on the global burden of diseases. BMJ Open, 9(10), e028461.
Ikeda, D. M. (Ed.). (2011a). Chapter 2 - Mammogram Interpretation. In Breast Imaging (Second Edition) (pp. 24–62). Mosby.
Ikeda, D. M. (Ed.). (2011b). Chapter 5 - Breast Ultrasound. In Breast Imaging (Second Edition) (pp. 149–193). Mosby.
James, J. J., Giannotti, E., & Chen, Y. (2018). Evaluation of a computer-aided detection (CAD)-enhanced 2D synthetic mammogram: comparison with standard synthetic 2D mammograms and conventional 2D digital mammography. Clinical Radiology, 73(10), 886–892.
Kalager, M., Zelen, M., Langmark, F., & Adami, H.-O. (2010). Effect of screening mammography on breast-cancer mortality in Norway. The New England Journal of Medicine, 363(13), 1203–1210. https://doi.org/10.1056/NEJMoa1000727
Kim, Y.-S., Park, H.-S., Lee, H.-H., Choi, Y.-W., Choi, J.-G., Kim, H. H., & Kim, H.-J. (2016). Comparison study of reconstruction algorithms for prototype digital breast tomosynthesis using various breast phantoms. La Radiologia Medica, 121(2), 81–92.
Koo, M. M., von Wagner, C., Abel, G. A., McPhail, S., Rubin, G. P., & Lyratzopoulos, G. (2017). Typical and atypical presenting symptoms of breast cancer and their associations with diagnostic intervals: Evidence from a national audit of cancer diagnosis. Cancer Epidemiology, 48, 140–146. https://doi.org/10.1016/j.canep.2017.04.010
Lång, K., Josefsson, V., Larsson, A.-M., Larsson, S., Högberg, C., Sartor, H., Hofvind, S., Andersson, I., & Rosso, A. (2023). Artificial intelligence-supported screen reading versus standard double reading in the Mammography Screening with Artificial Intelligence trial (MASAI): a clinical safety analysis of a randomised, controlled, non-inferiority, single-blinded, screening accuracy study. The Lancet Oncology, 24(8), 936–944.
Lauritzen, A. D., Rodríguez-Ruiz, A., von Euler-Chelpin, M. C., Lynge, E., Vejborg, I., Nielsen, M., Karssemeijer, N., & Lillholm, M. (2022). An Artificial Intelligence-based Mammography Screening Protocol for Breast Cancer: Outcome and Radiologist Workload.Radiology, 304(1), 41–49.
Lehman, C. D., Yala, A., Schuster, T., Dontchos, B., Bahl, M., Swanson, K., & Barzilay, R. (2019). Mammographic Breast Density Assessment Using Deep Learning: Clinical Implementation. Radiology, 290(1), 52–58.
Leibig, C., Brehmer, M., Bunk, S., Byng, D., Pinker, K., & Umutlu, L. (2022). Combining the strengths of radiologists and AI for breast cancer screening: a retrospective analysis. The Lancet. Digital Health, 4(7), e507–e519.
Lei, J., Yang, P., Zhang, L., Wang, Y., & Yang, K. (2014). Diagnostic accuracy of digital breast tomosynthesis versus digital mammography for benign and malignant lesions in breasts: a meta-analysis. European Radiology, 24(3), 595–602.
Lekadir, K., Osuala, R., Gallin, C., Lazrak, N., Kushibar, K., Tsakou, G., Aussó, S., Alberich, L. C., Marias, K., Tsiknakis, M., Colantonio, S., Papanikolaou, N., Salahuddin, Z., Woodruff, H. C., Lambin, P., & Martí-Bonmatí, L. (2021). FUTURE-AI: Guiding Principles and Consensus Recommendations for Trustworthy Artificial Intelligence in Medical Imaging. In arXiv [cs.CV].arXiv. https://arxiv.org/abs/2109.09658
Liu, J., Zarshenas, A., Qadir, A., Wei, Z., Yang, L., Fajardo, L., & Suzuki, K. (2018). Radiation dose reduction in digital breast tomosynthesis (DBT) by means of deep-learning-based supervised image processing. Medical Imaging 2018: Image Processing, 10574, 89–97.
