L’intelligenza artificiale (IA) è un campo che consente ai sistemi informatici di risolvere problemi adattandosi alle circostanze mutevoli, spesso imitando il ragionamento e il giudizio umano. L’uso dell’IA nell’imaging medico è attualmente orientato a varie tendenze demografiche e sanitarie. La quantità di dati di imaging medico che vengono acquisiti è in costante aumento (Larson et al., 2011; Smith- Bindman et al., 2008, 2012; Winder et al., 2021). Vi è inoltre una diffusa carenza di operatori sanitari (Core Health Indicators in the WHO European Region 2015. Special Focus: Human Resources for Health, 2017) con un carico di lavoro sempre crescente (Levin et al., 2017), e si prevede che il numero di esami di imaging medico crescerà esponenzialmente nei prossimi due decenni (Tsao, 2020). Mancano, in particolare, medici radiologi e tecnici di radiologia (AAMC Report Reinforces Mounting Physician Shortage, 2021, Clinical Radiology UK Workforce Census 2019 Report, 2019). Infine, si prevede che nel prossimo futuro questi problemi saranno aggravati dall’invecchiamento della popolazione mondiale (Population Ages 65 and above, senza data; OMS, senza data-a) e dal crescente carico globale di malattie croniche (OMS, senza data-b).
In generale, il vantaggio dell’intelligenza artificiale nell’imaging medico potrebbe consistere nella possibilità di ottenere informazioni che non sarebbe possibile ottenere con i metodi tradizionali (come con la semplice osservazione delle immagini da parte di un essere umano) e di averle più rapidamente e in modo più automatizzato (senza bisogno dell’intervento umano). Le soluzioni basate sull’IA nell’imaging medico potrebbero migliorare e accelerare l’identificazione delle patologie, generare una valutazione approfondita del rischio di sviluppo e progressione di una malattia e ridurre la soggettività nell’interpretazione dei dati di imaging medico.
Lo stato attuale dell’intelligenza artificiale nell’imaging medico
Negli ultimi anni il panorama dell’IA nell’imaging medico è cambiato radicalmente. Sono emerse molte applicazioni promettenti; il campo ha visto un aumento senza precedenti di stanziamenti di denaro e abbiamo assistito a tendenze positive nell’adozione di soluzioni di IA da parte dei radiologi, nonché nella loro approvazione da parte degli organismi normativi.
Applicazioni
Sebbene i reparti di radiologia forniscano una vasta gamma di servizi, il servizio principale è indubbiamente l’esame di imaging. È dunque possibile suddividere le applicazioni di IA in quelle da applicare prima, durante o dopo l’esame di imaging.
Prima dell'acquisizione delle immagini
Nel contesto del flusso di lavoro di un reparto di radiologia, vi sono alcuni passaggi da svolgere prima che un paziente venga sottoposto a un esame di imaging. Le applicazioni di IA che mirano a migliorare questi passaggi sono chiamate “IA a monte” (upstream AI) e potrebbero aumentarne l’efficienza rendendo più personalizzato il processo decisionale in un reparto di radiologia.
La mancata presentazione del paziente all’appuntamento per l’esame è piuttosto comune e l’efficienza degli ospedali, oltre a comportare uno spreco di risorse (Dantas et al., 2018). Studi condotti in Giappone (Kurasawa et al., 2016) e nel Regno Unito (Nelson et al., 2019) hanno dimostrato che l’IA può essere utilizzata per prevedere questa problematica con elevata accuratezza, il che consentirebbe di mettere in atto strategie volte a ridurre la probabilità che un paziente non si presenti all’esame, ad esempio inviando promemoria automatici.
Una delle decisioni più importanti che viene presa nei reparti di radiologia è l'esatto protocollo di scansione da utilizzare per un determinato paziente. Pur valendo per tutte le modalità di imaging, la gamma più ampia di scelta si ha con la risonanza magnetica (RM), per la quale si può scegliere la serie di sequenze più appropriata e si deve decidere se sia opportuno o meno somministrare mezzi di contrasto per via endovenosa. Per selezionare adeguati protocolli di RM sono stati utilizzati classificatori del linguaggio naturale che interpretano il testo delle richieste di scansione del medico. In uno studio, un classificatore di aumento del gradiente ha previsto il protocollo giusto da utilizzare in una RM cerebrale in base alla richiesta dell’esame con elevata accuratezza (95%) (Brown & Marotta, 2018). Per la RM muscoloscheletrica, un classificatore basato sul deep learning (apprendimento profondo) ha mostrato un’accuratezza dell'83% nel determinare la necessità di utilizzo di un mezzo di contrasto (Trivedi et al., 2018). Tali applicazioni possono migliorare sostanzialmente l’efficienza eliminando per il radiologo la gravosa (in termini di tempo) attività di comprensione dei testi non strutturati delle richieste di esami scritte dai medici richiedenti.
Durante l’acquisizione delle immagini
Recentemente sono stati apportati miglioramenti sostanziali nell’uso dell’IA per migliorare la qualità delle immagini. In un recente sondaggio, dei radiologi hanno indicato proprio il miglioramento della qualità dell’immagine come l’esigenza principale per cui l’IA potrebbe rivelarsi utile nell’imaging medico (Alexander et al., 2020). Anche se i primi tentativi di ridurre il rumore nelle immagini mediante tecniche di deep learning sono stati criticati perché avevano rimosso alcuni dettagli, mettendo a repentaglio la visibilità delle caratteristiche essenziali dell’immagine stessa, le implementazioni più recenti hanno per lo più superato questo problema.
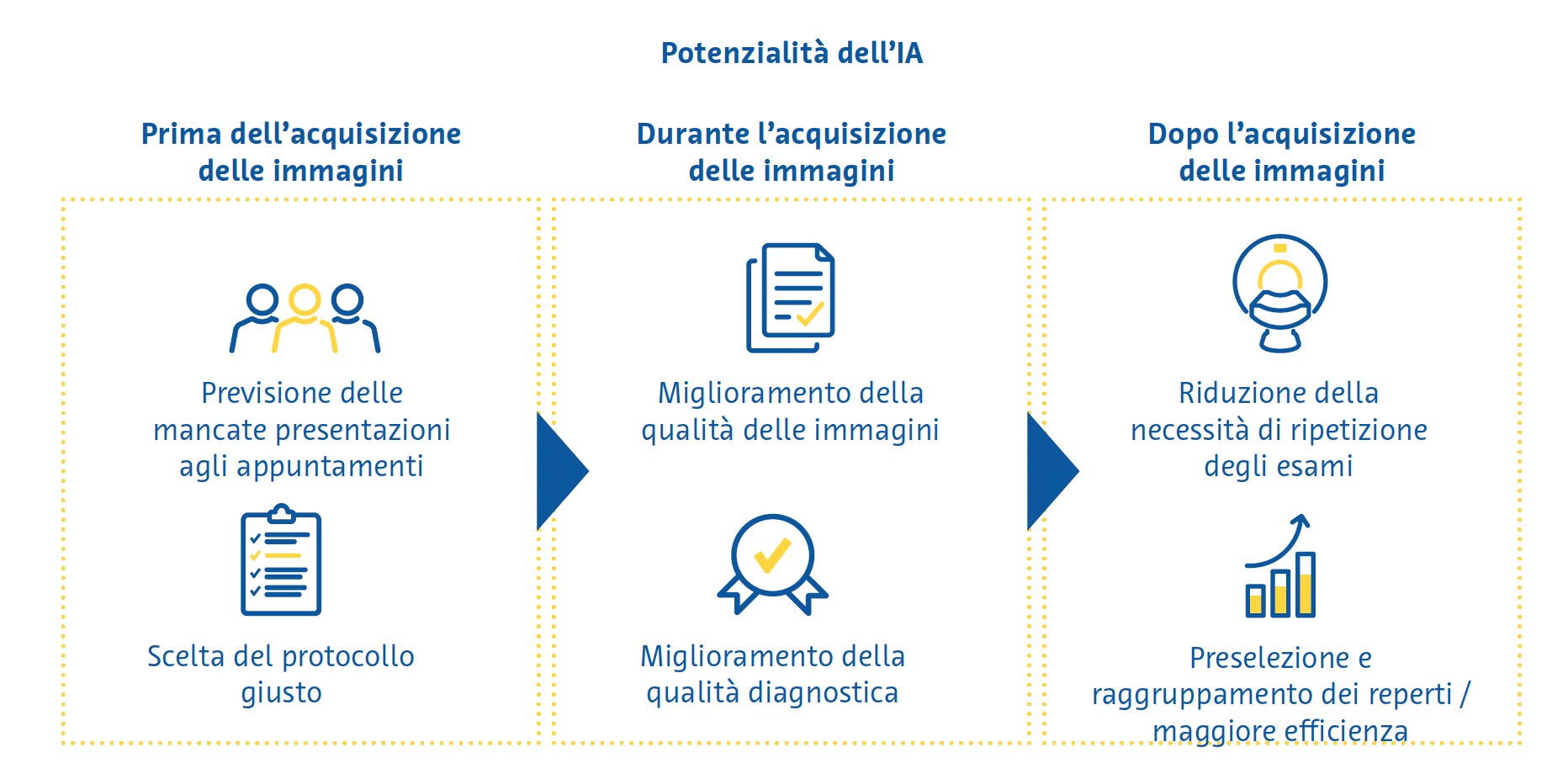
In particolare, tecniche di deep learning come le reti generative avversarie hanno mostrato di avere grandi potenzialità nel ridurre il rumore nelle immagini (Wang et al., 2021). Alcune di queste applicazioni agiscono sulla fase di ricostruzione dell'immagine (in cui i dati grezzi del sensore vengono convertiti in un'immagine interpretabile), fornendo rapporti segnale/rumore superiori e riducendo gli artefatti indesiderati nelle immagini (Zhu et al., 2018). Nello screening del cancro del polmone, la riduzione del rumore nelle immagini basata sul deep learning ha migliorato sia la qualità delle immagini che l’accuratezza diagnostica della tomografia computerizzata (TC) a dose ultra-bassa per il rilevamento di noduli polmonari sospetti (Hata et al., 2020; Kerpel et al., 2021). Le scansioni che sono state acquisite più velocemente del 40-60% rispetto alle scansioni standard e migliorate con algoritmi basati sul deep learning presentavano una qualità dell'immagine migliore e un valore diagnostico simile alle scansioni standard cerebrali (Bash, Wang, et al., 2021; Rudie et al., 2022) e della colonna vertebrale (Bash, Johnson, et al., 2021). Analogamente, per ridurre specifici artefatti in TC e RM e per migliorare la risoluzione spaziale si possono utilizzare le reti neurali convoluzionali (Hauptmann et al., 2019; K. H. Kim & Park, 2017; Park et al., 2018; Y. Zhang & Yu, 2018).
