La inteligencia artificial (IA) es un campo que permite a los sistemas informáticos resolver problemas adaptándose a circunstancias cambiantes, a menudo imitando el razonamiento y el criterio humanos. Diversas tendencias demográficas y sanitarias están impulsando el uso de la IA en el análisis de las imágenes médicas. La cantidad de datos de imágenes médicas que se adquieren aumenta de forma constante (Larson et al., 2011; Smith-Bindman et al., 2008, 2012; Winder et al., 2021). Asimismo, también existe una escasez generalizada de trabajadores sanitarios ( Core Health Indicators in the WHO European Region 2015. Special Focus: Human Resources for Health, 2017) que deben asumir una carga de trabajo cada vez mayor (Levin et al., 2017), y se prevé que el número de exploraciones por imagen aumente de forma exponencial durante las próximas dos décadas (Tsao, 2020). La oferta de radiólogos y técnicos de radiología es especialmente escasa (AAMC Report Reinforces Mounting Physician Shortage, 2021, Clinical Radiology UK Workforce Census 2019 Report, 2019). Por último, se prevé que el envejecimiento de la población mundial (Population Ages 65 and above,s.f.; OMS, s.f.-a) y el aumento de la carga mundial de enfermedades crónicas (OMS, s.f.-b) agraven estos problemas en un futuro próximo.
En términos generales, las ventajas que ofrece la IA en el diagnóstico por la imagen podrían incluir la capacidad de proporcionar información que de otro modo no sería posible con los métodos tradicionales (como la exploración de dichas imágenes por parte de personal médico) y de hacerlo de una forma más rápida y automatizada (sin necesidad de interacción humana). Las soluciones para el análisis de imágenes médicas basadas en inteligencia artificial podrían mejorar y acelerar la detección de enfermedades, evaluar en profundidad los riesgos del desarrollo y la progresión de la enfermedad y reducir la subjetividad en la interpretación de los datos.
El estado actual de la IA en el análisis de las imágenes médicas
En los últimos años, el uso de la IA en el diagnóstico por la imagen ha cambiado drásticamente. Han surgido un gran número de aplicaciones prometedoras, el campo ha experimentado un aumento de financiación sin precedentes y hemos observado tendencias positivas en la adopción de soluciones de IA por parte de los radiólogos, así como en su aprobación por parte de los organismos reguladores.
Aplicaciones
Si bien las unidades de radiología prestan una gran cantidad de servicios, el servicio básico que ofrecen es el estudio por imagen. Las aplicaciones de la IA en el análisis de imágenes pueden clasificarse en las que se aplican antes, durante o después del estudio de imagen.
Antes de la adquisición de imágenes
En el contexto del flujo de trabajo de una unidad de radiología, antes de someter a un paciente a un estudio por imagen deben seguirse varios pasos. Las aplicaciones de IA que pretenden mejorar estos pasos se denominan «IA ascendente» y podrían aumentar la eficiencia y hacer posible una toma de decisiones más personalizada en las unidades de radiología.
Así, por ejemplo, es bien sabido que hay pacientes que no acuden a sus citas médicas. Estas citas médicas perdidas reducen la eficiencia de los hospitales y desperdician recursos (Dantas et al., 2018). Estudios realizados en Japón (Kurasawa et al., 2016) y el Reino Unido (Nelson et al., 2019) han demostrado que la IA se puede utilizar para predecir las ausencias con gran precisión. Esto permite utilizar estrategias específicas para reducir la probabilidad de que un paciente falte a su cita, incluido el envío de recordatorios automáticos.
Una de las decisiones más importantes que se toman en las unidades de radiología es el protocolo exacto de exploración que se utilizará con un paciente determinado. Si bien esto se aplica a todas las modalidades de diagnóstico por la imagen, el mayor abanico de opciones se da en la resonancia magnética (RM), que incluye la elección del conjunto adecuado de secuencias y la toma de decisiones sobre la administración o no de medios de contraste intravenosos. Con este fin, se han utilizado, por ejemplo, clasificadores de lenguaje natural que interpretan el texto narrativo de las solicitudes de exploración del médico para seleccionar los protocolos de RM adecuados. En un estudio, un clasificador de aumento de gradiente predijo, con una elevada precisión (95 %), el protocolo de RM cerebral adecuado a utilizar en función de la solicitud de la exploración (Brown y Marotta, 2018). En el caso de la RM musculoesquelética, un clasificador de aprendizaje profundo ofreció una precisión del 83 % a la hora de determinar la necesidad de administrar un medio de contraste (Trivedi et al., 2018). Estas aplicaciones pueden mejorar de forma significativa la eficiencia, ya que evitan que los radiólogos tengan que dedicar una gran cantidad de tiempo a revisar solicitudes de exploración no estructuradas de los médicos que las solicitan.
Durante la adquisición de imágenes
Recientemente se han introducido mejoras sustanciales en el uso de la IA para mejorar la calidad de las imágenes. En una encuesta reciente, los radiólogos identificaron la mejora de la calidad de la imagen como el caso de uso actual más generalizado de la IA en las técnicas por imagen (Alexander et al., 2020). Si bien los intentos anteriores de reducir el ruido de la imagen utilizando técnicas de aprendizaje profundo fueron criticados por eliminar detalles de las imágenes que ponían en peligro la visibilidad de características esenciales dentro de las imágenes, las implementaciones más recientes han dejado este problema en gran medida obsoleto.