Liu, X., Cruz Rivera, S., Moher, D., Calvert, M. J., Denniston, A. K., & SPIRIT-AI and CONSORT-AI Working Group. (2020). Reporting guidelines for clinical trial reports for interventions involving artificial intelligence: the CONSORT-AI extension. Nature Medicine, 26(9), 1364–1374.
Łukasiewicz, S., Czeczelewski, M., Forma, A., Baj, J., Sitarz, R., & Stanisławek, A. (2021). Breast Cancer-Epidemiology, Risk Factors, Classification, Prognostic Markers, and Current Treatment Strategies-An Updated Review. Cancers, 13(17). https://doi.org/10.3390/cancers13174287
Lynge, E., Vejborg, I., Andersen, Z., von Euler-Chelpin, M., & Napolitano, G. (2019). Mammographic Density and Screening Sensitivity, Breast Cancer Incidence and Associated Risk Factors in Danish Breast Cancer Screening. Journal of Clinical Medicine Research, 8(11). https://doi.org/10.3390/jcm8112021
Mann, R. M., Cho, N., & Moy, L. (2019). Breast MRI: State of the Art. Radiology, 292(3), 520–536.
Martini, R., Newman, L., & Davis, M. (2022). Breast cancer disparities in outcomes; unmasking biological determinants associated with racial and genetic diversity. Clinical & Experimental Metastasis, 39(1), 7–14.
Mascara, M., & Constantinou, C. (2021). Global Perceptions of Women on Breast Cancer and Barriers to Screening. Current Oncology Reports, 23(7), 74.
McDonald, E. S., Clark, A. S., Tchou, J., Zhang, P., & Freedman, G. M. (2016). Clinical Diagnosis and Management of Breast Cancer. Journal of Nuclear Medicine: Official Publication, Society of Nuclear Medicine, 57 Suppl 1, 9S – 16S. https://doi.org/10.2967/jnumed.115.157834
McKinney, S. M., Sieniek, M., Godbole, V., Godwin, J., Antropova, N., Ashrafian, H., Back, T., Chesus, M., Corrado, G. S., Darzi, A., Etemadi, M., Garcia-Vicente, F., Gilbert, F. J., Halling-Brown, M., Hassabis, D., Jansen, S., Karthikesalingam, A., Kelly, C. J., King, D., … Shetty, S. (2020). International evaluation of an AI system for breast cancer screening. Nature, 577(7788), 89–94.
Mohamed, A. A., Berg, W. A., Peng, H., Luo, Y., Jankowitz, R. C., & Wu, S. (2018). A deep learning method for classifying mammographic breast density categories. Medical Physics, 45(1), 314–321.
Moran, S., & Warren-Forward, H. (2012). The Australian BreastScreen workforce: a snapshot. The Radiographer, 59(1), 26–30.
Nelson, H. D., Fu, R., Cantor, A., Pappas, M., Daeges, M., & Humphrey, L. (2016). Effectiveness of Breast Cancer Screening: Systematic Review and Meta-analysis to Update the 2009 U.S. Preventive Services Task Force Recommendation. Annals of Internal Medicine, 164(4), 244–255.
Nelson, H. D., Pappas, M., Cantor, A., Griffin, J., Daeges, M., & Humphrey, L. (2016). Harms of Breast Cancer Screening: Systematic Review to Update the 2009 U.S. Preventive Services Task Force Recommendation. Annals of Internal Medicine, 164(4), 256–267.
Nguyen, H. T., Nguyen, H. Q., Pham, H. H., Lam, K., Le, L. T., Dao, M., & Vu, V. (2023). VinDr-Mammo: A large-scale benchmark dataset for computer-aided diagnosis in full-field digital mammography. Scientific Data, 10(1), 277.