Gli algoritmi di ricostruzione basati sul deep learning hanno consentito di effettuare scansioni mediante tomografia computerizzata a dose ultra-bassa mantenendo la qualità diagnostica, un aspetto che apporta particolari benefici soprattutto nei bambini e nelle donne in gravidanza, nei quali è di fondamentale importanza ridurre la dose di radiazioni al minimo assoluto. Questi approcci di ricostruzione delle immagini TC basati sul deep learning si associano ad un minor rumore e a una migliore struttura dell'immagine rispetto ad alternative all'avanguardia come la ricostruzione iterativa (Higaki et al., 2020; McLeavy et al., 2021; Singh et al., 2020). Nella tomografia a emissione di positroni, il deep learning può ridurre la dose di tracciante iniettata di un terzo e i tempi di scansione fino alla metà, pur mantenendo la stessa qualità della scansione (Katsari et al., 2021; Le et al., 2020; Xu et al., 2020).
Dopo l'acquisizione delle immagini
I tecnici di radiologia e i medici radiologi di solito condividono il compito di richiamare i pazienti per ripetere gli esami, ma farlo in modo coerente e affidabile è estremamente difficile a causa della mancanza di tempo. È stato dimostrato che la qualità delle immagini delle scansioni di RM cerebrale potenziate dall’IA è uguale o migliore di quella delle scansioni convenzionali anche quando si utilizzano protocolli di acquisizione con tempi di scansione ridotti del 45-60% (Schreiber-Zinaman & Rosenkrantz, 2017).
La prioritizzazione della lettura delle immagini nella lista di lavoro di un radiologo viene spesso effettuata in base a vari fattori, tra cui il tipo di scansione, il reparto richiedente e la comunicazione diretta con il radiologo sull'urgenza dell’esame. Per decidere l’ordine di lettura delle immagini per una migliore efficienza e per garantire che le scansioni più importanti siano visualizzate per prime sono stati testati diversi approcci, tra cui l’assegnazione di specifici esami a diversi radiologi in base alla velocità di lettura di determinati tipi di scansioni (Wong et al., 2019) e il rilevamento automatico dei reperti emergenti sulle immagini, con lo spostamento di questi casi “in cima alla lista” (Prevedello et al., 2017; Winkel et al., 2019). Circa il 70% delle soluzioni basate sull’IA in radiologia si concentra sulla "percezione", una categoria di funzionalità che include la segmentazione, l'estrazione delle caratteristiche, nonché il rilevamento e la classificazione della patologia (Rezazade Mehrizi et al., 2021). All'interno di questa categoria, la maggior parte degli strumenti estrae le informazioni dai dati di imaging con o senza quantificazione e attira l'attenzione dell'utente sulle possibili patologie (Rezazade Mehrizi et al., 2021; van Leeuwen et al., 2021). Negli ultimi anni, alcune delle applicazioni più promettenti di questa categoria sono state il rilevamento di occlusioni dei vasi cerebrali, emorragie cerebrali, noduli polmonari, pneumotorace e versamenti pleurici e fratture, nonché la caratterizzazione delle lesioni mammarie.
Finanziamento
Tra il 2014 e il 2019, il totale degli investimenti fatti nelle aziende di imaging medico basate sull’IA è stato pari a 1,17 miliardi di dollari (Alexander et al., 2020). Nello stesso periodo il numero di aziende operanti in questo ambito è triplicato, il che ha determinato un calo quasi del 30% negli investimenti medi in ciascuna azienda (Alexander et al., 2020). Tra il 2019 e il 2020, gli investimenti privati nelle aziende di IA sono aumentati del 9,3% (D. Zhang et al., 2021). Entro il 2030 si prevede che gli investimenti in soluzioni basate sull’IA nell’imaging medico supereranno i 3 miliardi di dollari (Tsao, 2020).
Adozione
Negli ultimi anni si sono registrate tendenze positive nell’adozione di strumenti di IA da parte di medici radiologi e tecnici di radiologia. Secondo un sondaggio condotto dall’American College of Radiology (ACR) su 1.861 radiologi, tra il 2015 e il 2020 l’uso dell’IA nei reparti di radiologia è aumentato del 30% (Allen et al., 2021).
Nonostante questa tendenza promettente, l’adozione di strumenti di IA è ampiamente considerata sproporzionatamente bassa rispetto all’importo dei finanziamenti, al numero di aziende e al potenziale percepito di questi strumenti. Il sondaggio dell’ACR spiega in parte il perché e offre uno spunto iniziale da cui sviluppare strategie per migliorare l’adozione dell’IA.
Quasi tre quarti dei radiologi che non utilizzavano l’IA non avevano intenzione di farlo in futuro perché non erano convinti dei suoi benefici o non pensavano che i costi associati fossero giustificabili (Allen et al., 2021). Altri studi hanno dato risultati simili: i radiologi non solo si sono detti scettici sulla capacità degli strumenti di IA, ma hanno indicato come una delle ragioni per non adottarli nel loro ambito professionale il fatto che relativamente pochi di essi sono stati approvati dagli enti normativi (Alexander et al., 2020).
Successo normativo
Fino all’agosto 2019, il 60% delle soluzioni radiologiche disponibili basate sull’IA non disponeva dell’approvazione normativa (Rezazade Mehrizi et al., 2021). Nell’aprile 2020 erano 100 in totale le soluzioni di IA che avevano ottenuto il marchio CE, che costituisce un prerequisito per poterle immettere in commercio come dispositivi medici in Europa (van Leeuwen et al., 2021). Al momento della redazione del presente documento, hanno ottenuto l'approvazione della Food and Drug Administration (FDA) statunitense più di 150 soluzioni di IA (AI Central, senza data). Attualmente esistono numerosi database utili di soluzioni basate sull’IA approvate o omologate per il settore dell’assistenza sanitaria (AI Central, senza data;AI for Radiology, senza data;Medical AI Evaluation, senza data;The Medical Futurist, senza data).