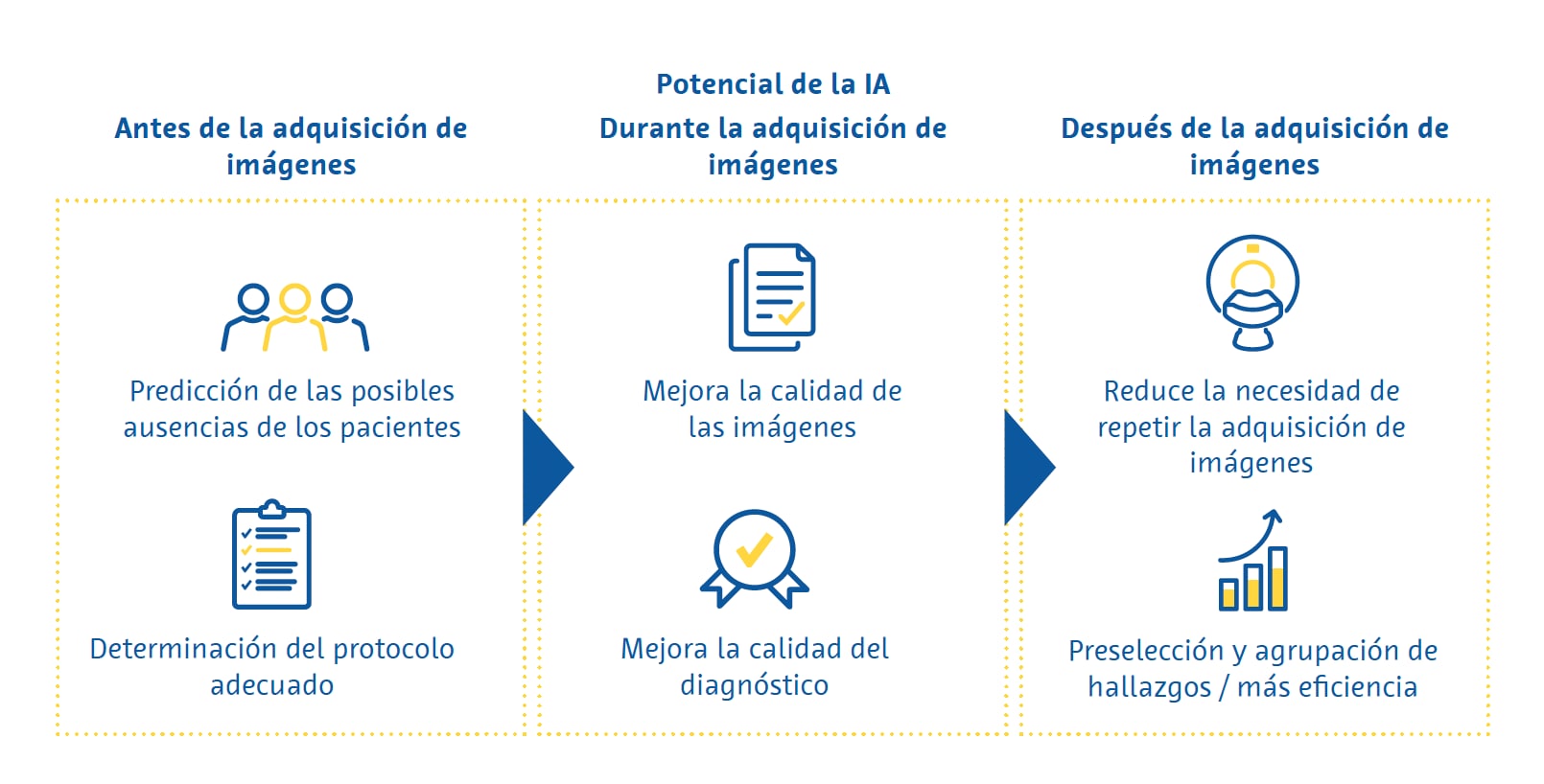
En particular, las técnicas de aprendizaje profundo, como las redes generativas antagónicas, han demostrado un gran potencial en la eliminación de ruido de imágenes (Wang et al., 2021). Algunas de estas aplicaciones se centran en la etapa de reconstrucción de la imagen (en la que los datos brutos del sensor se convierten en una imagen interpretable), proporcionando relaciones señal-ruido superiores y reduciendo los artefactos de la imagen (Zhu et al., 2018). En la detección sistemática del cáncer de pulmón, la eliminación de ruido de imágenes basada en el aprendizaje profundo mejoró tanto la calidad de la imagen como la precisión diagnóstica de la tomografía computarizada (TC) de dosis ultrabaja para detectar nódulos pulmonares sospechosos (Hata et al., 2020; Kerpel et al., 2021). Las exploraciones que se adquirieron entre un 40 % y un 60 % más rápidamente que las exploraciones estándar y se mejoraron con algoritmos basados en aprendizaje profundo ofrecieron una mejor calidad de imagen y un valor diagnóstico similar a las exploraciones estándar del cerebro (Bash, Wang, et al., 2021; Rudie et al., 2022) y la columna vertebral (Bash, Johnson, et al., 2021). Del mismo modo, las redes neuronales convolucionales pueden utilizarse para reducir artefactos específicos de TC y RM y mejorar la resolución espacial (Hauptmann et al., 2019; K. H. Kim & Park, 2017; Park et al., 2018; Y. Zhang & Yu, 2018).
Los algoritmos de reconstrucción basados en el aprendizaje profundo han permitido adquirir tomografías computarizadas de dosis ultrabaja manteniendo la calidad diagnóstica. Esto resulta especialmente beneficioso en niños y mujeres embarazadas, situaciones en las que es fundamental reducir la dosis de radiación al mínimo posible. Estos enfoques de reconstrucción de imágenes de TC basados en el aprendizaje profundo se asocian a un menor ruido de imagen y una mejor textura de la imagen que las alternativas de vanguardia como la reconstrucción iterativa (Higaki et al., 2020; McLeavy et al., 2021; Singh et al., 2020). En la tomografía por emisión de positrones, el aprendizaje profundo puede reducir la dosis de trazador inyectado en un tercio y los tiempos de exploración hasta la mitad, manteniendo la calidad de la exploración (Katsari et al., 2021; Le et al., 2020; Xu et al., 2020).
Después de la adquisición de imágenes
Los técnicos de radiología y los radiólogos suelen compartir la tarea de volver a llamar a los pacientes para repetir las exploraciones, si bien hacerlo de una forma coherente y fiable es sumamente difícil debido a la falta de tiempo. Se ha demostrado que la calidad de la imagen de las RM cerebrales mejoradas con IA es igual o superior que la de las exploraciones convencionales, incluso cuando se utilizan protocolos de adquisición que reducen los tiempos de exploración entre un 45 % y un 60 % (Schreiber-Zinaman & Rosenkrantz, 2017).
Con frecuencia, la priorización de la lectura de la exploración en la lista de trabajo de un radiólogo se basa en varios factores, incluido el tipo de exploración, la unidad que la solicita y la comunicación directa con el radiólogo sobre la urgencia de la misma. Se han explorado diversos enfoques para influir en el orden de lectura de las exploraciones, con el objetivo de mejorar la eficacia y asegurar que las exploraciones más importantes se presenten primero. Estos incluyen asignar a diferentes radiólogos exámenes específicos en función de la rapidez con la que leen ciertos tipos de exploraciones (Wong et al., 2019), detectar automáticamente hallazgos emergentes en las imágenes y situar estos casos en la «parte superior de la lista» (Prevedello et al., 2017; Winkel et al., 2019).
Alrededor del 70 % de todas las soluciones basadas en IA en radiología se centran en la «percepción», una categoría de funcionalidades que incluye la segmentación, la extracción de características, y la detección y clasificación de patologías (Rezazade Mehrizi et al., 2021). Dentro de esta categoría, la mayoría de las herramientas extraen información de los datos de imagen, ya sea con o sin cuantificación, y además alertan al usuario sobre posibles patologías. (Rezazade Mehrizi et al., 2021; van Leeuwen et al., 2021). En los últimos años, algunas de las aplicaciones más prometedoras de esta categoría han sido la detección de la oclusión de vasos cerebrales, hemorragias cerebrales, nódulos pulmonares, neumotórax y derrames pleurales, fracturas y la caracterización de lesiones mamarias.
Financiación
El importe total de la inversión en empresas de imagen médica basadas en IA ascendió a 1170 millones de dólares entre 2014 y 2019 (Alexander et al., 2020). En este mismo período, el número de empresas en este espacio se triplicó, lo que provocó una caída de casi el 30 % en la inversión mediana en cada empresa (Alexander et al., 2020). Entre los años 2019 y 2020, la inversión privada en empresas de IA aumentó un 9,3 % (D. Zhang et al., 2021). Para el año 2030, se prevé que la inversión en soluciones basadas en inteligencia artificial (IA) para imágenes médicas supere los 3 mil millones de dólares (Tsao, 2020).
Adopción
En los últimos años se han observado tendencias positivas en la adopción de herramientas de IA por parte de radiólogos y técnicos de radiología. Entre 2015 y 2020, el uso de IA en las unidades de radiología aumentó un 30 %, según una encuesta realizada por el Colegio Estadounidense de Radiología (ACR), en la que participaron 1861 radiólogos (Allen et al., 2021).
A pesar de esta prometedora tendencia, se considera que la adopción de herramientas de IA es desproporcionadamente baja en relación con la financiación, el número de empresas y la promesa que se asocia a estas herramientas. La encuesta del ACR permite comprender por qué y ofrece un punto de partida para desarrollar estrategias que mejoren la adopción de la IA. Casi tres cuartas partes de los radiólogos que no utilizaban IA no tenían previsto hacerlo en el futuro porque, o bien no estaban convencidos de sus beneficios, o bien no creían que los costes asociados a la misma estuvieran justificados (Allen et al., 2021). Asimismo, se han observado resultados similares en otros estudios, en los que los radiólogos citan el escepticismo en las capacidades de las herramientas de IA y el hecho de que relativamente pocas cuentan con una aprobación reglamentaria, como motivos para no adoptarla en la práctica clínica (Alexander et al., 2020).
Un éxito regulatorio
Hasta agosto de 2019, el 60 % de las soluciones radiológicas basadas en IA disponibles no contaban con una aprobación regulatoria (Rezazade Mehrizi et al., 2021). En abril de 2020, un total de 100 soluciones de IA contaban con la marca CE, un requisito previo para que puedan comercializarse como productos sanitarios en Europa (van Leeuwen et al., 2021). En el momento de escribir este artículo, más de 150 soluciones de IA han obtenido la aprobación de la FDA (AI Central, s.f.). En la actualidad existen varias bases de datos útiles de soluciones basadas en IA aprobadas o autorizadas para la atención sanitaria médica (AI Central, s.f., AI for Radiology, s.f., Medical AI Assessment, s.f., The Medical Futurist, s.f.).
El futuro de la IA en las técnicas por imagen
En los últimos años se ha producido un crecimiento exponencial del interés por la IA en el diagnóstico médico por imagen, tanto en lo que se refiere al volumen de investigación como a la cantidad de dinero que se invierte en este campo. Este interés abarca todo el espectro del flujo de trabajo en radiología, si bien, hasta ahora, las aplicaciones de «percepción» (como la cuantificación de biomarcadores y la detección de procesos patológicos) han sido las más dominantes. En la comunidad radiológica, las tendencias han pasado de percibir la IA como un intruso indeseado a adoptarla cada vez más, aunque con cierto escepticismo y dudas sobre su valor. Las primeras soluciones de IA utilizadas en las técnicas por imagen han recibido la aprobación reglamentaria, y hemos visto los primeros indicios de cómo pueden reembolsarse estas soluciones.
Nuevas direcciones
A medida que se reconoce cada vez más que una gran proporción del potencial de la IA en el diagnóstico médico por imagen puede residir en aplicaciones «previas» o «no interpretativas», es probable que su campo de acción se amplíe en los próximos años. Esto incluirá una mayor investigación sobre aplicaciones que mejoren la eficiencia de los flujos de trabajo de radiología y ofrezcan una atención al paciente más personalizada (Alexander et al., 2020). En este sentido, es probable que la IA se utilice en mayor medida en una fase más temprana del proceso de tratamiento del paciente, es decir, antes de que el médico decida que es necesario realizar una prueba de diagnóstico por la imagen. Estas aplicaciones, fundamentalmente sistemas de apoyo a la toma de decisiones clínicas, han demostrado su eficacia en la toma de decisiones sobre tratamientos en diversos contextos (Bennett & Hauser, 2013; Komorowski et al., 2018), y se han utilizado con éxito en la toma de decisiones sobre tratamientos (Bennett & Hauser, 2013). En el futuro, las soluciones de IA pueden llamar la atención de los médicos sobre la necesidad de llevar a cabo un mayor número de pruebas por imagen basándose en la revisión de la información clínica del paciente, las pruebas analíticas y las pruebas de imagen previas (Makeeva et al., 2019).
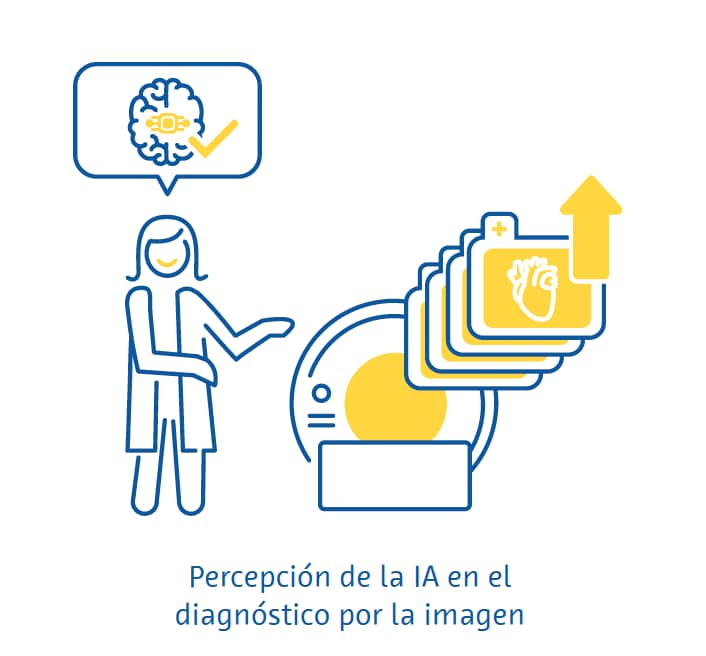
La gran mayoría (77 %-84 %) de las soluciones de IA disponibles actualmente en el ámbito del diagnóstico por la imagen se centran en la TC, la RM y las radiografías simples (Rezazade Mehrizi et al., 2021; van Leeuwen et al., 2021). Las técnicas de imagen nuclear, como la tomografía por emisión de positrones (PET), proporcionan información única que no es posible obtener fácilmente con otras modalidades. Hasta ahora, la investigación en IA ha prestado escasa atención a la PET (Rezazade Mehrizi et al., 2021; van Leeuwen et al., 2021), por lo que es una vía de expansión potencialmente prometedora.
También se espera que la investigación en IA experimente un cambio en el tipo de datos que se utilizan. El paciente hospitalizado típico se somete a más de un estudio por imagen distinto durante su estancia hospitalaria (Shinagare et al., 2014). A pesar de ello, solo alrededor del 3 % de las soluciones radiológicas actuales basadas en IA combinan datos de múltiples modalidades (Rezazade Mehrizi et al., 2021; van Leeuwen et al., 2021). La combinación de datos de múltiples fuentes de imágenes puede mejorar las capacidades de diagnóstico de las soluciones de IA. Además, es probable que las futuras soluciones de IA en radiología combinen información de imágenes, información clínica y pruebas diagnósticas no relacionadas con el diagnóstico por la imagen (Huang et al., 2020). De este modo, las soluciones de IA podrán identificar patrones en los datos recopilados durante la estancia de un paciente en el hospital que no sean fácilmente identificables por el personal sanitario (Rockenbach, 2021). En última instancia, esto podría dar lugar a unos diagnósticos más precisos y contribuir a tomar mejores decisiones terapéuticas más personalizadas.
También es probable que las expectativas para las soluciones del diagnóstico por la imagen basadas en la IA cambien respecto al enfoque actual de triaje, mejora de la imagen y automatización. Con el aumento de la complejidad algorítmica, la disponibilidad de datos y la experiencia con estas herramientas, este cambio puede conllevar a que las soluciones de IA permitan obtener diagnósticos específicos y recomienden pasos específicos en el plan de tratamiento de un paciente. Del mismo modo que la introducción de las primeras herramientas de IA para el cribado y procesamiento de imágenes en torno a 2018 estimuló la inversión en este campo, los análisis de marketing predicen un aumento similar de la inversión en los próximos años, a medida que se generalicen las herramientas de IA que proporcionan diagnósticos y pasos de gestión específicos (Michoud et al., 2019).
Una crítica importante al panorama actual de la IA en el diagnóstico por la imagen, posiblemente aún incipiente, es que está demasiado fragmentada. Sin duda, los profesionales de radiología apreciarían una integración más eficiente de las soluciones de inteligencia artificial en su rutina diaria. Esto incluye la integración perfecta de estas soluciones en los flujos de trabajo de radiología establecidos, con el mayor número posible de procesos «en segundo plano», sin intervención del usuario. Además, los resultados de estas soluciones podrían integrarse en los sistemas de información radiológica disponibles. En consecuencia, el campo podría pasar de la gran cantidad de soluciones de IA de nicho disponibles actualmente, cada una dirigida a una única aplicación muy específica, a paquetes de software más amplios que abarquen diversas funciones para una modalidad de imagen o una región corporal determinada.
La fragmentación de la inversión en el mercado de la IA en el diagnóstico por la imagen (Alexander et al., 2020) fomenta la innovación, lo que permite a muchos actores probar diferentes estrategias en este campo emergente. Sin embargo, a largo plazo, la consolidación puede aumentar la adopción y estimular el tipo de integración perfecta que se necesita en los flujos de trabajo existentes, permitiendo a menos empresas ofrecer estas soluciones a escala (Alexander et al., 2020).
Desafíos
Calidad y presentación de los datos
En una revisión de 100 soluciones de IA con marcado CE, el 64 % de ellas carecían de pruebas científicas con revisión científica externa sobre su eficacia (van Leeuwen et al., 2021). En los casos en los que se disponía de pruebas científicas, su nivel era bajo, y rara vez superaba la demostración de exactitud diagnóstica (van Leeuwen et al., 2021). Otra revisión sistemática de la evidencia de algoritmos de aprendizaje profundo en imágenes halló que la precisión diagnóstica era, por lo general, elevada, si bien se observó un alto riesgo de sesgo entre los estudios (Aggarwal et al., 2021). Las principales fuentes de sesgo son la falta de validación externa (D. W. Kim y otros, 2019; Liu et al., 2019), informes con resultados insuficientemente detallados (Liu et al., 2019), un diseño de estudio retrospectivo (Nagendran et al., 2020) y la inaccesibilidad de los datos y el código por parte los revisores y lectores (Nagendran et al., 2020).
En general, los estudios sobre herramientas de IA han mostrado una preocupante falta de informes estandarizados y de adhesión a las directrices de información recomendadas (Aggarwal et al., 2021; Yusuf et al., 2020). A pesar de que actualmente existen diversas ampliaciones de las directrices de notificación establecidas, así como directrices específicas para la IA (Shelmerdine et al., 2021). La aplicación generalizada de estas directrices debería ser uno de los objetivos de los desarrolladores de IA en el futuro.
Los desarrolladores de IA también deben ser conscientes de que es probable que el nivel de datos actualmente «aceptables» para las soluciones basadas en IA se quede obsoleto en un futuro próximo. Es probable que tanto los reguladores como los posibles usuarios exijan unos niveles de datos más sólidos para estas soluciones, similares a los requeridos para los nuevos fármacos. En los próximos años, veremos cómo se prueban más de estas soluciones de IA en ensayos clínicos aleatorizados. En un futuro más lejano, es plausible que tales expectativas vayan más allá de aportar pruebas de la seguridad, eficacia o rendimiento diagnóstico de estas soluciones, para llegar a demostrar que también aportan un valor monetario o social añadido.
Afrontar el reto de mejorar la calidad y la presentación de pruebas para las soluciones basadas en IA puede resultar rentable a largo plazo. Lo que podría reducir el riesgo de sesgo en los estudios de IA, permitir una evaluación exhaustiva y transparente de la calidad de los estudios por parte de los usuarios potenciales y los organismos reguladores, y facilitar las revisiones sistemáticas y los metanálisis. Estas acciones pueden fortalecer la confianza en las soluciones basadas en la inteligencia artificial y fomentar su adopción, a la vez que se garantiza que ofrezcan mejoras realistas y sostenibles en la vida de las personas.
Regulación
Existen varios aspectos inherentes a la IA que presentan retos en cuanto a los intentos de regularla como otras intervenciones sanitarias. El funcionamiento interno de las soluciones de IA suele ser opaco y difícil de describir de forma exhaustiva, tal como esperan tradicionalmente los organismos reguladores.
Sin embargo, los últimos años nos han demostrado que estos desafíos regulatorios están lejos de ser irresolubles. Tanto la Administración de Alimentos y Medicamentos de los EE. UU. como la Comisión Europea han propuesto recientemente marcos regulatorios iniciales para las soluciones de IA (Center for Devices & Radiological Health, 2021; European Commission, 2021).
En parte, como respuesta a la transparencia necesaria para la aprobación regulatoria, los investigadores han logrado avances significativos para hacer que la toma de decisiones de la IA sea más comprensible y explicable. Este movimiento hacia una «IA interpretable» cobrará un mayor impulso en un futuro próximo, a medida que aumente la dependencia de la IA para la toma de decisiones clínicas en la vida real.
Ello ofrece múltiples ventajas, como, por ejemplo, facilitar la aprobación regulatoria, aumentar la confianza de los usuarios en estas soluciones, reducir los sesgos y mejorar la reproducibilidad de estas soluciones (Holzinger et al., 2017; Kolyshkina & Simoff, 2021; “Towards Trustable Machine Learning”, 2018; Yoon et al., 2021).
Privacidad de los datos
Desde el desarrollo y las pruebas hasta la implantación, las soluciones de IA en el diagnóstico por la imagen requieren acceder a los datos de los pacientes. Esto ha generado preocupación en relación a la privacidad de los datos, una problemática polifacética y sumamente compleja (Murdoch, 2021), que ocupa un lugar destacado en las estrategias regulatorias de diversos países (COCIR, Comité Europeo de Coordinación de la Industria Radiológica, Electromédica y de las Tecnologías de la Información Sanitaria, 2020). Las soluciones sugeridas a este respecto van desde las centradas en la supervisión hasta enfoques más técnicos.
Los pacientes que faciliten los datos tienen que ser conscientes de que lo están haciendo, así como ser informados de por qué y cómo se utilizarán sus datos (Lotan et al., 2020), tal y como se estipula explícitamente en el Reglamento General de Protección de Datos de la UE (Reglamento General de Protección de Datos [RGPD]– Texto Legal Oficial, 2016). Teniendo en cuenta la rapidez con la que se desarrollan las soluciones de IA, se ha cuestionado si es posible mantener a los pacientes suficientemente informados a medida que estos algoritmos se actualizan (Kritikos, 2020). Aunque los datos totalmente anonimizados no están sujetos a requisitos tan estrictos en virtud del RGPD (¿Qué son los datos personales?, 2021), la anonimización es sumamente difícil de lograr en los datos de imágenes médicas.
La privacidad de los datos deberá abordarse desde varios frentes. Además de la legislación que regula el uso de los datos de los pacientes, cada vez está más claro que todas las partes implicadas en el desarrollo y el uso de soluciones de IA -desarrolladores, seguros médicos, organismos reguladores, investigadores y radiólogos- tienen un papel que desempeñar para garantizar que los datos estén protegidos y se utilicen de forma responsable.
Asimismo, es probable que en los próximos años se sigan investigando enfoques técnicos para reforzar la protección de datos. Entre ellos, se incluyen la mejora de los métodos para reducir las posibilidades de que se pueda rastrear a la persona a partir de sus datos, métodos para mantener los datos sensibles almacenados a nivel local, incluso cuando el algoritmo que se está entrenando se aloja en alguna ubicación «central», la alteración de los datos para minimizar la información dentro de un conjunto de datos dado que pertenezca a pacientes individuales, y el cifrado de datos (G. Kaissis et al., 2021; G. A. Kaissis et al., 2020).
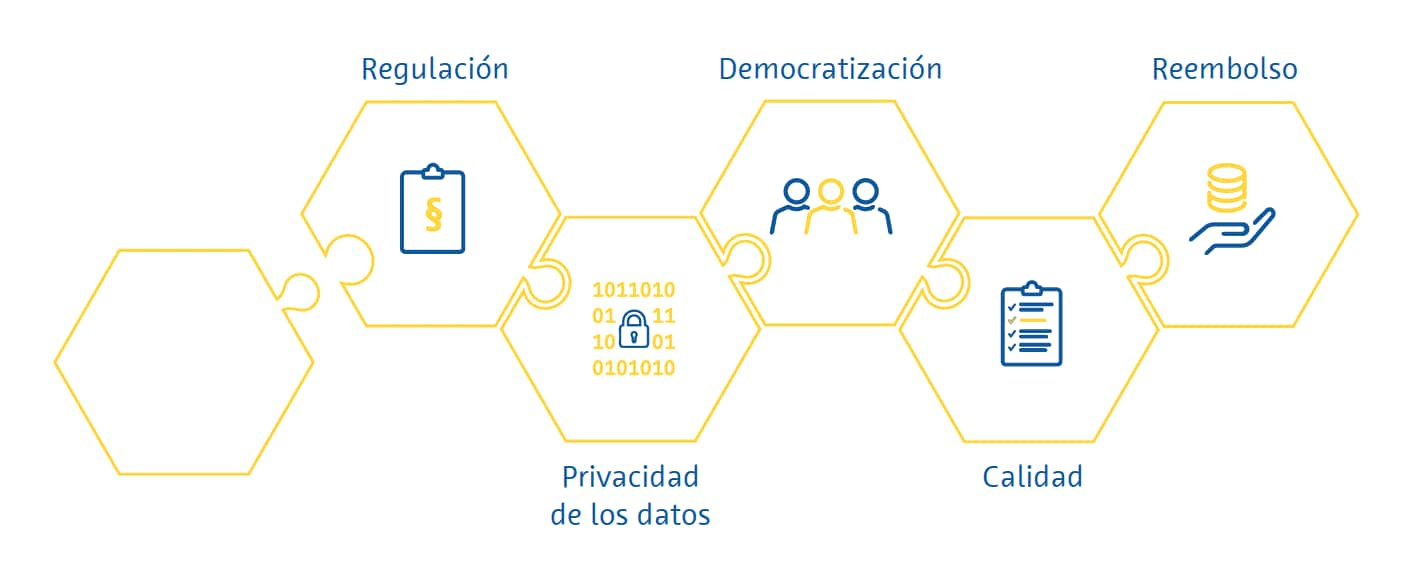
Democratización
A fin de que el uso de la IA en el diagnóstico por la imagen logre alcanzar todo su potencial, los algoritmos que se están desarrollando deben poder ser utilizados por todo el mundo. Esta «democratización» de la IA implica garantizar que los profesionales sanitarios dispongan de los conocimientos y habilidades necesarios para utilizar soluciones basadas en la IA. Salvo algunas excepciones (Paranjape et al., 2019), actualmente, los planes de estudio de los estudiantes de medicina incluyen poca o ninguna formación específica sobre IA (Banerjee et al., 2021; Blease et al., 2022). Encuestas llevadas a cabo en todo el mundo han demostrado que la exposición de los estudiantes de medicina y los médicos (Ahmed et al., 2022; Bisdas et al., 2021; Collado-Mesa et al., 2018; Kansal et al., 2022; Pinto Dos Santos et al., 2019; Sit et al., 2020) a la IA durante la formación fue baja, a pesar de la gran demanda de acceder a contenidos formativos sobre esta temática (Kansal et al., 2022; Ooi et al., 2021; Sit et al., 2020). Además, sigue habiendo grandes diferencias entre géneros y países en cuanto a los conocimientos percibidos sobre la IA entre los estudiantes de medicina (Bisdas et al., 2021). Son muchas las razones que dan lugar a estas diferencias, y muchos los desafíos asociados a la integración generalizada de la educación en IA en los planes de estudios de los profesionales sanitarios. En los próximos años, habrá que investigar estrategias para abordar estos problemas y garantizar que los futuros profesionales sanitarios dispongan de los conocimientos y habilidades que necesitan para trabajar en un entorno en el que la IA desempeña un papel cada vez más importante.
La democratización también implica garantizar que pacientes de distintos géneros, estilos de vida, etnias y ubicaciones geográficas puedan beneficiarse de las soluciones basadas en la IA. Para que esto suceda, estas soluciones deben ser accesibles y su rendimiento generalizable. Esto último requiere la adquisición de datos diversos de múltiples instituciones, preferiblemente de múltiples países, para entrenar soluciones basadas en IA. Asimismo, además de la legislación que regula el uso de los datos de los pacientes, también es crucial implementar medidas preventivas para asegurar que las fuentes de sesgo a lo largo del proceso de desarrollo no se propaguen al algoritmo entrenado (Vokinger et al., 2021), una cuestión que solo recientemente ha pasado a primer plano (Larrazabal et al., 2020; Obermeyer et al., 2019; Seyyed-Kalantari et al., 2021).
Reembolso
A medida que las políticas de los países para regular la IA en la atención sanitaria empiezan a tomar forma, un aspecto importante que requiere atención es quién pagará estas soluciones de IA, y según qué marco.
Muchos consideran que la Ley de Suministro Digital de 2020 de Alemania es un paso en la dirección correcta para el reembolso de las soluciones de salud digitales. Según esta política, las aplicaciones digitales prescritas por los médicos son reembolsables por los seguros médicos obligatorios si se demuestra que son seguras, cumplen las leyes de protección de datos y mejoran la atención al paciente. El Reino Unido, por su parte, ha publicado una guía para posibles compradores de soluciones basadas en IA, que sirve de punto de partida para que las empresas se preparen para solicitar el reembolso (A Buyer's Guide to AI in Health and Care, 2020).
Hasta ahora, los casos de éxito en el reembolso en el ámbito de la sanidad digital han sido escasos (Brinkmann- Sass et al., 2020; Hassan, 2021). Esto se debe en parte a que los requisitos varían mucho de un país a otro (COCIR, Comité Europeo de Coordinación de la Industria Radiológica, Electromédica y de las Tecnologías de la Información Sanitaria, 2020). En general, los proveedores de soluciones sanitarias digitales deberán presentar evidencias del valor global que ofrecen estas soluciones, incluyendo estudios detallados de economía sanitaria que demuestren el posible ahorro de costes.
La posición de la Unidad de Radiología como proveedor de servicios para múltiples unidades hospitalarias implica que se espera que las soluciones basadas en IA en este ámbito tengan un impacto significativo (van Duffelen, 2021). Las empresas deberán demostrar su valor tanto a corto plazo (mediante mejoras en la velocidad de lectura e informes de imágenes) como a largo plazo (mediante diagnósticos y tratamientos tempranos, prevención de enfermedades y reducción de seguimientos innecesarios). En los próximos años, las empresas competirán por demostrar su impacto, experimentando con distintos modelos de precios y sorteando el cambiante panorama burocrático de los reembolsos.
Conclusión
En los últimos años, el campo de la IA en el diagnóstico por la imagen ha experimentado una transformación rápida pero constante. En la actualidad, la IA en radiología puede lograr cosas que pocos creían posibles hace apenas una década. Este campo también está superando gradualmente uno de los obstáculos más importantes: la aprobación regulatoria. Además, si bien hace unos años el miedo y el escepticismo dominaban la percepción de los radiólogos sobre el futuro de la IA en su especialidad, ya no es así.
Se espera que el enorme progreso y el interés en el campo de la IA en el diagnóstico por la imagen se mantenga a lo largo de 2022, y más allá. En los próximos años, este campo experimentará transformaciones apasionantes. Es probable que amplíe su ámbito de acción para mejorar la eficiencia del flujo de trabajo radiológico, incluir modalidades de imagen hasta ahora desatendidas, combinar datos de múltiples modalidades y ofrecer predicciones diagnósticas y recomendaciones más específicas sobre los tratamientos. Se incorporarán paquetes de software completos y fáciles de usar que utilizan IA en los flujos de trabajo de radiología existentes, lo que hará que el trabajo de los radiólogos y radiógrafos sea más fácil y eficiente.
Como en cualquier campo de rápido crecimiento, el uso de la IA en el diagnóstico por la imagen debe hacer frente a varios desafíos científicos, regulatorios y económicos. Sin embargo, los últimos años nos han demostrado que incluso los problemas más difíciles pueden resolverse. Los desarrolladores y usuarios de soluciones basadas en IA deben ser conscientes de estas cuestiones para poder adaptar sus estrategias a las expectativas cambiantes a nivel regulatorio y social. Ello les permitirá prosperar en un campo fascinante, con el potencial de mejorar prácticamente todos los aspectos de la atención médica.
Referencias bibliográficas
AAMC Report Reinforces Mounting Physician Shortage. (2021). AAMC. https://www.aamc.org/news-insights/press- releases/aamc-report-reinforces-mounting-physician-shortage
A buyer’s guide to AI in health and care. (2020). NHS Transformation Directorate. https://www.nhsx.nhs.uk/ai-lab/ explore-all-resources/adopt-ai/a-buyers-guide-to-ai-in-health- and-care/
Aggarwal, R., Sounderajah, V., Martin, G., Ting, D. S. W., Karthikesalingam, A., King, D., Ashrafian, H., & Darzi, A. (2021). Diagnostic accuracy of deep learning in medical imaging: a systematic review and meta-analysis. NPJ Digital Medicine, 4(1), 65.
Ahmed, Z., Bhinder, K. K., Tariq, A., Tahir, M. J., Mehmood, Q., Tabassum, M. S., Malik, M., Aslam, S., Asghar, M. S., & Yousaf, Z. (2022). Knowledge, attitude, and practice of artificial intelligence among doctors and medical students in Pakistan: A cross-sectional online survey. Annals of Medicine and Surgery (2012), 76, 103493.
AI Central. (n.d.). Retrieved February 23, 2022, from https://aicentral.acrdsi.org/
AI for Radiology. (n.d.). Retrieved February 23, 2022, from https://grand-challenge.org/aiforradiology/
Alexander, A., Jiang, A., Ferreira, C., & Zurkiya, D. (2020). An Intelligent Future for Medical Imaging: A Market Outlook on Artificial Intelligence for Medical Imaging. Journal of the American College of Radiology: JACR, 17(1 Pt B), 165–170.
Allen, B., Agarwal, S., Coombs, L., Wald, C., & Dreyer, K. (2021). 2020 ACR Data Science Institute Artificial Intelligence Survey. Journal of the American College of Radiology: JACR, 18(8), 1153–1159.
Banerjee, M., Chiew, D., Patel, K. T., Johns, I., Chappell, D., Linton, N., Cole, G. D., Francis, D. P., Szram, J., Ross, J., & Zaman, S. (2021). The impact of artificial intelligence on clinical education: perceptions of postgraduate trainee doctors in London (UK) and recommendations for trainers. BMC Medical Education, 21(1), 429.
Bash, S., Johnson, B., Gibbs, W., Zhang, T., Shankaranarayanan, A., & Tanenbaum, L. N. (2021). Deep Learning Image Processing Enables 40 % Faster Spinal MR Scans Which Match or Exceed Quality of Standard of Care : A Prospective Multicenter Multireader Study. Clinical Neuroradiology. https://doi.org/10.1007/s00062-021-01121-2
Bash, S., Wang, L., Airriess, C., Zaharchuk, G., Gong, E., Shankaranarayanan, A., & Tanenbaum, L. N. (2021). Deep Learning Enables 60 % Accelerated Volumetric Brain MRI While Preserving Quantitative Performance: A Prospective, Multicenter, Multireader Trial. AJNR. American Journal of Neuroradiology, 42(12), 2130–2137.
Bennett, C. C., & Hauser, K. (2013). Artificial intelligence framework for simulating clinical decision-making: a Markov decision process approach. Artificial Intelligence in Medicine, 57(1), 9–19.
Bisdas, S., Topriceanu, C.-C., Zakrzewska, Z., Irimia, A.-V., Shakallis, L., Subhash, J., Casapu, M.-M., Leon-Rojas, J., Pinto Dos Santos, D., Andrews, D. M., Zeicu, C., Bouhuwaish, A. M., Lestari, A. N., Abu-Ismail, L. ’i, Sadiq, A. S., Khamees, A. ’atasim, Mohammed, K. M. G., Williams, E., Omran, A. I.,… Ebrahim, E. H. (2021). Artificial Intelligence in Medicine: A Multinational Multi-Center Survey on the Medical and Dental Students’ Perception. Frontiers in Public Health, 9, 795284.
Blease, C., Kharko, A., Bernstein, M., Bradley, C., Houston, M., Walsh, I., Hägglund, M., DesRoches, C., & Mandl, K. D. (2022). Machine learning in medical education: a survey of the experiences and opinions of medical students in Ireland. BMJ Health & Care Informatics, 29(1). https://doi.org/10.1136/ bmjhci-2021-100480
Brown, A. D., & Marotta, T. R. (2018). Using machine learning for sequence-level automated MRI protocol selection in neuroradiology. Journal of the American Medical Informatics Association: JAMIA, 25(5), 568–571.
Center for Devices, & Radiological Health. (2021, June 22). Digital Health Software Precertification (Pre-Cert) program.
U.S. Food and Drug Administration. https://www.fda.gov/ medical-devices/digital-health-center-excellence/digital-health- software-precertification-pre-cert-program
Clinical radiology UK workforce census 2019 report. (2019). https://www.rcr.ac.uk/publication/clinical-radiology-uk- workforce-census-2019-report
COCIR, the European Coordination Committee of the Radiological, Electromedical and Healthcare IT Industry. (2020). Market Access Pathways for Digital Health Solutions. https://www.cocir.org/fileadmin/Publications_2020/20062_ COCIR_Market_Access_Pathways_Digital_Health.pdf
Collado-Mesa, F., Alvarez, E., & Arheart, K. (2018). The Role of Artificial Intelligence in Diagnostic Radiology: A Survey at a Single Radiology Residency Training Program. Journal of the American College of Radiology: JACR, 15(12), 1753–1757.
Core Health Indicators in the WHO European Region 2015. Special focus: Human resources for health. (2017, August 14). World Health Organization. https://www.euro.who.int/en/ data-and-evidence/evidence-resources/core-health-indicators- in-the-who-european-region/core-health-indicators-in-the- who-european-region-2015.-special-focus-human-resources- for-health
Dantas, L. F., Fleck, J. L., Cyrino Oliveira, F. L., & Hamacher, S. (2018). No-shows in appointment scheduling - a systematic literature review. Health Policy, 122(4), 412–421.
Esses, S. J., Lu, X., Zhao, T., Shanbhogue, K., Dane, B., Bruno, M., & Chandarana, H. (2018). Automated image quality evaluation of T2 -weighted liver MRI utilizing deep learning architecture. Journal of Magnetic Resonance Imaging: JMRI, 47(3), 723–728.
European Commission. (2021). Proposal for a Regulation Of The European Parliament And Of The Council Laying Down
Harmonised Rules On Artificial Intelligence (Artificial Intelligence Act) And Amending Certain Union Legislative Act. https://eur-lex. europa.eu/resource.html?uri=cellar:e0649735-a372-11eb- 9585-01aa75ed71a1.0001.02/DOC_1&format=PDF
General Data Protection Regulation (GDPR) – Official Legal Text. (2016, July 13). General Data Protection Regulation (GDPR). https://gdpr-info.eu/
Hata, A., Yanagawa, M., Yoshida, Y., Miyata, T., Tsubamoto, M., Honda, O., & Tomiyama, N. (2020). Combination of Deep Learning-Based Denoising and Iterative Reconstruction for Ultra-Low-Dose CT of the Chest: Image Quality and Lung-RADS Evaluation. AJR. American Journal of Roentgenology, 215(6), 1321–1328.
Hauptmann, A., Arridge, S., Lucka, F., Muthurangu, V., & Steeden, J. A. (2019). Real-time cardiovascular MR with
spatio-temporal artifact suppression using deep learning-proof of concept in congenital heart disease. Magnetic Resonance in Medicine: Official Journal of the Society of Magnetic Resonance in Medicine / Society of Magnetic Resonance in Medicine, 81(2), 1143–1156.
Higaki, T., Nakamura, Y., Zhou, J., Yu, Z., Nemoto, T., Tatsugami, F., & Awai, K. (2020). Deep Learning Reconstruction at CT: Phantom Study of the Image Characteristics. Academic Radiology, 27(1), 82–87.
Holzinger, A., Biemann, C., Pattichis, C. S., & