- Il carcinoma mammario
- Tecniche di imaging
- Screening e problemi di diagnostica
- Il ruolo dell'intelligenza artificiale
- Problemi e orientamenti futuri
- Conclusione
- Bibliografia
Il carcinoma mammario
Il carcinoma mammario è il tipo specifico di cancro più comune tra le donne a livello globale (Sung et. al., 2021). Nella popolazione femminile, il carcinoma mammario rappresenta 1 caso di cancro su 4 e 1 decesso per cancro su 6, classificandosi al primo posto nella stragrande maggioranza dei Paesi (159 su 185 Paesi) per incidenza e in 110 Paesi per mortalità (Sung et. al., 2021). La maggior parte dei casi si verifica nelle donne di età superiore ai 50 anni, ma la malattia può colpire anche le donne più giovani. Altri fattori di rischio includono: predisposizione genetica, anamnesi familiare, menarca precoce, terapia ormonale sostitutiva, consumo di alcol e obesità (Łukasiewicz et al., 2021).
Il seno è composto da lobuli secernenti latte, da un sistema di dotti galattofori e da tessuto adiposo (Bazira et al., 2021). Tutti i carcinomi mammari hanno origine nelle cellule che rivestono le unità terminali dotto-lobulari (l'unità funzionale del seno) dei dotti collettori. Il tipo più comune di carcinoma mammario maschile è il carcinoma duttale infiltrante, che si sviluppa nei dotti galattofori e invade i tessuti vicini (Harbeck et al., 2019). Lo sviluppo del carcinoma mammario è legato a mutazioni genetiche che causano una proliferazione cellulare incontrollata, nonché ai geni BRCA1 e BRCA2 coinvolti nella riparazione del DNA (Harbeck et al., 2019). I recettori degli estrogeni e del progesterone svolgono un ruolo importante a livello fisiopatologico, pertanto tutti i pazienti con tumori che esprimono questi recettori dovrebbero ricevere una terapia ormonale per bloccare l'attività dei recettori degli estrogeni (Harbeck et al., 2019).
Il carcinoma mammario può manifestarsi in diversi modi. La manifestazione clinica più comune è rappresentata da un nodulo al seno, cambiamenti nelle dimensioni del capezzolo, secrezione dal capezzolo e alterazioni cutanee, nonché da infezione e/o infiammazione mammaria (Koo et al., 2017). Il carcinoma mammario in stadio iniziale è spesso asintomatico, per cui è estremamente importante lo screening di routine (Kalager et al., 2010).
Il carcinoma mammario viene generalmente diagnosticato attraverso lo screening o un esame diagnostico eseguito a seguito del rilevamento di un sintomo (dolore o nodulo palpabile) (McDonald et al., 2016). A questi si aggiungono tecniche di imaging per cercare anomalie e caratterizzarle in modo più dettagliato (McDonald et al., 2016). Di solito, in caso di sospetto, viene eseguita una biopsia mammaria per confermare la presenza di cancro, la quale può anche determinarne il tipo specifico se la lesione è cancerosa (McDonald et al., 2016). Il carcinoma mammario viene stadiato in base all'estensione del tumore, alla diffusione ai linfonodi vicini, alla diffusione a sedi distanti, allo stato dei recettori degli estrogeni, allo stato dei recettori del progesterone, allo stato HER2 e al grado del tumore (McDonald et al., 2016).
Esistono diversi tipi di carcinoma mammario e il trattamento può variare in base alle caratteristiche molecolari della malattia, allo stadio, al tipo di cancro e allo stato dei recettori del paziente (Hong & Xu, 2022). Il trattamento di solito prevede una combinazione di diverse modalità e l’intervento di un team multidisciplinare di professionisti sanitari (Hong & Xu, 2022). Le opzioni chirurgiche spaziano dagli interventi con conservazione del seno alla mastectomia, che ne prevede la rimozione integrale (Hong & Xu, 2022). Potrebbe anche essere necessaria l’asportazione di linfonodi per valutare l’entità della diffusione del cancro (Hong & Xu, 2022). La radioterapia viene spesso utilizzata dopo la terapia conservativa del seno o la mastectomia (con fattori di rischio) (Hong & Xu, 2022). La chemioterapia sistemica può essere somministrata prima o dopo l'intervento chirurgico, a seconda delle circostanze specifiche (Hong & Xu, 2022). I carcinomi mammari positivi ai recettori ormonali possono essere trattati con farmaci che bloccano gli effetti degli estrogeni e del progesterone. L’immunoterapia è un’opzione terapeutica emergente per alcuni carcinomi mammari, poiché aiuta il sistema immunitario a riconoscere e attaccare le cellule tumorali (Hong & Xu, 2022).
Tecniche di imaging
Mammografia digitale
La mammografia digitale è la tecnica più comunemente utilizzata per lo screening del carcinoma mammario. Si tratta di una tecnica di proiezione bidimensionale in cui i raggi X emessi da un tubo radiogeno vengono assorbiti in varia misura dai tessuti e misurati da un rilevatore situato all'altra estremità. Nelle immagini risultanti, i tessuti più densi appaiono più luminosi rispetto ai tessuti meno densi. Durante l'acquisizione dell'immagine i seni vengono compressi per distribuire il tessuto mammario su una superficie più ampia (Ikeda, 2011a). Ciò riduce la sovrapposizione tra le diverse componenti del tessuto mammario, diminuisce la dispersione dei raggi X in transito e migliora il contrasto. Di solito vengono acquisite due proiezioni di ciascun seno: cranio-caudale (CC) e medio-laterale (MLO) (Ikeda, 2011a).
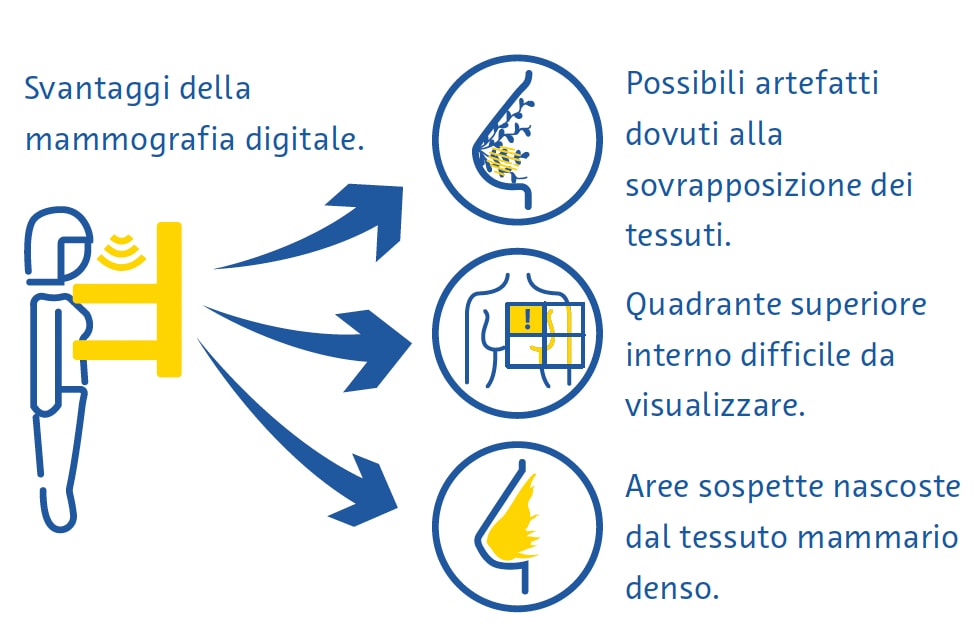
La mammografia digitale è una tecnica veloce e utile per lo screening del carcinoma mammario, ma presenta alcuni inconvenienti (Ikeda, 2011a). La compressione del seno può essere dolorosa e la sovrapposizione di tessuti diversi nonostante la compressione spesso porta ad artefatti (Ikeda, 2011a). Il quadrante superiore interno del seno, che è meno mobile poiché fissato alla parete toracica, è particolarmente difficile da visualizzare alla mammografia (Ikeda, 2011a). Il carcinoma può inoltre essere molto difficile da individuare alla mammografia nel caso dei seni con una grande percentuale di tessuto denso (Ikeda, 2011a).
Tomosintesi mammaria digitale
La tomosintesi mammaria digitale (Digital Breast Tomosynthesis, DBT) prevede l'acquisizione di immagini utilizzando una sorgente di raggi X che si muove lungo un arco di escursione. Le “fette” (sezioni) sottili vengono ricostruite consentendo funzionalità di imaging a 3D destinate a ridurre al minimo l'influenza del tessuto mammario sovrapposto. Questo è particolarmente utile nel caso dell'imaging di lesioni mammarie situate nel parenchima mammario eterogeneo e denso. Uno studio ha rilevato che la DBT è più sensibile per il rilevamento del carcinoma mammario rispetto alla mammografia digitale (Digital Mammography, DM). La DBT può essere combinata con la DM, e uno studio ha mostrato come la combinazione di queste tecniche migliori il rilevamento del carcinoma mammario (Alabousi et al., 2020; Lei et al., 2014; Skaane et al., 2019). È inoltre possibile una combinazione con la mammografia. Tuttavia, la DBT richiede un maggior tempo di acquisizione rispetto alla mammografia e presenta movimento e altri artefatti (Tirada et al., 2019).
Ecografia
Nell'ecografia diagnostica, un trasduttore emette onde sonore ad alta frequenza che attraversano i tessuti, rimbalzando su di essi e creando "echi" che vengono riflessi e rilevati dal trasduttore. Questi echi vengono quindi elaborati per creare immagini in tempo reale su un monitor in base al tempo impiegato dagli echi per raggiungere i tessuti e tornare indietro. Si tratta di una tecnica sicura e relativamente a basso costo che viene spesso utilizzata in aggiunta alla mammografia (Ikeda, 2011b), soprattutto per un’ulteriore valutazione di un reperto rilevato alla palpazione o un reperto mammografico.
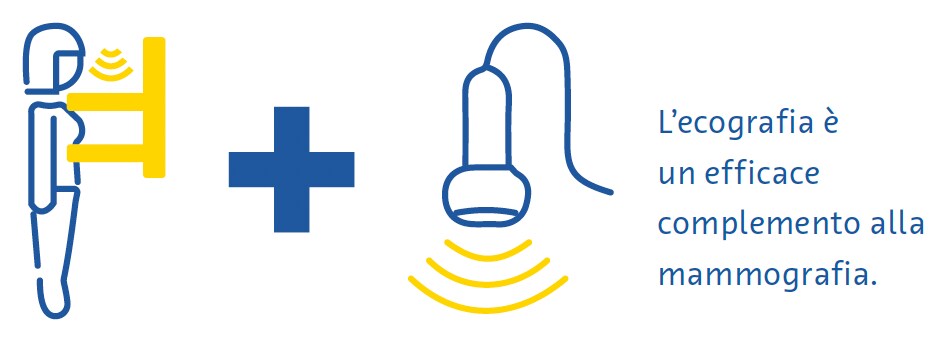
Può anche essere utilizzata come modalità di screening principale nelle donne di età inferiore ai 30 anni o nelle donne in gravidanza o in allattamento (Dixon, 2008; Ikeda, 2011b). L'ecografia è molto utile per chiarire se una massa è cistica o solida e quale tipo di margini e vascolarizzazione presenta (Dixon, 2008; Ikeda, 2011b). Aiuta altresì a rilevare altre masse e linfonodi ascellari sospetti (Dixon, 2008; Ikeda, 2011b). Il suo principale svantaggio è che la qualità dell'esame dipende fortemente dall'operatore (Dixon, 2008; Ikeda, 2011b).
Risonanza magnetica per immagini
La risonanza magnetica per immagini (RMI) utilizza un potente campo magnetico e una serie di onde a radiofrequenza per perturbare i nuclei di idrogeno nei tessuti e creare così immagini dettagliate del corpo in sezione trasversale (Daniel & Ikeda, 2011; Mann et al., 2019). Poiché i tessuti con composizioni diverse rispondono a questa perturbazione in modi diversi, la RMI può rilevare molto bene anche sottili differenze tra i tipi di tessuti molli ed è considerata la modalità più sensibile per la diagnosi del carcinoma mammario (Daniel & Ikeda, 2011; Mann et al., 2019). Viene utilizzata principalmente per lo screening di pazienti ad alto rischio in base a fattori di rischio genetici o acquisiti (Daniel & Ikeda, 2011).
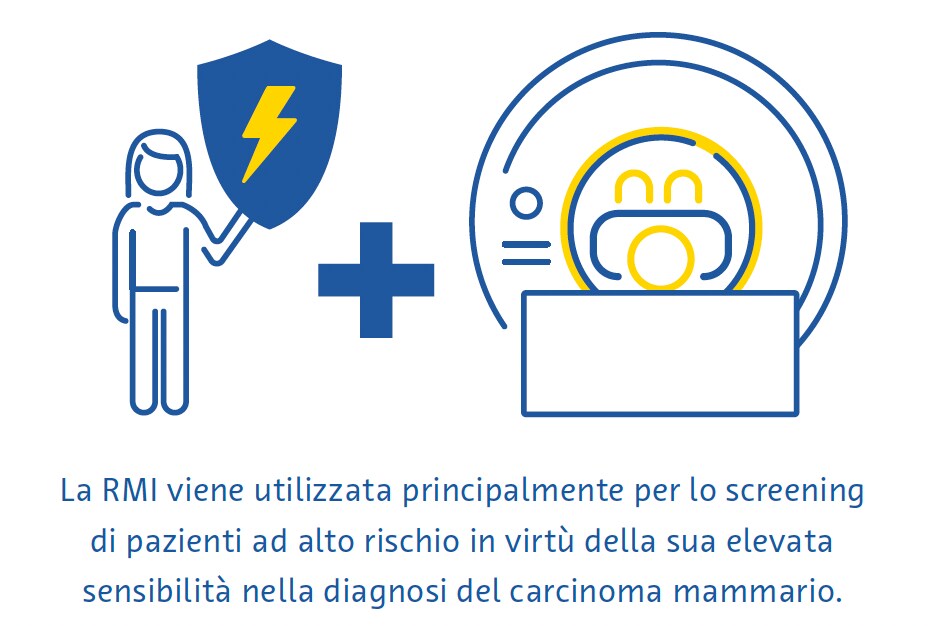
La RMI della mammella richiede bobine mammarie dedicate che trasmettono le onde a radiofrequenza e ricevono il segnale generato. Le immagini vengono spesso acquisite con una risoluzione spaziale nel piano di 1 mm, uno spessore della sezione inferiore a 3 mm e la soppressione del segnale del tessuto adiposo. Le sequenze comunemente utilizzate includono immagini pesate in T2, immagini pesate in diffusione e RMI dinamica con mezzo di contrasto. Per ridurre i falsi positivi dovuti a cambiamenti aspecifici del parenchima mammario, è preferibile eseguire la RMI tra il 7º e il 13º giorno del ciclo mestruale (Daniel & Ikeda, 2011).
A differenza della mammografia, la RMI non prevede l’uso di radiazioni ionizzanti e produce immagini tridimensionali che facilitano il rilevamento di lesioni molto piccole (DeMartini & Lehman, 2008; Shahid et al., 2016). La RMI consente inoltre una valutazione più dettagliata della parete toracica rispetto alla mammografia e all’ecografia (DeMartini & Lehman, 2008). Gli svantaggi della RMI mammaria includono una bassa sensibilità alle microcalcificazioni, il costo elevato e la controindicazione nelle persone con determinati impianti metallici (Daniel & Ikeda, 2011).
Screening e problemi di diagnostica
Nonostante le evidenze a sostegno del beneficio complessivo dello screening del carcinoma mammario (Dibden et al., 2020; Kalager et al., 2010; Tabár et al., 2019), sono diverse le problematiche tecniche e logistiche che si trova ad affrontare. Più della metà delle donne sottoposte a screening annuale per 10 anni presenterà un test falso positivo (Hubbard et al., 2011). Ciò ha conseguenze significative e di ampia portata, tra cui il carico fisico ed emotivo di biopsie non necessarie e l’aumento della spesa sanitaria (Nelson, Pappas, et al., 2016; Ong & Mandl, 2015). Inoltre, spesso lo screening non rileva il carcinoma mammario, in particolare nelle donne con seni densi (Banks et al., 2006).
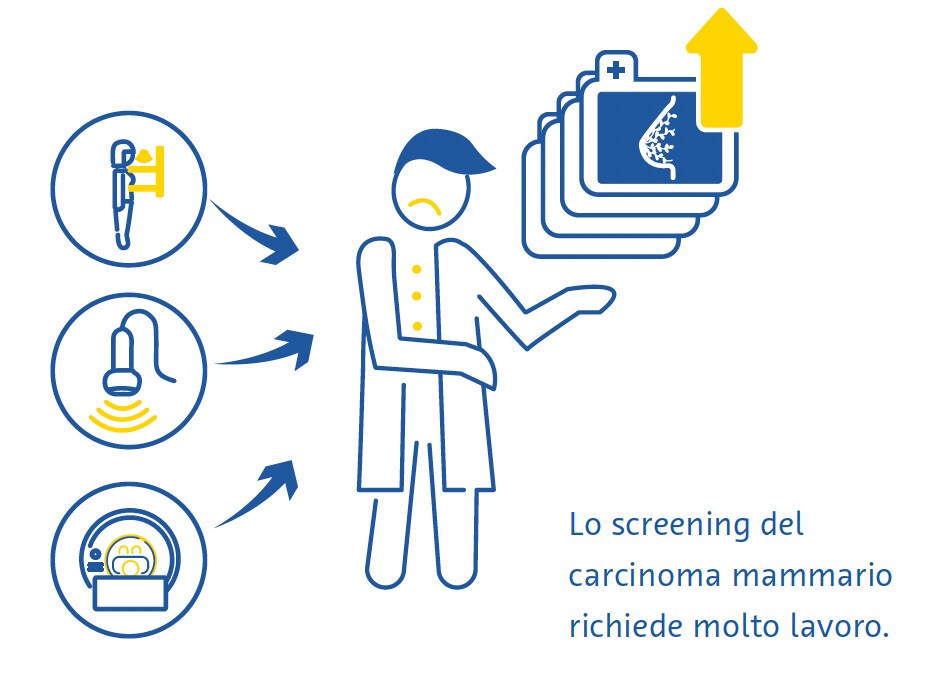
Lo screening del carcinoma mammario richiede operatori altamente qualificati, tra cui radiologi e tecnici di radiologia, di cui attualmente vi è una carenza a livello globale (Moran & Warren-Forward, 2012; Rimmer, 2017; Wing & Langelier, 2009). Questo problema è aggravato dal fatto che lo standard di cura nello screening mammografico in molti Paesi europei prevede che ogni esame venga letto da due radiologi in modo indipendente (Giordano et al., 2012) e dal fatto che, in alcuni Paesi come gli Stati Uniti, gli ostacoli per l’ottenimento della qualifica per l’interpretazione delle mammografie sono elevati a causa dei rigorosi standard di certificazione professionale (Food and Drug Administration, 2001).
Esistono anche notevoli ostacoli alla diffusione dello screening del carcinoma mammario in tutto il mondo. Questi includono la mancanza o la difficoltà di accesso ai programmi di screening, la mancanza di conoscenza o l’incomprensione dei benefici di questi programmi, nonché barriere sociali e culturali (Mascara & Constantinou, 2021).
Il ruolo dell'intelligenza artificiale (IA)
Miglioramenti tecnici
Pochi studi pubblicati hanno studiato finora direttamente l’uso dell’IA per apportare miglioramenti tecnici agli esami senologici. Un’applicazione disponibile in commercio fornisce ai tecnici di radiologia feedback in tempo reale sull’adeguatezza del posizionamento del paziente per le mammografie. (Volpara Health, 2022). Altre applicazioni di IA si sono concentrate sulla riduzione delle dosi di radiazioni (J. Liu et al., 2018), sul miglioramento della ricostruzione delle immagini (Kim et al., 2016) e sulla riduzione del rumore e degli artefatti alla DBT (Garrett et al., 2018).
La DBT è spesso combinata con la mammografia digitale per lo screening del carcinoma mammario, con il conseguente raddoppiamento della dose di radiazioni ricevuta dal paziente (Svahn et al., 2015). Per evitare ciò, è stato rivolto un crescente interesse alla generazione di mammografie sintetiche a partire dai dati della DBT (Chikarmane et al., 2023). In un ampio studio prospettico norvegese, l’accuratezza della DBT combinata con la mammografia digitale o la mammografia sintetica per il rilevamento del carcinoma mammario è risultata molto simile (Skaane et al., 2019). Studi recenti hanno valutato il miglioramento della qualità della mammografia sintetica mediante l’IA con risultati promettenti (Balleyguier et al., 2017; James et al., 2018).
Miglioramenti diagnostici
Valutazione della densità del seno
Il tessuto mammario denso visibile alla mammografia costituisce il tessuto fibroghiandolare. Le donne con seni densi presentano un rischio da 2 a 4 volte maggiore di soffrire di carcinoma mammario rispetto alle donne con seni con tessuto mammario più adiposo (Byrne et al., 1995; Duffy et al., 2018; Torres-Mejía et al., 2005). Inoltre, la sensibilità della mammografia per il carcinoma mammario è inferiore del 20-30% nei seni densi rispetto a quelli meno densi (Lynge et al., 2019). Lo standard di cura nella valutazione della densità del seno utilizza la classificazione BI-RADS (Berg et al., 2000).
Diversi studi di grande respiro hanno esaminato il potenziale della valutazione automatica della densità del seno sulle mammografie utilizzando strumenti basati sull’IA. Una rete neurale convoluzionale (Convolutional Neural Network, CNN) addestrata con 14.000 mammografie e testata su quasi 2.000 mammografie ha classificato la densità del seno come “densità fibroghiandolare sparsa” o “densità eterogenea” con un’area sotto la curva (Area Under the Curve, AUC) di 0,93 (Mohamed et al., 2018). Un altro studio ha utilizzato una CNN in grado di effettuare la classificazione BI-RADS sia a due che a quattro categorie addestrata con oltre 40.000 mammografie (Lehman et al., 2019). In un set di dati di test composto da oltre 8.000 mammografie è stato riscontrato un buon accordo sulla densità del seno tra l’algoritmo e i singoli radiologi (kappa = 0,67), nonché il consenso di cinque radiologi (kappa = 0,78) (Lehman et al., 2019).
Rilevamento del carcinoma mammario
In una revisione sistematica di 82 studi in cui è stata utilizzata l'IA per il rilevamento del carcinoma mammario con vari standard di riferimento, gli autori hanno rilevato un'AUC di 0,87 per gli studi che utilizzavano la mammografia, di 0,91 per gli studi che utilizzavano l’ecografia e per quelli che utilizzavano la DBT e di 0,87 per gli studi che utilizzavano la RMI (Aggarwal et al., 2021). Si tratta di risultati promettenti, tuttavia i confronti diretti tra gli algoritmi basati sull’IA e i radiologi presentano margini di miglioramento. In un'altra revisione sistematica di studi con l'istopatologia o il follow-up (per le donne negative allo screening) come riferimento, il 94% delle 36 CNN identificate sono risultate meno accurate di un singolo radiologo e tutte sono risultate meno accurate del consenso di 2 o più radiologi se utilizzate come sistema indipendente (Freeman et al., 2021). Le evidenze attuali, dunque, non supportano l’uso dell’IA come strategia indipendente per il rilevamento del carcinoma mammario.
Previsione del carcinoma mammario
L’IA si è dimostrata promettente nel predire il rischio di sviluppare il carcinoma mammario sulla base delle mammografie di screening, fornendo una migliore valutazione della densità del seno, che costituisce un fattore di rischio accertato per il carcinoma mammario (Duffy et al., 2018), oppure rilevando sottili caratteristiche dell’imaging che sono foriere di cancro (Batchu et al., 2021). Diversi studi hanno utilizzato modelli basati sull’IA per prevedere il rischio di sviluppare il carcinoma mammario in futuro sulla base delle mammografie (Batchu et al., 2021; Geras et al., 2019).
Una CNN addestrata con quasi 1.000.000 di immagini mammografiche ha mostrato un’AUC di 0,65 per la previsione dello sviluppo futuro del carcinoma mammario rispetto a un valore di 0,57-0,60 per i punteggi di densità del seno basati sulla mammografia convenzionale (Dembrower, Liu, et al., 2020). Uno studio più piccolo ha rilevato un’AUC di 0,73 per un metodo basato su CNN per la previsione del carcinoma mammario sulla base di normali immagini mammografiche. (Arefan et al., 2020). Un altro algoritmo di deep learning ha mostrato un’AUC di 0,82 per la previsione dei cancri intervallo (tumori rilevati entro 12 mesi dopo una mammografia negativa) rispetto a un valore di 0,65 per la valutazione visiva BIRADS della densità del seno (Hinton et al., 2019). Un altro modello basato sul deep learning che incorporava sia fattori di rischio che reperti mammografici per prevedere il rischio di carcinoma mammario presentava un’AUC fino a 0,7, superando l’accuratezza dei modelli predittivi basati solo sui fattori di rischio o sui reperti mammografici. (Yala, Lehman, et al., 2019).
Miglioramenti dell'efficienza
L’enorme volume di esami mammografici e la carenza di radiologi qualificati hanno reso il miglioramento dell’efficienza una delle aree di ricerca più interessanti sull’uso dell’IA nel carcinoma mammario.
In uno studio, gli autori hanno simulato un flusso di lavoro in cui le mammografie venivano interpretate da un radiologo e da un modello di deep learning e la decisione veniva considerata definitiva in caso di concordanza (McKinney et al., 2020). È stato consultato un secondo radiologo solo in caso di disaccordo, con una conseguente riduzione del carico di lavoro dell’88% per il secondo radiologo con un valore predittivo negativo di oltre il 99,9% (McKinney et al., 2020).
In un ampio studio clinico randomizzato, primo nel suo genere, condotto in Svezia, circa 80.000 donne sono state assegnate alla lettura o meno delle loro mammografie di screening da parte di una CNN (Lång et al., 2023). Nel braccio di intervento, sono state sottoposte a doppia lettura solo le mammografie a cui era stato assegnato un punteggio corrispondente a un’elevata probabilità di malignità (il resto è stato letto da un solo radiologo) e i risultati sono stati confrontati con la doppia lettura convenzionale senza l’aiuto dell’algoritmo. In un'analisi ad interim dei dati di 80.000 donne, entrambi i bracci dello studio hanno mostrato un identico tasso di falsi positivi, par all'1,5%. Il valore predittivo positivo del richiamo è stato del 28,3% nel gruppo di intervento e del 24,8% nel gruppo di controllo, e la strategia ha ridotto il carico di lavoro del 44,3% (Lång et al., 2023).
Altri studi hanno utilizzato l’IA per effettuare uno screening preliminare delle mammografie, selezionando quelle con una bassa probabilità di cancro e mostrando a un radiologo solo quelle con un’alta probabilità. Uno studio statunitense ha utilizzato un flusso di lavoro simulato che coinvolgeva una CNN addestrata con oltre 212.000 mammografie e testata su oltre 26.000 a questo scopo (Yala, Schuster, et al., 2019). Il flusso di lavoro che utilizzava l’algoritmo ha mostrato una sensibilità non inferiore per il carcinoma mammario (90,1% vs. 90,6%) e una specificità leggermente maggiore (94,2% vs. 93,5%) rispetto ai radiologi che lavoravano da soli ed è risultato associato a un carico di lavoro inferiore del 19,3% (Yala, Schuster, et al., 2019). Uno studio più piccolo condotto in Spagna ha rilevato una riduzione del carico di lavoro del 72,5% grazie all’utilizzo dell'IA per il triage solo dei casi di DBT ad alto rischio per la lettura da parte di un secondo radiologo e del 29,7% grazie all’utilizzo dell'IA per il triage solo degli studi di DBT ad alto rischio per la lettura da parte di un secondo radiologo, rispetto alla tradizionale doppia lettura dei flussi di lavoro mammografici (Raya-Povedano et al., 2021). È stata inoltre riscontrata una sensibilità non inferiore di questa strategia di utilizzo dell’IA per il triage dei casi di mammografia e DBT ad alto rischio per una seconda lettura rispetto alla doppia lettura standard dei flussi di lavoro mammografici e di DBT (Raya-Povedano et al., 2021). In uno studio svedese, una strategia simile che si avvaleva di un algoritmo di IA disponibile in commercio ha prodotto un tasso di falsi negativi non superiore al 4% e l’algoritmo ha dimostrato di essere in grado di rilevare potenzialmente ulteriori 71 tumori in più ogni 1.000 esami rispetto a una doppia lettura negativa da parte di radiologi umani in pazienti ritenuti ad altissimo rischio dall'algoritmo di IA (Dembrower, Wåhlin, et al., 2020).
In uno studio su oltre un milione di mammografie in otto centri di screening e con dispositivi di tre diversi produttori, un algoritmo di deep learning disponibile in commercio ha determinato nel 63% dei casi che non fossero necessari ulteriori esami sulla base di valutazioni degli esami ad alta affidabilità (Leibig et al., 2022). Il resto degli esami, per i quali la sicurezza dell'algoritmo era bassa, sono stati mostrati ai radiologi. Questa strategia ha migliorato la sensibilità dei radiologi (rispetto alla lettura senza ausilio) del 2,6-4% e la specificità dello 0,5-1,0% (Leibig et al., 2022).
Problemi e orientamenti futuri
Diverse problematiche etiche, tecniche e metodologiche associate all’uso dell’IA nello screening del carcinoma mammario forniscono un quadro per guidare la ricerca futura in questo campo (Hickman et al., 2021).
La maggior parte degli strumenti basati sull'IA si sono finora concentrati sulla mammografia digitale (Aggarwal et al., 2021), ma altre tecniche di esame come la DBT e la RMI presentano vantaggi unici (Alsheik et al., 2019; Mann et al., 2019) e probabilmente ricopriranno in futuro un ruolo più importante nello screening del carcinoma mammario. Tuttavia, poiché la DBT e la RMI sono tecniche tomografiche che producono immagini tridimensionali, la loro elaborazione mediante strumenti basati sull’IA richiederà uno spazio di archiviazione e una potenza di calcolo maggiori (Prevedello et al., 2019).
L’incidenza, la presentazione e l’esito del carcinoma mammario sono correlati a diversi fattori sociodemografici, tra cui razza ed etnia (Hirko et al., 2022; Hu et al., 2019; Martini et al., 2022). L’addestramento di strumenti basati sull’IA con set di dati rappresentativi di una popolazione diversificata è fondamentale per garantire che possano essere generalizzati e arrecare benefici al maggior numero di persone possibile.
Le prestazioni complessive dell’IA nel rilevamento del carcinoma mammario sono state ragguardevoli. Tuttavia, è interessante notare che in uno studio non è stato possibile dimostrare la non inferiorità della sensibilità dell’IA nel rilevamento del carcinoma mammario rispetto a quella dei radiologi (Lauritzen et al., 2022). Inoltre, si nutrono preoccupazioni circa la qualità delle evidenze alla base di molti studi su questo argomento. Una revisione sistematica che ha studiato l’accuratezza degli strumenti basati sull’IA nel rilevamento del carcinoma mammario ha identificato diverse aree di potenziale miglioramento (Freeman et al., 2021). La revisione non ha individuato studi prospettici e gli studi identificati erano di scarsa qualità metodologica. In particolare, gli autorihanno osservato che gli studi più piccoli hanno mostrato risultati più positivi che non sono stati riprodotti in studi di più ampio respiro. In un’altra revisione sistematica, solo circa un decimo degli studi aveva utilizzato un set di dati esterno per la convalida, nessuno studio aveva fornito un calcolo prespecificato delle dimensioni del campione e sono stati identificati seri problemi con bias di selezione e standard di riferimento inappropriati (Aggarwal et al., 2021). Questi problemi metodologici possono potenzialmente essere mitigati in futuro con l’introduzione di grandi archivi di dati aperti (Nguyen et al., 2023) e una maggiore aderenza alle linee guida per la conduzione di ricerche mediche basate sull'IA (Lekadir et al., 2021; X. Liu et al., 2020).
Conclusioni
L’integrazione dell’intelligenza artificiale nei programmi di screening del carcinoma mammario è promettente nel migliorare la qualità delle immagini e l'efficienza e nel prevedere il rischio futuro di carcinoma mammario. Per il rilevamento del carcinoma mammario negli esami di screening, le evidenze disponibili suggeriscono che l’intelligenza artificiale presenta risultati migliori quando viene usata in sinergia con i radiologi. Sono fondamentali ricerche continue per affrontare le problematiche associate all'uso dell'IA nello screening del carcinoma mammario, tra cui l'espansione del suo campo di applicazione oltre la mammografia e la garanzia di un uso etico e responsabile. Con la continua evoluzione delle applicazioni di IA, il futuro dello screening del carcinoma mammario racchiude un immenso potenziale per una maggiore accessibilità, un intervento precoce e, in definitiva, migliori risultati per i/le pazienti.
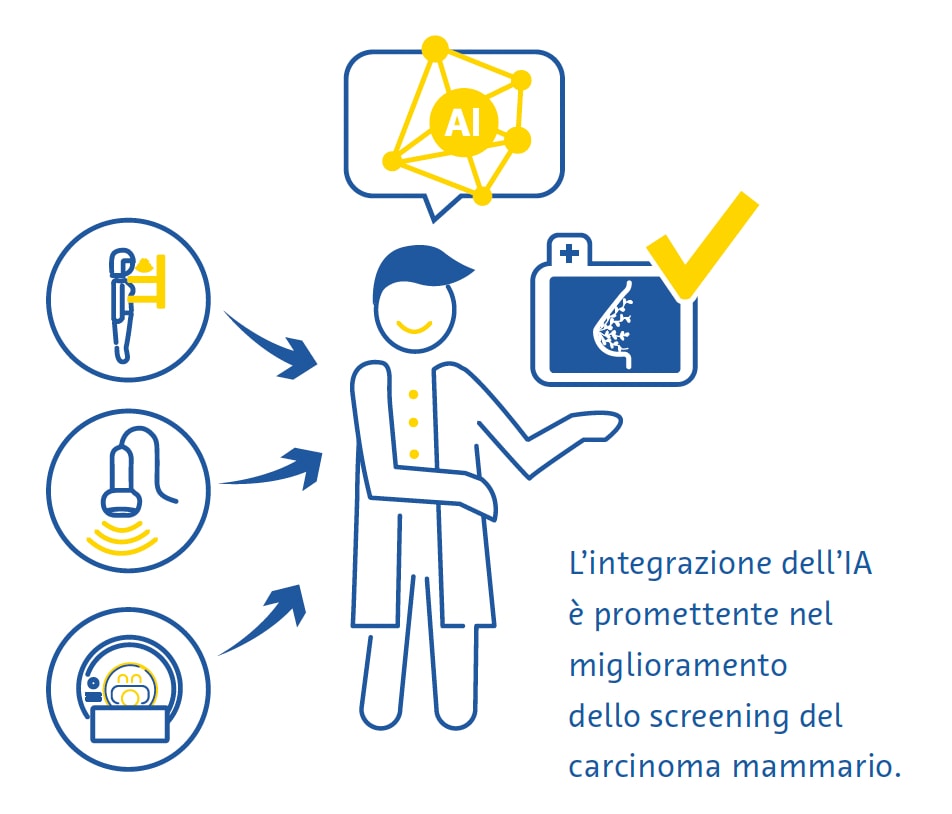
Bibliografia
Aggarwal, R., Sounderajah, V., Martin, G., Ting, D. S. W., Karthikesalingam, A., King, D., Ashrafian, H., & Darzi, A. (2021). Diagnostic accuracy of deep learning in medical imaging: a systematic review and meta-analysis. NPJ Digital Medicine, 4(1), 65.
Alabousi, M., Zha, N., Salameh, J.-P., Samoilov, L., Sharifabadi, A. D., Pozdnyakov, A., Sadeghirad, B., Freitas, V., McInnes, M. D. F., & Alabousi, A. (2020). Digital breast tomosynthesis for breast cancer detection: a diagnostic test accuracy systematic review and meta-analysis. European Radiology, 30(4), 2058–2071.
Alkabban FM and Ferguson T. (2022). Breast Cancer, 2022. In: StatPearls [Internet]. Treasure Island (FL): StatPearls Publishing.
Alsheik, N. H., Dabbous, F., Pohlman, S. K., Troeger, K. M., Gliklich, R. E., Donadio, G. M., Su, Z., Menon, V., & Conant, E. F. (2019). Comparison of Resource Utilization and Clinical Outcomes Following Screening with Digital Breast Tomosynthesis Versus Digital Mammography: Findings From a Learning Health System. Academic Radiology, 26(5), 597–605.
Arefan, D., Mohamed, A. A., Berg, W. A., Zuley, M. L., Sumkin, J. H., & Wu, S. (2020). Deep learning modeling using normal mammograms for predicting breast cancer risk. Medical Physics, 47(1), 110–118.
Balleyguier, C., Arfi-Rouche, J., Levy, L., Toubiana, P. R., Cohen-Scali, F., Toledano, A. Y., & Boyer, B. (2017). Improving digital breast tomosynthesis reading time: A pilot multi-reader, multi-case study using concurrent Computer-Aided Detection (CAD). European Journal of Radiology, 97, 83–89.
Banks, E., Reeves, G., Beral, V., Bull, D., Crossley, B., Simmonds, M., Hilton, E., Bailey, S., Barrett, N., Briers, P., English, R., Jackson, A., Kutt, E., Lavelle, J., Rockall, L., Wallis, M. G., Wilson, M., & Patnick, J. (2006). Hormone replacement therapy and false positive recall in the Million Women Study: patterns of use, hormonal constituents and consistency of effect. Breast Cancer Research: BCR, 8(1), R8.
Batchu, S., Liu, F., Amireh, A., Waller, J., & Umair, M. (2021). A Review of Applications of Machine Learning in Mammography and Future Challenges. Oncology, 99(8), 483–490. https://doi.org/10.1159/000515698
Bazira, P. J., Ellis, H., & Mahadevan, V. (2022). Anatomy and physiology of the breast. Surgery, 40(2), 79–83. https://doi.org/10.1016/j.mpsur.2021.11.015
Berg, W. A., Campassi, C., Langenberg, P., & Sexton, M. J. (2000). Breast Imaging Reporting and Data System: interand intraobserver variability in feature analysis and final assessment. AJR. American Journal of Roentgenology, 174(6), 1769–1777.
Boyd, N. F., Guo, H., Martin, L. J., Sun, L., Stone, J., Fishell, E., Jong, R. A., Hislop, G., Chiarelli, A., Minkin, S., & Yaffe, M. J. (2007). Mammographic density and the risk and detection of breast cancer. The New England Journal of Medicine, 356(3), 227–236.
Bray, F., Ferlay, J., Soerjomataram, I., Siegel, R. L., Torre, L. A., & Jemal, A. (2018). Global cancer statistics 2018: GLOBOCAN estimates of incidence and mortality worldwide for 36 cancers in 185 countries. CA: A Cancer Journal for Clinicians, 68(6), 394–424.
Breast Cancer Statistics. (2020, October 27). Susan G. Komen®. https://www.komen.org/breast-cancer/facts-statistics/breastcancer- statistics/
Byrne, C., Schairer, C., Wolfe, J., Parekh, N., Salane, M., Brinton, L. A., Hoover, R., & Haile, R. (1995). Mammographic features and breast cancer risk: effects with time, age, and menopause status. Journal of the National Cancer Institute, 87(21), 1622–1629.
Chikarmane, S. A., Offit, L. R., & Giess, C. S. (2023). Synthetic Mammography: Benefits, Drawbacks, and Pitfalls. Radiographics: A Review Publication of the Radiological Society of North America, Inc, 43(10), e230018. https://doi.org/10.1148/rg.230018
Daniel, B. L., & Ikeda, D. M. (2011). Chapter 7 - Magnetic Resonance Imaging of Breast Cancer and MRI-Guided Breast Biopsy. In D. M. Ikeda (Ed.), Breast Imaging (Second Edition) (pp. 239–296). Mosby.
DeMartini W & Lehman C, (2008). Top Magn Reson Imaging, Jun;19(3):143-50.
Dembrower, K., Liu, Y., Azizpour, H., Eklund, M., Smith, K., Lindholm, P., & Strand, F. (2020). Comparison of a Deep Learning Risk Score and Standard Mammographic Density Score for Breast Cancer Risk Prediction. Radiology, 294(2), 265–272.
Dembrower, K., Wåhlin, E., Liu, Y., Salim, M., Smith, K., Lindholm, P., Eklund, M., & Strand, F. (2020). Effect of artificial intelligence-based triaging of breast cancer screening mammograms on cancer detection and radiologist workload: a retrospective simulation study. The Lancet. Digital Health, 2(9), e468–e474.
Dibden, A., Offman, J., Duffy, S. W., & Gabe, R. (2020). Worldwide Review and Meta-Analysis of Cohort Studies Measuring the Effect of Mammography Screening Programmes on Incidence-Based Breast Cancer Mortality. Cancers, 12(4). https://doi.org/10.3390/cancers12040976
Duffy, S. W., Morrish, O. W. E., Allgood, P. C., Black, R., Gillan, M. G. C., Willsher, P., Cooke, J., Duncan, K. A., Michell, M. J., Dobson, H. M., Maroni, R., Lim, Y. Y., Purushothaman, H. N., Suaris, T., Astley, S. M., Young, K. C., Tucker, L., & Gilbert, F. J. (2018). Mammographic density and breast cancer risk in breast screening assessment cases and women with a family history of breast cancer. European Journal of Cancer, 88, 48–56.
Food and Drug Administration. (2001). The Mammography Quality Standards Act Final Regulations: Preparing for MQSA Inspections; Final Guidance for Industry and FDA.
Freeman, K., Geppert, J., Stinton, C., Todkill, D., Johnson, S., Clarke, A., & Taylor-Phillips, S. (2021). Use of artificial intelligence for image analysis in breast cancer screening programmes: systematic review of test accuracy. BMJ, 374, n1872.
Freer, P. E. (2015). Mammographic breast density: impact on breast cancer risk and implications for screening. Radiographics: A Review Publication of the Radiological Society of North America, Inc, 35(2), 302–315.
Garrett, J. W., Li, Y., Li, K., & Chen, G.-H. (2018). Reduced anatomical clutter in digital breast tomosynthesis with statistical iterative reconstruction. Medical Physics, 45(5), 2009–2022.
Geras, K. J., Mann, R. M., & Moy, L. (2019). Artificial Intelligence for Mammography and Digital Breast Tomosynthesis: Current Concepts and Future Perspectives. Radiology, 293(2), 246–259. https://doi.org/10.1148/radiol.2019182627
Giordano, L., von Karsa, L., Tomatis, M., Majek, O., de Wolf, C., Lancucki, L., Hofvind, S., Nyström, L., Segnan, N., Ponti, A., Eunice Working Group, Van Hal, G., Martens, P., Májek, O., Danes, J., von Euler-Chelpin, M., Aasmaa, A., Anttila, A., Becker, N., … Suonio, E. (2012). Mammographic screening programmes in Europe: organization, coverage and participation. Journal of Medical Screening, 19 Suppl 1, 72–82.
Harbeck, N., Penault-Llorca, F., Cortes, J., Gnant, M., Houssami, N., Poortmans, P., Ruddy, K., Tsang, J., & Cardoso, F. (2019). Breast cancer. Nature Reviews. Disease Primers, 5(1), 66. https://doi.org/10.1038/s41572-019-0111-2
Hickman, S. E., Baxter, G. C., & Gilbert, F. J. (2021). Adoption of artificial intelligence in breast imaging: evaluation, ethical constraints and limitations. British Journal of Cancer, 125(1), 15–22.
Hinton, B., Ma, L., Mahmoudzadeh, A. P., Malkov, S., Fan, B., Greenwood, H., Joe, B., Lee, V., Kerlikowske, K., & Shepherd, J. (2019). Deep learning networks find unique mammographic differences in previous negative mammograms between interval and screen-detected cancers: a case-case study. Cancer Imaging: The Official Publication of the International Cancer Imaging Society, 19(1), 41.
Hirko, K. A., Rocque, G., Reasor, E., Taye, A., Daly, A., Cutress, R. I., Copson, E. R., Lee, D.-W., Lee, K.-H., Im, S.-A., & Park, Y. H. (2022). The impact of race and ethnicity in breast cancer-disparities and implications for precision oncology.BMC Medicine, 20(1), 72.
Hong, R., & Xu, B. (2022). Breast cancer: an up-to-date review and future perspectives. Cancer Communications, 42(10), 913–936. https://doi.org/10.1002/cac2.12358
Hubbard, R. A., Kerlikowske, K., Flowers, C. I., Yankaskas, B. C., Zhu, W., & Miglioretti, D. L. (2011). Cumulative probability of false-positive recall or biopsy recommendation after 10 years of screening mammography: a cohort study.Annals of Internal Medicine, 155(8), 481–492.
Hu, K., Ding, P., Wu, Y., Tian, W., Pan, T., & Zhang, S. (2019). Global patterns and trends in the breast cancer incidence and mortality according to sociodemographic indices: an observational study based on the global burden of diseases. BMJ Open, 9(10), e028461.
Ikeda, D. M. (Ed.). (2011a). Chapter 2 - Mammogram Interpretation. In Breast Imaging (Second Edition) (pp. 24–62). Mosby.
Ikeda, D. M. (Ed.). (2011b). Chapter 5 - Breast Ultrasound. In Breast Imaging (Second Edition) (pp. 149–193). Mosby.
James, J. J., Giannotti, E., & Chen, Y. (2018). Evaluation of a computer-aided detection (CAD)-enhanced 2D synthetic mammogram: comparison with standard synthetic 2D mammograms and conventional 2D digital mammography. Clinical Radiology, 73(10), 886–892.
Kalager, M., Zelen, M., Langmark, F., & Adami, H.-O. (2010). Effect of screening mammography on breast-cancer mortality in Norway. The New England Journal of Medicine, 363(13), 1203–1210. https://doi.org/10.1056/NEJMoa1000727
Kim, Y.-S., Park, H.-S., Lee, H.-H., Choi, Y.-W., Choi, J.-G., Kim, H. H., & Kim, H.-J. (2016). Comparison study of reconstruction algorithms for prototype digital breast tomosynthesis using various breast phantoms. La Radiologia Medica, 121(2), 81–92.
Koo, M. M., von Wagner, C., Abel, G. A., McPhail, S., Rubin, G. P., & Lyratzopoulos, G. (2017). Typical and atypical presenting symptoms of breast cancer and their associations with diagnostic intervals: Evidence from a national audit of cancer diagnosis. Cancer Epidemiology, 48, 140–146. https://doi.org/10.1016/j.canep.2017.04.010
Lång, K., Josefsson, V., Larsson, A.-M., Larsson, S., Högberg, C., Sartor, H., Hofvind, S., Andersson, I., & Rosso, A. (2023). Artificial intelligence-supported screen reading versus standard double reading in the Mammography Screening with Artificial Intelligence trial (MASAI): a clinical safety analysis of a randomised, controlled, non-inferiority, single-blinded, screening accuracy study. The Lancet Oncology, 24(8), 936–944.
Lauritzen, A. D., Rodríguez-Ruiz, A., von Euler-Chelpin, M. C., Lynge, E., Vejborg, I., Nielsen, M., Karssemeijer, N., & Lillholm, M. (2022). An Artificial Intelligence-based Mammography Screening Protocol for Breast Cancer: Outcome and Radiologist Workload.Radiology, 304(1), 41–49.
Lehman, C. D., Yala, A., Schuster, T., Dontchos, B., Bahl, M., Swanson, K., & Barzilay, R. (2019). Mammographic Breast Density Assessment Using Deep Learning: Clinical Implementation. Radiology, 290(1), 52–58.
Leibig, C., Brehmer, M., Bunk, S., Byng, D., Pinker, K., & Umutlu, L. (2022). Combining the strengths of radiologists and AI for breast cancer screening: a retrospective analysis. The Lancet. Digital Health, 4(7), e507–e519.
Lei, J., Yang, P., Zhang, L., Wang, Y., & Yang, K. (2014). Diagnostic accuracy of digital breast tomosynthesis versus digital mammography for benign and malignant lesions in breasts: a meta-analysis. European Radiology, 24(3), 595–602.
Lekadir, K., Osuala, R., Gallin, C., Lazrak, N., Kushibar, K., Tsakou, G., Aussó, S., Alberich, L. C., Marias, K., Tsiknakis, M., Colantonio, S., Papanikolaou, N., Salahuddin, Z., Woodruff, H. C., Lambin, P., & Martí-Bonmatí, L. (2021). FUTURE-AI: Guiding Principles and Consensus Recommendations for Trustworthy Artificial Intelligence in Medical Imaging. In arXiv [cs.CV].arXiv. https://arxiv.org/abs/2109.09658
Liu, J., Zarshenas, A., Qadir, A., Wei, Z., Yang, L., Fajardo, L., & Suzuki, K. (2018). Radiation dose reduction in digital breast tomosynthesis (DBT) by means of deep-learning-based supervised image processing. Medical Imaging 2018: Image Processing, 10574, 89–97.
Liu, X., Cruz Rivera, S., Moher, D., Calvert, M. J., Denniston, A. K., & SPIRIT-AI and CONSORT-AI Working Group. (2020). Reporting guidelines for clinical trial reports for interventions involving artificial intelligence: the CONSORT-AI extension. Nature Medicine, 26(9), 1364–1374.
Łukasiewicz, S., Czeczelewski, M., Forma, A., Baj, J., Sitarz, R., & Stanisławek, A. (2021). Breast Cancer-Epidemiology, Risk Factors, Classification, Prognostic Markers, and Current Treatment Strategies-An Updated Review. Cancers, 13(17). https://doi.org/10.3390/cancers13174287
Lynge, E., Vejborg, I., Andersen, Z., von Euler-Chelpin, M., & Napolitano, G. (2019). Mammographic Density and Screening Sensitivity, Breast Cancer Incidence and Associated Risk Factors in Danish Breast Cancer Screening. Journal of Clinical Medicine Research, 8(11). https://doi.org/10.3390/jcm8112021
Mann, R. M., Cho, N., & Moy, L. (2019). Breast MRI: State of the Art. Radiology, 292(3), 520–536.
Martini, R., Newman, L., & Davis, M. (2022). Breast cancer disparities in outcomes; unmasking biological determinants associated with racial and genetic diversity. Clinical & Experimental Metastasis, 39(1), 7–14.
Mascara, M., & Constantinou, C. (2021). Global Perceptions of Women on Breast Cancer and Barriers to Screening. Current Oncology Reports, 23(7), 74.
McDonald, E. S., Clark, A. S., Tchou, J., Zhang, P., & Freedman, G. M. (2016). Clinical Diagnosis and Management of Breast Cancer. Journal of Nuclear Medicine: Official Publication, Society of Nuclear Medicine, 57 Suppl 1, 9S – 16S. https://doi.org/10.2967/jnumed.115.157834
McKinney, S. M., Sieniek, M., Godbole, V., Godwin, J., Antropova, N., Ashrafian, H., Back, T., Chesus, M., Corrado, G. S., Darzi, A., Etemadi, M., Garcia-Vicente, F., Gilbert, F. J., Halling-Brown, M., Hassabis, D., Jansen, S., Karthikesalingam, A., Kelly, C. J., King, D., … Shetty, S. (2020). International evaluation of an AI system for breast cancer screening. Nature, 577(7788), 89–94.
Mohamed, A. A., Berg, W. A., Peng, H., Luo, Y., Jankowitz, R. C., & Wu, S. (2018). A deep learning method for classifying mammographic breast density categories. Medical Physics, 45(1), 314–321.
Moran, S., & Warren-Forward, H. (2012). The Australian BreastScreen workforce: a snapshot. The Radiographer, 59(1), 26–30.
Nelson, H. D., Fu, R., Cantor, A., Pappas, M., Daeges, M., & Humphrey, L. (2016). Effectiveness of Breast Cancer Screening: Systematic Review and Meta-analysis to Update the 2009 U.S. Preventive Services Task Force Recommendation. Annals of Internal Medicine, 164(4), 244–255.
Nelson, H. D., Pappas, M., Cantor, A., Griffin, J., Daeges, M., & Humphrey, L. (2016). Harms of Breast Cancer Screening: Systematic Review to Update the 2009 U.S. Preventive Services Task Force Recommendation. Annals of Internal Medicine, 164(4), 256–267.
Nguyen, H. T., Nguyen, H. Q., Pham, H. H., Lam, K., Le, L. T., Dao, M., & Vu, V. (2023). VinDr-Mammo: A large-scale benchmark dataset for computer-aided diagnosis in full-field digital mammography. Scientific Data, 10(1), 277.
Ong, M.-S., & Mandl, K. D. (2015). National expenditure for false-positive mammograms and breast cancer overdiagnoses estimated at $4 billion a year. Health Affairs , 34(4), 576–583.
Prevedello, L. M., Halabi, S. S., Shih, G., Wu, C. C., Kohli, M. D., Chokshi, F. H., Erickson, B. J., Kalpathy-Cramer, J., Andriole, K. P., & Flanders, A. E. (2019). Challenges Related to Artificial Intelligence Research in Medical Imaging and the Importance of Image Analysis Competitions. Radiology. Artificial Intelligence, 1(1), e180031. https://doi.org/10.1148/ ryai.2019180031
Raya-Povedano, J. L., Romero-Martín, S., Elías-Cabot, E., Gubern-Mérida, A., Rodríguez-Ruiz, A., & Álvarez-Benito, M. (2021). AI-based Strategies to Reduce Workload in Breast Cancer Screening with Mammography and Tomosynthesis: A Retrospective Evaluation. Radiology, 300(1), 57–65.
Rimmer, A. (2017). Radiologist shortage leaves patient care at risk, warns royal college. BMJ, 359, j4683.
Skaane, P., Bandos, A. I., Niklason, L. T., Sebuødegård, S., Østerås, B. H., Gullien, R., Gur, D., & Hofvind, S. (2019). Digital Mammography versus Digital Mammography Plus Tomosynthesis in Breast Cancer Screening: The Oslo Tomosynthesis Screening Trial. Radiology, 291(1), 23–30.
Sung H et al,(2021). CA Cancer J Clin, 2021 May;71(3):209-249. doi: 10.3322/caac.21660
Tabár, L., Dean, P. B., Chen, T. H.-H., Yen, A. M.-F., Chen, S. L.- S., Fann, J. C.-Y., Chiu, S. Y.-H., Ku, M. M.-S., Wu, W. Y.-Y., Hsu, C.-Y., Chen, Y.-C., Beckmann, K., Smith, R. A., & Duffy, S. W. (2019). The incidence of fatal breast cancer measures the increased effectiveness of therapy in women participating in mammography screening. Cancer, 125(4), 515–523.
Svahn, T. M., Houssami, N., Sechopoulos, I., & Mattsson, S. (2015). Review of radiation dose estimates in digital breast tomosynthesis relative to those in two-view full-field digital mammography. Breast, 24(2), 93–99. https://doi.org/10.1016/j. breast.2014.12.002
Tirada, N., Li, G., Dreizin, D., Robinson, L., Khorjekar, G., Dromi, S., & Ernst, T. (2019). Digital Breast Tomosynthesis: Physics, Artifacts, and Quality Control Considerations. Radiographics: A Review Publication of the Radiological Society of North America, Inc, 39(2), 413–426.
Torres-Mejía, G., De Stavola, B., Allen, D. S., Pérez-Gavilán, J. J., Ferreira, J. M., Fentiman, I. S., & Dos Santos Silva, I. (2005). Mammographic features and subsequent risk of breast cancer: a comparison of qualitative and quantitative evaluations in the Guernsey prospective studies. Cancer Epidemiology, Biomarkers & Prevention: A Publication of the American Association for Cancer Research, Cosponsored by the American Society of Preventive Oncology, 14(5), 1052–1059.
Volpara Health. (2022). TruPGMI: AI for mammography quality improvement. https://www.volparahealth.com/breast-healthsoftware/ products/analytics/
Wing, P., & Langelier, M. H. (2009). Workforce shortages in breast imaging: impact on mammography utilization. AJR. American Journal of Roentgenology, 192(2), 370–378.
Yala, A., Lehman, C., Schuster, T., Portnoi, T., & Barzilay, R. (2019). A Deep Learning Mammography-based Model for Improved Breast Cancer Risk Prediction. Radiology, 292(1), 60–66.
Yala, A., Schuster, T., Miles, R., Barzilay, R., & Lehman, C. (2019). A Deep Learning Model to Triage Screening Mammograms: A Simulation Study. Radiology, 293(1), 38–46.