Il futuro dell’intelligenza artificiale nell’imaging medico
Negli ultimi anni si è assistito a una crescita esponenziale dell’interesse per l’IA nell’imaging medico, sia in termini di quantità di ricerca che di quantità di denaro investito nel campo. Questo interesse abbraccia l’intero flusso di lavoro radiologico, ma finora hanno dominato le applicazioni concentrate sulla “percezione”, ossia quelle per la quantificazione dei biomarcatori e per l’individuazione dei processi patologici. Nella comunità radiologica si è osservato uno spostamento dalla percezione dell’IA come un intruso sgradito a una maggiore adozione delle soluzioni basate su di essa, seppur con un certo scetticismo ed esitazione riguardo al suo valore. Le prime soluzioni di IA nell’imaging medico hanno ottenuto l’approvazione normativa e abbiamo osservato le prime indicazioni su come tali soluzioni potrebbero essere rimborsate.
Nuove direzioni
A mano a mano che si riconoscerà che gran parte delle potenzialità dell’IA nell’imaging medico potrebbero risiedere in applicazioni “a monte” o “non interpretative”, è probabile che il campo si allargherà nei prossimi anni. Vedremo dunque maggiori ricerche su applicazioni che potrebbero migliorare l’efficienza dei flussi di lavoro radiologici e fornire un’assistenza più personalizzata ai pazienti (Alexander et al., 2020). È probabile che si utilizzerà maggiormente l’IA anche nelle prime fasi del processo di gestione del paziente, ovvero prima che il medico decida che è necessario un esame di imaging. Tali applicazioni, costituite essenzialmente da sistemi di supporto alle decisioni cliniche, sono state utilizzate con successo per il processo decisionale relativo ai trattamenti in vari contesti (Bennett & Hauser, 2013; Komorowski et al., 2018; Bennett & Hauser, 2013). In futuro le soluzioni di IA potrebbero attirare l'attenzione dei medici sulla necessità di eseguire ulteriori esami di imaging sulla base dello studio dei dati clinici che riguardano il paziente, degli esami di laboratorio e degli esami di imaging precedenti (Makeeva et al., 2019).
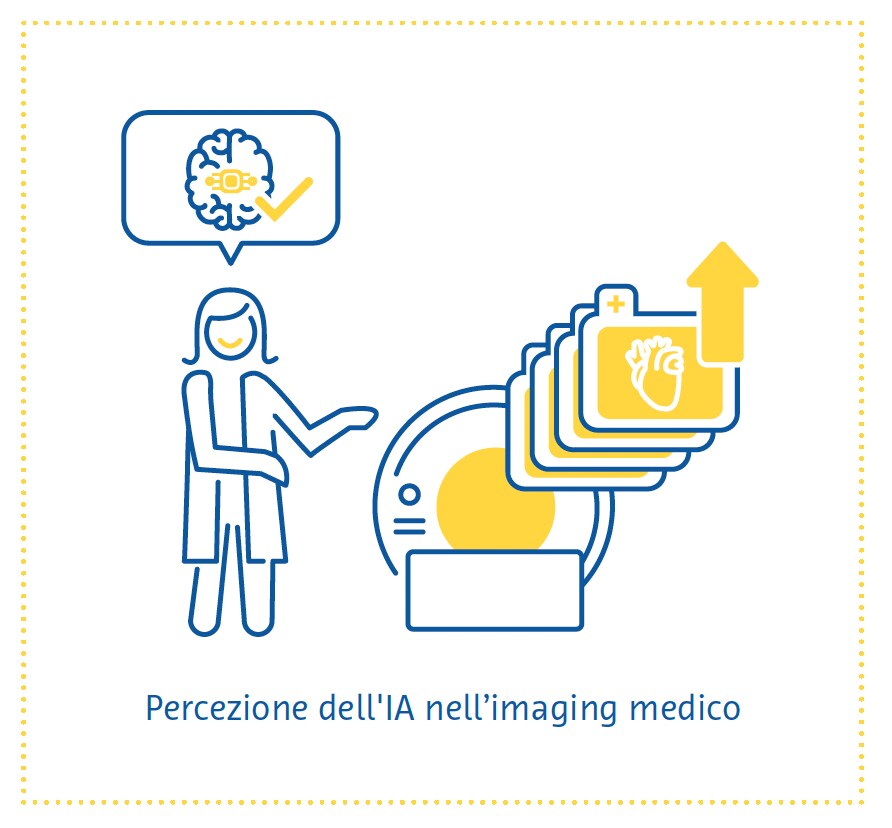
La stragrande maggioranza (77-84%) delle soluzioni di IA attualmente utilizzate nell’imaging medico riguarda TC, RM e radiografie semplici (Rezazade Mehrizi et al., 2021; van Leeuwen et al., 2021). Le tecniche di imaging nucleare, come la tomografia a emissione di positroni (PET), forniscono informazioni uniche, non facili da ottenere con altre modalità. La PET è stata finora largamente trascurata in termini di ricerca sull’IA (Rezazade Mehrizi et al., 2021; van Leeuwen et al., 2021) ed è quindi un ambito potenzialmente promettente per l’espansione del campo.
Si prevede inoltre un grosso cambiamento nel tipo di dati che verranno utilizzati nella ricerca sull’IA. Ogni paziente ricoverato viene sottoposto generalmente a più di un esame di imaging durante il periodo di degenza ospedaliera (Shinagare et al., 2014). Nonostante ciò, solo il 3% circa delle attuali soluzioni radiologiche basate sull’IA combina dati ottenuti con più modalità (Rezazade Mehrizi et al., 2021; van Leeuwen et al., 2021). La combinazione di dati provenienti da più fonti di imaging potrebbe migliorare le capacità diagnostiche delle soluzioni di IA. Inoltre, è probabile che le future soluzioni di IA in radiologia combinino dati ottenuti tramite imaging, informazioni cliniche e test diagnostici non costituiti da immagini (Huang et al., 2020). In questo modo, le soluzioni di IA potrebbero essere in grado di identificare nei dati raccolti durante la degenza ospedaliera di un paziente degli schemi che potrebbero non essere facilmente identificabili dagli operatori sanitari (Rockenbach, 2021). Questo potrebbe portare in ultima istanza a diagnosi più accurate e contribuire alla presa di decisioni terapeutiche migliori e più personalizzate.
È probabile che anche le aspettative nei confronti delle soluzioni di imaging medico basate sull’IA si spostino dall’attuale focus sul triage, sul miglioramento delle immagini e sull’automazione. Con l'aumento della complessità degli algoritmi, della disponibilità dei dati e dell'esperienza con questi strumenti, questo cambiamento potrebbe portare le soluzioni di IA a raggiungere diagnosi specifiche e a raccomandare interventi specifici nel piano di gestione di un paziente. Proprio come l’introduzione dei primi strumenti di IA per lo screening e l’elaborazione delle immagini intorno al 2018 aveva stimolato gli investimenti nel settore, le analisi di marketing prevedono un simile incremento degli investimenti nei prossimi anni man mano che si diffonderanno strumenti di IA capaci di emettere diagnosi specifiche e di determinare interventi di gestione (Michoud et al. al., 2019).
Una delle critiche più importanti che vengono mosse all’attuale, probabilmente ancora nascente, panorama dell’IA nell’imaging medico è che è troppo frammentato. I radiologi professionisti apprezzerebbero piuttosto un’integrazione più snella delle soluzioni di IA nel loro flusso di lavoro quotidiano. Ciò include la perfetta integrazione di queste soluzioni nei flussi di lavoro radiologici consolidati, con la maggior quantità possibile di processi che avvengono "in background" senza l'intervento dell'utente. Inoltre, i risultati di queste soluzioni potrebbero essere integrati nei sistemi informatici radiologici esistenti. Di conseguenza, il campo potrebbe spostarsi dalla sovrabbondanza di soluzioni di IA di nicchia attualmente disponibili, ciascuna mirata a una singola applicazione molto specifica, a suite software più ampie che svolgano molteplici funzioni diverse per una determinata modalità di imaging o area del corpo.
L’investimento frammentato in IA nel mercato dell’imaging medico (Alexander et al., 2020) promuove l’innovazione, consentendo a molti attori di testare diverse strategie in questo campo emergente. Tuttavia, nel lungo termine il consolidamento potrebbe aumentare l’adozione di tali soluzioni e stimolarne l’integrazione diretta nei flussi di lavoro esistenti, consentendo a un minor numero di aziende di offrire queste soluzioni su larga scala (Alexander et al., 2020).
Sfide
Qualità e comunicazione delle evidenze
In una revisione di 100 soluzioni di intelligenza artificiale con marchio CE, il 64% di esse non disponeva di evidenze scientifiche sottoposte a revisione paritaria dell’efficacia (van Leeuwen et al., 2021). Laddove esistevano evidenze scientifiche, il livello era basso, raramente superiore alla dimostrazione dell’accuratezza diagnostica (van Leeuwen et al., 2021). Un’altra revisione sistematica delle evidenze a favore degli algoritmi di deep learning nell’imaging medico ha rilevato un’accuratezza diagnostica generalmente elevata, seppur con un alto rischio di bias tra gli studi (Aggarwal et al., 2021). Le principali fonti di bias sono la mancanza di validazione esterna (D.W. Kim et al., 2019; Liu et al., 2019), una refertazione non sufficientemente dettagliata dei risultati (Liu et al., 2019), un disegno degli studi di tipo retrospettivo (Nagendran et al., 2020) e l’inaccessibilità di dati e codici da parte dei revisori e dei lettori (Nagendran et al., 2020).
Nel complesso, gli studi sugli strumenti di IA hanno mostrato una preoccupante mancanza di refertazione standardizzata e di aderenza alle linee guida di refertazione raccomandate (Aggarwal et al., 2021; Yusuf et al., 2020), nonostante siano attualmente disponibili diverse estensioni delle linee guida di refertazione consolidate, nonché linee guida specifiche per l’IA (Shelmerdine et al., 2021). L’implementazione diffusa di queste linee guida dovrebbe essere al centro dell’attenzione degli sviluppatori di IA in futuro.
Gli sviluppatori di IA dovrebbero anche essere a conoscenza del fatto che il livello di evidenza attualmente “accettabile” per le soluzioni basate sull’IA diventerà probabilmente obsoleto nel prossimo futuro. Sia gli enti normativi che i potenziali utenti probabilmente richiederanno livelli di evidenza più elevati per queste soluzioni, simili a quelli previsti per i nuovi farmaci. Nei prossimi anni molte di queste soluzioni di IA verranno testate in studi clinici randomizzati. In un futuro più lontano, è plausibile che tali aspettative vadano oltre la semplice fornitura di evidenze sulla sicurezza, sull’efficacia o sulle prestazioni diagnostiche di queste soluzioni, per arrivare alla dimostrazione della loro capacità di dare un valore aggiunto monetario o sociale.
Raccogliere la sfida di migliorare la qualità e la comunicazione delle evidenze per le soluzioni basate sull’IA può ripagare nel lungo termine: potrebbe ridurre il rischio di bias negli studi sull’IA, consentire una valutazione approfondita e trasparente della qualità degli studi da parte di potenziali utenti ed enti normativi e facilitare l’esecuzione di revisioni sistematiche e meta-analisi. Questi processi potrebbero aumentare la fiducia nelle soluzioni basate sull’IA e la loro adozione, nonché garantire che offrano miglioramenti realistici e sostenibili nella vita delle persone.
Regolamentazione
Vari aspetti dell’intelligenza artificiale pongono delle difficoltà a chi tenta di regolamentarla come fa con altri interventi nel settore sanitario. I meccanismi interni delle soluzioni di IA sono spesso poco trasparenti e difficili da descrivere approfonditamente nelle modalità tradizionalmente previste dagli organismi di regolamentazione.
Gli ultimi anni ci hanno dimostrato che queste difficoltà a livello di regolamentazione sono tutt’altro che irrisolvibili. Sia la Food and Drug Administration statunitense che la Commissione europea hanno recentemente proposto quadri normativi iniziali per le soluzioni di IA (Center for Devices & Radiological Health, 2021; Commissione europea, 2021).
In parte in risposta alla trasparenza necessaria per l’approvazione normativa, i ricercatori hanno compiuto progressi sostanziali nel rendere il processo decisionale dell’IA più comprensibile e spiegabile. Questo movimento verso un’intelligenza artificiale “interpretabile” acquisirà ulteriore slancio nel prossimo futuro, man mano che aumenterà la dipendenza dall’IA per il processo decisionale clinico nella pratica clinica reale.
Questo presenterà molti vantaggi, tra cui la semplificazione dell’approvazione normativa, l’aumento della fiducia in queste soluzioni da parte degli utenti, la riduzione al minimo dei pregiudizi e il miglioramento della riproducibilità di queste soluzioni (Holzinger et al., 2017; Kolyshkina & Simoff, 2021; “Towards Trustable Machine Learning”, 2018; Yoon et al., 2021).
Riservatezza dei dati
Dalle fasi di sviluppo e collaudo all'implementazione, le soluzioni di IA nell'imaging medico richiedono l'accesso ai dati dei pazienti. Questo aspetto ha sollevato preoccupazioni relative alla riservatezza dei dati, una questione poliedrica e altamente complessa (Murdoch, 2021) ben rappresentata nei percorsi normativi di vari Paesi (COCIR, the European Coordination Committee of the Radiological, Electromedical and Healthcare IT Industry, 2020). Le soluzioni suggerite per la questione della riservatezza dei dati spaziano da quelle incentrate sulla supervisione ad approcci più tecnici.
I pazienti che forniscono i dati devono essere consapevoli di quello che stanno facendo ed essere informati sul perché e come verranno utilizzati i loro dati (Lotan et al., 2020), come esplicitamente previsto dal Regolamento generale sulla protezione dei dati (GDPR – Testo giuridico ufficiale, 2016). Data la velocità di sviluppo delle soluzioni di IA, ci si è chiesti se sia possibile tenere sufficientemente informati i pazienti visto il continuo “riaddestramento” di questi algoritmi (Kritikos, 2020). Sebbene i dati completamente anonimizzati non siano soggetti a requisiti così rigorosi ai sensi del GDPR (What Is Personal Data?, 2021), è estremamente difficile anonimizzare i dati ottenuti con l’imaging medico.
La questione della riservatezza dovrà essere affrontata su più fronti. Oltre alle leggi che regolamentano l’uso dei dati dei pazienti, sta diventando sempre più chiaro che tutti coloro che sono coinvolti nello sviluppo e nell’uso delle soluzioni di IA (sviluppatori, pagatori, organismi di regolamentazione, ricercatori e radiologi) hanno un ruolo da svolgere nel garantire la protezione e l’uso responsabile dei dati.
Inoltre, probabilmente nei prossimi anni verranno svolte ulteriori ricerche sugli approcci tecnici per rafforzare la protezione dei dati, ovvero si cercheranno metodi migliori per ridurre le possibilità che dai dati si possa risalire all’identità personale, metodi per tenere archiviati localmente i dati sensibili anche quando l’algoritmo che si sta addestrando è ospitato in una ubicazione “centrale”, la perturbazione dei dati per ridurre al minimo le informazioni che si trovano all’interno di un determinato set di dati relativo a singoli pazienti e la crittografia dei dati (G. Kaissis et al., 2021; G. A. Kaissis et al., 2020).
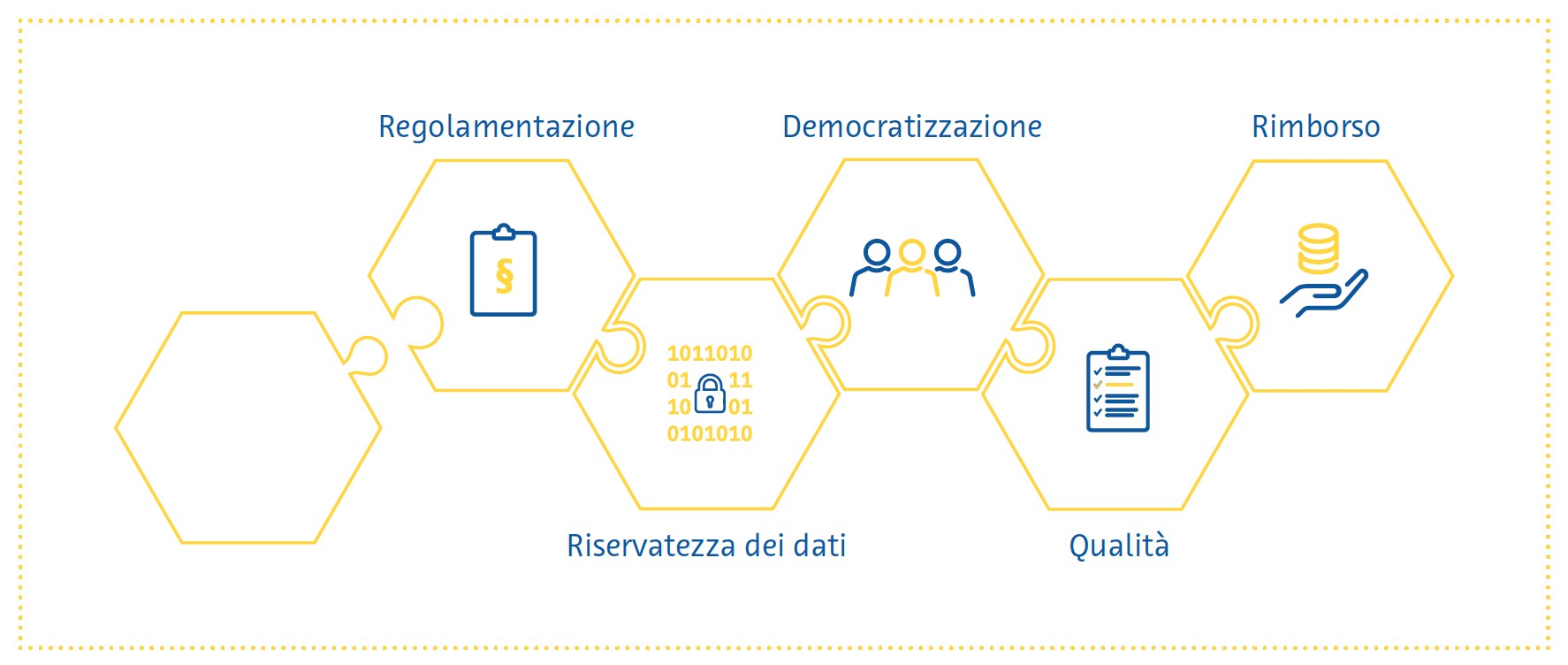
Democratizzazione
Per far sì che l’IA mantenga tutte le sue promesse nel campo dell’imaging medico, gli algoritmi in fase di sviluppo devono funzionare per tutti. Per questa “democratizzazione” dell’IA occorre che gli operatori sanitari abbiano le conoscenze e le competenze necessarie per utilizzare le soluzioni basate sull’IA. Con poche eccezioni (Paranjape et al., 2019), i programmi di studio degli studenti di medicina attualmente includono raramente, se non mai, formazione in materia di IA (Banerjee et al., 2021; Blease et al., 2022). I sondaggi condotti in tutto il mondo hanno dimostrato che l'esposizione degli studenti di medicina e dei medici (Ahmed et al., 2022; Bisdas et al., 2021; Collado-Mesa et al., 2018; Kansal et al., 2022; Pinto Dos Santos et al., 2019; Sit et al., 2020) all’IA nel corso del loro percorso educativo è bassa nonostante l'elevata domanda di maggiore formazione in tale ambito (Kansal et al., 2022; Ooi et al., 2021; Sit et al., 2020). Inoltre, c’è ancora un grande divario tra i sessi e tra diversi Paesi nella conoscenza percepita dell’IA tra gli studenti di medicina (Bisdas et al., 2021). Le ragioni di queste differenze sono molte, e molte sono le difficoltà associate all’integrazione diffusa dell’istruzione sull’IA nei programmi di formazione medica. Nei prossimi anni si dovrebbero studiare strategie per affrontare questi problemi, al fine di garantire che i futuri operatori sanitari siano dotati delle conoscenze e delle competenze necessarie per lavorare in un ambiente in cui l’IA svolge un ruolo crescente.
Democratizzare implica anche garantire che pazienti di sessi, stili di vita, etnie e ubicazioni geografiche diverse possano trarre vantaggio dalle soluzioni basate sull’IA. Affinché ciò accada, queste soluzioni devono essere accessibili e le loro prestazioni devono essere generalizzabili. Queste ultime richiedono l’acquisizione di dati diversi da più istituti, e preferibilmente da più Paesi, per l’addestramento delle soluzioni basate sull’IA. Occorre inoltre mettere in atto misure di salvaguardia per garantire che le fonti di bias durante l’intero processo di sviluppo non si propaghino all’algoritmo addestrato (Vokinger et al., 2021), una questione che è venuta alla ribalta solo di recente (Larrazabal et al., 2020; Obermeyer et al., 2019; Seyyed-Kalantari et al., 2021).
Rimborso
Via via che in vari Paesi iniziano gradualmente a prendere forma delle politiche di regolamentazione dell’IA nel settore sanitario, un aspetto importante che merita attenzione è chi pagherà queste soluzioni e in base a quali criteri.
Molti considerano la legge tedesca sull’offerta digitale del 2020 un passo nella giusta direzione per il rimborso delle soluzioni sanitarie digitali. Secondo questa legge, le applicazioni digitali prescritte dai medici sono rimborsabili dall’assicurazione sanitaria pubblica quando si dimostra che sono sicure e conformi alle norme in materia di riservatezza dei dati, nonché che migliorano l’assistenza sanitaria del paziente. Il Regno Unito, invece, ha pubblicato una guida per i potenziali acquirenti di soluzioni basate sull’IA che funge da punto di partenza per le aziende che intendono presentare domanda di rimborso (A Buyer’s Guide to AI in Health and Care, 2020).
Finora le storie finite bene in materia di rimborsi nel mondo della sanità digitale sono state poche e rare (Brink-mann-Sass et al., 2020; Hassan, 2021), in parte perché i requisiti variano notevolmente da Paese a Paese (COCIR, the European Coordination Committee of the Radiological, Electromedical and Healthcare IT Industry, 2020). In generale, i fornitori di soluzioni sanitarie digitali dovranno fornire prove del valore complessivo apportato da tali soluzioni, anche con studi dettagliati di economia sanitaria che mostrino i potenziali risparmi sui costi.
In virtù della posizione della radiologia come fornitore di servizi per più reparti ospedalieri, l’aspettativa è che le soluzioni basate sull’IA in questo ambito abbiano un impatto di vasta portata (van Duffelen, 2021). Le aziende dovranno mostrare il valore che esse apportano sia a breve termine (ad esempio, in una lettura e refertazione delle immagini più rapida/ migliore) che a lungo termine (ad esempio, diagnosi e trattamento precoci, prevenzione di malattie, riduzione di follow-up non necessari). I prossimi anni vedranno le aziende competere per dimostrare tale impatto, sperimentando allo stesso tempo diversi modelli di prezzo e orientandosi nel panorama burocratico dei rimborsi in evoluzione.
Conclusione
Negli ultimi anni il campo dell’IA nell’imaging medico ha subito una trasformazione rapida ma costante. L’IA oggi può ottenere risultati in radiologia che pochi ritenevano possibili solo dieci anni fa. Il settore sta inoltre gradualmente superando uno degli aspetti maggiormente percepiti come un ostacolo: l’approvazione normativa. Inoltre, se qualche anno fa il futuro dell’IA nella loro specialità era percepito dai radiologi principalmente con timore e scetticismo, oggi non è più così.
Si prevede che gli enormi progressi e l’interesse nel campo dell’IA nell’imaging medico continueranno nel 2022 e oltre. Il settore ha di fronte a sé varie trasformazioni entusiasmanti: probabilmente nei prossimi anni si concentrerà maggiormente sul miglioramento dell’efficienza del flusso di lavoro radiologico, coinvolgerà modalità di imaging finora trascurate, combinerà dati provenienti da più modalità e fornirà previsioni diagnostiche e raccomandazioni per la gestione dei pazienti più concrete. Nei flussi di lavoro radiologici di oggi verranno integrate suite software complete e facili da utilizzare che si avvarranno dell'IA per rendere più semplice ed efficiente il lavoro dei medici radiologi e dei tecnici di radiologia.
Come accade in tutti i campi a crescita rapida, anche l’IA nell’imaging medico ha di fronte svariate sfide scientifiche, normative ed economiche da affrontare, ma gli ultimi anni ci hanno dimostrato che si possono risolvere anche i problemi più difficili. Gli sviluppatori e gli utenti di soluzioni basate sull’IA devono essere consapevoli di queste problematiche, in modo da poter adattare le loro strategie per affrontare le sempre diverse aspettative a livello normativo e sociale. Questo consentirà loro di prosperare in un campo affascinante che ha le potenzialità per migliorare praticamente ogni aspetto dell’assistenza sanitaria.
Bibliografia
AAMC Report Reinforces Mounting Physician Shortage. (2021). AAMC. https://www.aamc.org/news-insights/press- releases/aamc-report-reinforces-mounting-physician-shortage
A buyer’s guide to AI in health and care. (2020). NHS Transformation Directorate. https://www.nhsx.nhs.uk/ai-lab/ explore-all-resources/adopt-ai/a-buyers-guide-to-ai-in-health- and-care/
Aggarwal, R., Sounderajah, V., Martin, G., Ting, D. S. W., Karthikesalingam, A., King, D., Ashrafian, H., & Darzi, A. (2021). Diagnostic accuracy of deep learning in medical imaging: a systematic review and meta-analysis. NPJ Digital Medicine, 4(1), 65.
Ahmed, Z., Bhinder, K. K., Tariq, A., Tahir, M. J., Mehmood, Q., Tabassum, M. S., Malik, M., Aslam, S., Asghar, M. S., & Yousaf, Z. (2022). Knowledge, attitude, and practice of artificial intelligence among doctors and medical students in Pakistan: A cross-sectional online survey. Annals of Medicine and Surgery (2012), 76, 103493.
AI Central. (n.d.). Retrieved February 23, 2022, from https://aicentral.acrdsi.org/
AI for Radiology. (n.d.). Retrieved February 23, 2022, from https://grand-challenge.org/aiforradiology/
Alexander, A., Jiang, A., Ferreira, C., & Zurkiya, D. (2020). An Intelligent Future for Medical Imaging: A Market Outlook on Artificial Intelligence for Medical Imaging. Journal of the American College of Radiology: JACR, 17(1 Pt B), 165–170.
Allen, B., Agarwal, S., Coombs, L., Wald, C., & Dreyer, K. (2021). 2020 ACR Data Science Institute Artificial Intelligence Survey. Journal of the American College of Radiology: JACR, 18(8), 1153–1159.
Banerjee, M., Chiew, D., Patel, K. T., Johns, I., Chappell, D., Linton, N., Cole, G. D., Francis, D. P., Szram, J., Ross, J., & Zaman, S. (2021). The impact of artificial intelligence on clinical education: perceptions of postgraduate trainee doctors in London (UK) and recommendations for trainers. BMC Medical Education, 21(1), 429.
Bash, S., Johnson, B., Gibbs, W., Zhang, T., Shankaranarayanan, A., & Tanenbaum, L. N. (2021). Deep Learning Image Processing Enables 40 % Faster Spinal MR Scans Which Match or Exceed Quality of Standard of Care : A Prospective Multicenter Multireader Study. Clinical Neuroradiology. https://doi.org/10.1007/s00062-021-01121-2
Bash, S., Wang, L., Airriess, C., Zaharchuk, G., Gong, E., Shankaranarayanan, A., & Tanenbaum, L. N. (2021). Deep Learning Enables 60 % Accelerated Volumetric Brain MRI While Preserving Quantitative Performance: A Prospective, Multicenter, Multireader Trial. AJNR. American Journal of Neuroradiology, 42(12), 2130–2137.
Bennett, C. C., & Hauser, K. (2013). Artificial intelligence framework for simulating clinical decision-making: a Markov decision process approach. Artificial Intelligence in Medicine, 57(1), 9–19.
Bisdas, S., Topriceanu, C.-C., Zakrzewska, Z., Irimia, A.-V., Shakallis, L., Subhash, J., Casapu, M.-M., Leon-Rojas, J., Pinto Dos Santos, D., Andrews, D. M., Zeicu, C., Bouhuwaish, A. M., Lestari, A. N., Abu-Ismail, L. ’i, Sadiq, A. S., Khamees, A. ’atasim, Mohammed, K. M. G., Williams, E., Omran, A. I.,… Ebrahim, E. H. (2021). Artificial Intelligence in Medicine: A Multinational Multi-Center Survey on the Medical and Dental Students’ Perception. Frontiers in Public Health, 9, 795284.
Blease, C., Kharko, A., Bernstein, M., Bradley, C., Houston, M., Walsh, I., Hägglund, M., DesRoches, C., & Mandl, K. D. (2022). Machine learning in medical education: a survey of the experiences and opinions of medical students in Ireland. BMJ Health & Care Informatics, 29(1). https://doi.org/10.1136/ bmjhci-2021-100480
Brown, A. D., & Marotta, T. R. (2018). Using machine learning for sequence-level automated MRI protocol selection in neuroradiology. Journal of the American Medical Informatics Association: JAMIA, 25(5), 568–571.
Center for Devices, & Radiological Health. (2021, June 22). Digital Health Software Precertification (Pre-Cert) program.
U.S. Food and Drug Administration. https://www.fda.gov/ medical-devices/digital-health-center-excellence/digital-health- software-precertification-pre-cert-program
Clinical radiology UK workforce census 2019 report. (2019). https://www.rcr.ac.uk/publication/clinical-radiology-uk- workforce-census-2019-report
COCIR, the European Coordination Committee of the Radiological, Electromedical and Healthcare IT Industry. (2020). Market Access Pathways for Digital Health Solutions. https://www.cocir.org/fileadmin/Publications_2020/20062_ COCIR_Market_Access_Pathways_Digital_Health.pdf
Collado-Mesa, F., Alvarez, E., & Arheart, K. (2018). The Role of Artificial Intelligence in Diagnostic Radiology: A Survey at a Single Radiology Residency Training Program. Journal of the American College of Radiology: JACR, 15(12), 1753–1757.
Core Health Indicators in the WHO European Region 2015. Special focus: Human resources for health. (2017, August 14). World Health Organization. https://www.euro.who.int/en/ data-and-evidence/evidence-resources/core-health-indicators- in-the-who-european-region/core-health-indicators-in-the- who-european-region-2015.-special-focus-human-resources- for-health
Dantas, L. F., Fleck, J. L., Cyrino Oliveira, F. L., & Hamacher, S. (2018). No-shows in appointment scheduling - a systematic literature review. Health Policy, 122(4), 412–421.
Esses, S. J., Lu, X., Zhao, T., Shanbhogue, K., Dane, B., Bruno, M., & Chandarana, H. (2018). Automated image quality evaluation of T2 -weighted liver MRI utilizing deep learning architecture. Journal of Magnetic Resonance Imaging: JMRI, 47(3), 723–728.
European Commission. (2021). Proposal for a Regulation Of The European Parliament And Of The Council Laying Down
Harmonised Rules On Artificial Intelligence (Artificial Intelligence Act) And Amending Certain Union Legislative Act. https://eur-lex. europa.eu/resource.html?uri=cellar:e0649735-a372-11eb- 9585-01aa75ed71a1.0001.02/DOC_1&format=PDF
General Data Protection Regulation (GDPR) – Official Legal Text. (2016, July 13). General Data Protection Regulation (GDPR). https://gdpr-info.eu/
Hata, A., Yanagawa, M., Yoshida, Y., Miyata, T., Tsubamoto, M., Honda, O., & Tomiyama, N. (2020). Combination of Deep Learning-Based Denoising and Iterative Reconstruction for Ultra-Low-Dose CT of the Chest: Image Quality and Lung-RADS Evaluation. AJR. American Journal of Roentgenology, 215(6), 1321–1328.
Hauptmann, A., Arridge, S., Lucka, F., Muthurangu, V., & Steeden, J. A. (2019). Real-time cardiovascular MR with
spatio-temporal artifact suppression using deep learning-proof of concept in congenital heart disease. Magnetic Resonance in Medicine: Official Journal of the Society of Magnetic Resonance in Medicine / Society of Magnetic Resonance in Medicine, 81(2), 1143–1156.
Higaki, T., Nakamura, Y., Zhou, J., Yu, Z., Nemoto, T., Tatsugami, F., & Awai, K. (2020). Deep Learning Reconstruction at CT: Phantom Study of the Image Characteristics. Academic Radiology, 27(1), 82–87.
Holzinger, A., Biemann, C., Pattichis, C. S., & Kell, D. B. (2017). What do we need to build explainable AI systems for the medical domain? In arXiv [cs.AI]. arXiv. http://arxiv.org/ abs/1712.09923
Hötker, A. M., Da Mutten, R., Tiessen, A., Konukoglu, E., & Donati, O. F. (2021). Improving workflow in prostate MRI: AI- based decision-making on biparametric or multiparametric MRI. Insights into Imaging, 12(1), 112.
Huang, S.-C., Pareek, A., Seyyedi, S., Banerjee, I., & Lungren, M. P. (2020). Fusion of medical imaging and electronic health records using deep learning: a systematic review and implementation guidelines. NPJ Digital Medicine, 3, 136.
Kaissis, G. A., Makowski, M. R., Rückert, D., & Braren, R. F. (2020). Secure, privacy-preserving and federated machine learning in medical imaging. Nature Machine Intelligence, 2(6), 305–311.
Kaissis, G., Ziller, A., Passerat-Palmbach, J., Ryffel, T., Usynin, D., Trask, A., Lima, I., Mancuso, J., Jungmann, F., Steinborn, M.-M., Saleh, A., Makowski, M., Rueckert, D., & Braren, R. (2021). End-to-end privacy preserving deep learning on multi-institutional medical imaging. Nature Machine Intelligence, 3(6), 473–484.
Kansal, R., Bawa, A., Bansal, A., Trehan, S., Goyal, K., Goyal, N., & Malhotra, K. (2022). Differences in Knowledge and Perspectives on the Usage of Artificial Intelligence Among Doctors and Medical Students of a Developing Country: A Cross- Sectional Study. Cureus, 14(1), e21434.
Katsari, K., Penna, D., Arena, V., Polverari, G., Ianniello, A., Italiano, D., Milani, R., Roncacci, A., Illing, R. O., & Pelosi, E. (2021). Artificial intelligence for reduced dose 18F-FDG PET examinations: a real-world deployment through a standardized framework and business case assessment. EJNMMI Physics, 8(1), 25.
Kerpel, A., Marom, E. M., Green, M., Eifer, M., Konen, E., Mayer, A., & Betancourt Cuellar, S. L. (2021). Ultra-Low Dose Chest CT with Denoising for Lung Nodule Detection. The Israel Medical Association Journal: IMAJ, 23(9), 550–555.
Kim, D. W., Jang, H. Y., Kim, K. W., Shin, Y., & Park, S. H. (2019).
Design Characteristics of Studies Reporting the Performance of Artificial Intelligence Algorithms for Diagnostic Analysis of
Medical Images: Results from Recently Published Papers. Korean Journal of Radiology: Official Journal of the Korean Radiological Society, 20(3), 405–410.
Kim, K. H., & Park, S.-H. (2017). Artificial neural network for suppression of banding artifacts in balanced steady-state free precession MRI. Magnetic Resonance Imaging, 37, 139–146.
Kolyshkina, I., & Simoff, S. (2021). Interpretability of Machine Learning Solutions in Public Healthcare: The CRISP-ML Approach. Frontiers in Big Data, 4, 660206.
Komorowski, M., Celi, L. A., Badawi, O., Gordon, A. C., & Faisal, A. A. (2018). The Artificial Intelligence Clinician learns optimal treatment strategies for sepsis in intensive care. Nature Medicine, 24(11), 1716–1720.
Kritikos, M. (2020). What if artificial intelligence in medical imaging could accelerate Covid-19 treatment? Think Tank
- European Parliament. https://www.europarl.europa.eu/ thinktank/en/document/EPRS_ATA(2020)656333
Kurasawa, H., Hayashi, K., Fujino, A., Takasugi, K., Haga, T., Waki, K., Noguchi, T., & Ohe, K. (2016). Machine-Learning- Based Prediction of a Missed Scheduled Clinical Appointment by Patients With Diabetes. Journal of Diabetes Science and Technology, 10(3), 730–736.
Larrazabal, A. J., Nieto, N., Peterson, V., Milone, D. H., & Ferrante, E. (2020). Gender imbalance in medical imaging datasets produces biased classifiers for computer-aided diagnosis. Proceedings of the National Academy of Sciences of the United States of America, 117(23), 12592–12594.
Larson, D. B., Johnson, L. W., Schnell, B. M., Salisbury, S. R., & Forman, H. P. (2011). National trends in CT use in the emergency department: 1995-2007. Radiology, 258(1), 164–173.
Le, V., Frye, S., Botkin, C., Christopher, K., Gulaka, P., Sterkel, B., Frye, R., Muzaffar, R., & Osman, M. (2020). Effect of PET Scan with Count Reduction Using AI-Based Processing Techniques on Image Quality. Journal of Nuclear Medicine: Official Publication, Society of Nuclear Medicine, 61(supplement 1), 3095–3095.
Levin, D. C., Parker, L., & Rao, V. M. (2017). Recent Trends in Imaging Use in Hospital Settings: Implications for Future Planning. Journal of the American College of Radiology: JACR, 14(3), 331–336.
Liu, X., Faes, L., Kale, A. U., Wagner, S. K., Fu, D. J., Bruynseels, A., Mahendiran, T., Moraes, G., Shamdas, M., Kern, C., Ledsam, J. R., Schmid, M. K., Balaskas, K., Topol, E.J., Bachmann, L. M., Keane, P. A., & Denniston, A. K. (2019). A comparison of deep learning performance against health-care professionals in detecting diseases from medical imaging: a systematic review and meta-analysis. The Lancet. Digital Health, 1(6), e271–e297.
Lotan, E., Tschider, C., Sodickson, D. K., Caplan, A. L., Bruno, M., Zhang, B., & Lui, Y. W. (2020). Medical Imaging and Privacy in the Era of Artificial Intelligence: Myth, Fallacy, and the Future. Journal of the American College of Radiology: JACR, 17(9), 1159–1162.
Mairhöfer, D., Laufer, M., Simon, P. M., Sieren, M., Bischof, A., Käster, T., Barth, E., Barkhausen, J., & Martinetz, T. (2021). An AI-based Framework for Diagnostic Quality Assessment of Ankle Radiographs. https://openreview.net/pdf?id=bj04hJss_xZ
Makeeva, V., Gichoya, J., Hawkins, C. M., Towbin, A. J., Heilbrun, M., & Prater, A. (2019). The Application of Machine Learning to Quality Improvement Through the Lens of the Radiology Value Network. Journal of the American College of Radiology: JACR, 16(9 Pt B), 1254–1258.
McLeavy, C. M., Chunara, M. H., Gravell, R. J., Rauf, A., Cushnie, A., Staley Talbot, C., & Hawkins, R. M. (2021). The future of CT: deep learning reconstruction. Clinical Radiology, 76(6), 407–415.
Medical AI Evaluation. (n.d.). Retrieved February 23, 2022, from https://ericwu09.github.io/medical-ai-evaluation/
Michoud, L., Tschudi, Y., & Villien, Y. (2019). Artificial Intelligence for Medical Imaging: Market and Technology Report 2020. Yole Développement. https://s3.i-micronews.com/ uploads/2020/01/YDR20059-AI-for-Medical-Imaging_Yole_ sample.pdf
Murdoch, B. (2021). Privacy and artificial intelligence: challenges for protecting health information in a new era. BMC Medical Ethics, 22(1), 122.
Nagendran, M., Chen, Y., Lovejoy, C. A., Gordon, A. C., Komorowski, M., Harvey, H., Topol, E. J., Ioannidis, J. P. A., Collins, G. S., & Maruthappu, M. (2020). Artificial intelligence versus clinicians: systematic review of design, reporting standards, and claims of deep learning studies. BMJ, 368. https://doi.org/10.1136/bmj.m689
Nelson, A., Herron, D., Rees, G., & Nachev, P. (2019). Predicting scheduled hospital attendance with artificial intelligence. Npj Digital Medicine, 2(1), 26.
Obermeyer, Z., Powers, B., Vogeli, C., & Mullainathan, S. (2019). Dissecting racial bias in an algorithm used to manage the health of populations. Science, 366(6464), 447–453.
Ooi, S. K. G., Makmur, A., Soon, A. Y. Q., Fook-Chong, S., Liew, C., Sia, S. Y., Ting, Y. H., & Lim, C. Y. (2021). Attitudes toward artificial intelligence in radiology with learner needs assessment within radiology residency programmes: a national multi-programme survey. Singapore Medical Journal, 62(3), 126–134.
Paranjape, K., Schinkel, M., Nannan Panday, R., Car, J., & Nanayakkara, P. (2019). Introducing Artificial Intelligence Training in Medical Education. JMIR Medical Education, 5(2), e16048.
Park, J., Hwang, D., Kim, K. Y., Kang, S. K., Kim, Y. K., & Lee, J. S. (2018). Computed tomography super-resolution using deep convolutional neural network. Physics in Medicine and Biology, 63(14), 145011.
Pinto Dos Santos, D., Giese, D., Brodehl, S., Chon, S. H., Staab, W., Kleinert, R., Maintz, D., & Baeßler, B. (2019). Medical students’ attitude towards artificial intelligence: a multicentre survey. European Radiology, 29(4), 1640–1646.
Population ages 65 and above. (n.d.). The World Bank. Retrieved February 23, 2022, from https://data.worldbank.org/ indicator/SP.POP.65UP.TO.ZS
Prevedello, L. M., Erdal, B. S., Ryu, J. L., Little, K. J., Demirer, M., Qian, S., & White, R. D. (2017). Automated Critical Test Findings Identification and Online Notification System Using Artificial Intelligence in Imaging. Radiology, 285(3), 923–931.
Rezazade Mehrizi, M. H., van Ooijen, P., & Homan, M. (2021). Applications of artificial intelligence (AI) in diagnostic radiology: a technography study. European Radiology, 31(4), 1805–1811.
Rockenbach, M. A. B. (2021, June 13). Multimodal AI in healthcare: Closing the gaps. CodeX. https://medium.com/codex/ multimodal-ai-in-healthcare-1f5152e83be2
Rudie, J. D., Gleason, T., Barkovich, M. J., Wilson, D. M., Shankaranarayanan, A., Zhang, T., Wang, L., Gong, E., Zaharchuk, G., & Villanueva-Meyer, J. E. (2022). Clinical Assessment of Deep Learning–based Super-Resolution for 3D Volumetric Brain MRI. Radiology: Artificial Intelligence, e210059.
Schreiber-Zinaman, J., & Rosenkrantz, A. B. (2017). Frequency and reasons for extra sequences in clinical abdominal MRI examinations. Abdominal Radiology (New York), 42(1), 306–311.
Seyyed-Kalantari, L., Zhang, H., McDermott, M. B. A., Chen, I. Y., & Ghassemi, M. (2021). Underdiagnosis bias of artificial intelligence algorithms applied to chest radiographs in under-served patient populations. Nature Medicine, 27(12), 2176–2182.
Shelmerdine, S. C., Arthurs, O. J., Denniston, A., & Sebire, N. J. (2021). Review of study reporting guidelines for clinical studies using artificial intelligence in healthcare. BMJ Health & Care Informatics, 28(1). https://doi.org/10.1136/ bmjhci-2021-100385
Shinagare, A. B., Ip, I. K., Abbett, S. K., Hanson, R., Seltzer, S. E., & Khorasani, R. (2014). Inpatient imaging utilization: trends of the past decade. AJR. American Journal of Roentgenology, 202(3), W277–W283.
Singh, R., Digumarthy, S. R., Muse, V. V., Kambadakone, A. R., Blake, M. A., Tabari, A., Hoi, Y., Akino, N., Angel, E., Madan, R., & Kalra, M. K. (2020). Image Quality and Lesion Detection on Deep Learning Reconstruction and Iterative Reconstruction of Submillisievert Chest and Abdominal CT. AJR. American Journal of Roentgenology, 214(3), 566–573.
Sit, C., Srinivasan, R., Amlani, A., Muthuswamy, K., Azam, A., Monzon, L., & Poon, D. S. (2020). Attitudes and perceptions of UK medical students towards artificial intelligence and radiology: a multicentre survey. Insights into Imaging, 11(1), 14.
Smith-Bindman, R., Miglioretti, D. L., Johnson, E., Lee, C., Feigelson, H. S., Flynn, M., Greenlee, R. T., Kruger, R. L., Hornbrook, M. C., Roblin, D., Solberg, L. I., Vanneman, N., Weinmann, S., & Williams, A. E. (2012). Use of diagnostic imaging studies and associated radiation exposure for patients enrolled in large integrated health care systems, 1996-2010. JAMA: The Journal of the American Medical Association, 307(22), 2400–2409.
Smith-Bindman, R., Miglioretti, D. L., & Larson, E. B. (2008). Rising use of diagnostic medical imaging in a large integrated health system. Health Affairs, 27(6), 1491–1502.
The Medical Futurist. (n.d.). The Medical Futurist. Retrieved February 23, 2022, from https://medicalfuturist.com/fda- approved-ai-based-algorithms/
Towards trustable machine learning. (2018). Nature Biomedical Engineering, 2(10), 709–710.
Trivedi, H., Mesterhazy, J., Laguna, B., Vu, T., & Sohn, J. H. (2018). Automatic Determination of the Need for Intravenous Contrast in Musculoskeletal MRI Examinations Using IBM Watson’s Natural Language Processing Algorithm. Journal of Digital Imaging, 31(2), 245–251.
Tsao, D. N. (2020, July 27). AI in medical diagnostics 2020- 2030: Image recognition, players, clinical applications, forecasts: IDTechEx. https://www.idtechex.com/en/research- report/ai-in-medical-diagnostics-2020-2030-image- recognition-players-clinical-applications-forecasts/766
van Duffelen, J. (2021, February 22). Making a case for buying medical imaging AI: How to define the return on investment.
Aidence. https://www.aidence.com/articles/medical-imaging- ai-roi/
van Leeuwen, K. G., Schalekamp, S., Rutten, M. J. C. M., van Ginneken, B., & de Rooij, M. (2021). Artificial intelligence in radiology: 100 commercially available products and their scientific evidence. European Radiology, 31(6), 3797–3804.
Vokinger, K. N., Feuerriegel, S., & Kesselheim, A. S. (2021). Mitigating bias in machine learning for medicine. Communication & Medicine, 1, 25.
Wang, S., Cao, G., Wang, Y., Liao, S., Wang, Q., Shi, J., Li, C., & Shen, D. (2021). Review and Prospect: Artificial Intelligence in Advanced Medical Imaging. Frontiers in Radiology, 1. https://doi.org/10.3389/fradi.2021.781868
What is personal data? (2021, January 1). ICO - Information Commissioner’s Office; ICO. https://ico.org.uk/for-organisations/ guide-to-data-protection/guide-to-the-general-data-protection- regulation-gdpr/what-is-personal-data/what-is-personal-data
WHO. (n.d.-a). Ageing and health. Retrieved February 23, 2022, from https://www.who.int/news-room/fact-sheets/detail/ ageing-and-health
WHO. (n.d.-b). Noncommunicable diseases. Retrieved March 6, 2022, from https://www.who.int/news-room/fact-sheets/detail/ noncommunicable-diseases
Winder, M., Owczarek, A. J., Chudek, J., Pilch-Kowalczyk, J., & Baron, J. (2021). Are We Overdoing It? Changes in Diagnostic Imaging Workload during the Years 2010-2020 including the Impact of the SARS-CoV-2 Pandemic. Healthcare (Basel, Switzerland), 9(11). https://doi.org/10.3390/healthcare9111557
Winkel, D. J., Heye, T., Weikert, T. J., Boll, D. T., & Stieltjes, B. (2019). Evaluation of an AI-Based Detection Software for Acute Findings in Abdominal Computed Tomography Scans: Toward an Automated Work List Prioritization of Routine CT Examinations. Investigative Radiology, 54(1), 55–59.
Wong, T. T., Kazam, J. K., & Rasiej, M. J. (2019). Effect of Analytics-Driven Worklists on Musculoskeletal MRI
Interpretation Times in an Academic Setting. AJR. American Journal of Roentgenology, 1–5.
Xu, F., Pan, B., Zhu, X., Gulaka, P., Xiang, L., Gong, E., Zhang, T., Wang, J., Lin, L., Ma, Y., & Gong, N.-J. (2020). Evaluation of Deep Learning Based PET Image Enhancement Method in Diagnosis of Lymphoma. Journal of Nuclear Medicine: Official Publication, Society of Nuclear Medicine, 61(supplement 1), 431–431.
Yoon, C. H., Torrance, R., & Scheinerman, N. (2021). Machine learning in medicine: should the pursuit of enhanced interpretability be abandoned? Journal of Medical Ethics. https://doi.org/10.1136/medethics-2020-107102
Yusuf, M., Atal, I., Li, J., Smith, P., Ravaud, P., Fergie, M., Callaghan, M., & Selfe, J. (2020). Reporting quality of studies using machine learning models for medical diagnosis: a systematic review. BMJ Open, 10(3), e034568.
Zhang, D., Mishra, S., Brynjolfsson, E., Etchemendy, J., Ganguli, D., Grosz, B., Lyons, T., Manyika, J., Niebles, J. C., Sellitto, M., Shoham, Y., Clark, J., & Perrault, R. (2021). The AI Index 2021 Annual Report. In arXiv [cs.AI]. arXiv. http://arxiv.org/ abs/2103.06312
Zhang, Y., & Yu, H. (2018). Convolutional Neural Network Based Metal Artifact Reduction in X-Ray Computed Tomography. IEEE Transactions on Medical Imaging, 37(6), 1370–1381.
Zhu, B., Liu, J. Z., Cauley, S. F., Rosen, B. R., & Rosen, M. S. (2018). Image reconstruction by domain-transform manifold learning. Nature, 555(7697), 487–492.