Ong, M.-S., & Mandl, K. D. (2015). National expenditure for false-positive mammograms and breast cancer overdiagnoses estimated at $4 billion a year. Health Affairs , 34(4), 576–583.
Prevedello, L. M., Halabi, S. S., Shih, G., Wu, C. C., Kohli, M. D., Chokshi, F. H., Erickson, B. J., Kalpathy-Cramer, J., Andriole, K. P., & Flanders, A. E. (2019). Challenges Related to Artificial Intelligence Research in Medical Imaging and the Importance of Image Analysis Competitions. Radiology. Artificial Intelligence, 1(1), e180031. https://doi.org/10.1148/ ryai.2019180031
Raya-Povedano, J. L., Romero-Martín, S., Elías-Cabot, E., Gubern-Mérida, A., Rodríguez-Ruiz, A., & Álvarez-Benito, M. (2021). AI-based Strategies to Reduce Workload in Breast Cancer Screening with Mammography and Tomosynthesis: A Retrospective Evaluation. Radiology, 300(1), 57–65.
Rimmer, A. (2017). Radiologist shortage leaves patient care at risk, warns royal college. BMJ, 359, j4683.
Skaane, P., Bandos, A. I., Niklason, L. T., Sebuødegård, S., Østerås, B. H., Gullien, R., Gur, D., & Hofvind, S. (2019). Digital Mammography versus Digital Mammography Plus Tomosynthesis in Breast Cancer Screening: The Oslo Tomosynthesis Screening Trial. Radiology, 291(1), 23–30.
Sung H et al,(2021). CA Cancer J Clin, 2021 May;71(3):209-249. doi: 10.3322/caac.21660
Tabár, L., Dean, P. B., Chen, T. H.-H., Yen, A. M.-F., Chen, S. L.- S., Fann, J. C.-Y., Chiu, S. Y.-H., Ku, M. M.-S., Wu, W. Y.-Y., Hsu, C.-Y., Chen, Y.-C., Beckmann, K., Smith, R. A., & Duffy, S. W. (2019). The incidence of fatal breast cancer measures the increased effectiveness of therapy in women participating in mammography screening. Cancer, 125(4), 515–523.
Svahn, T. M., Houssami, N., Sechopoulos, I., & Mattsson, S. (2015). Review of radiation dose estimates in digital breast tomosynthesis relative to those in two-view full-field digital mammography. Breast, 24(2), 93–99. https://doi.org/10.1016/j. breast.2014.12.002
Tirada, N., Li, G., Dreizin, D., Robinson, L., Khorjekar, G., Dromi, S., & Ernst, T. (2019). Digital Breast Tomosynthesis: Physics, Artifacts, and Quality Control Considerations. Radiographics: A Review Publication of the Radiological Society of North America, Inc, 39(2), 413–426.
Torres-Mejía, G., De Stavola, B., Allen, D. S., Pérez-Gavilán, J. J., Ferreira, J. M., Fentiman, I. S., & Dos Santos Silva, I. (2005). Mammographic features and subsequent risk of breast cancer: a comparison of qualitative and quantitative evaluations in the Guernsey prospective studies. Cancer Epidemiology, Biomarkers & Prevention: A Publication of the American Association for Cancer Research, Cosponsored by the American Society of Preventive Oncology, 14(5), 1052–1059.
Volpara Health. (2022). TruPGMI: AI for mammography quality improvement. https://www.volparahealth.com/breast-healthsoftware/ products/analytics/
Wing, P., & Langelier, M. H. (2009). Workforce shortages in breast imaging: impact on mammography utilization. AJR. American Journal of Roentgenology, 192(2), 370–378.
Yala, A., Lehman, C., Schuster, T., Portnoi, T., & Barzilay, R. (2019). A Deep Learning Mammography-based Model for Improved Breast Cancer Risk Prediction. Radiology, 292(1), 60–66.
Yala, A., Schuster, T., Miles, R., Barzilay, R., & Lehman, C. (2019). A Deep Learning Model to Triage Screening Mammograms: A Simulation Study. Radiology, 293(1), 38–46.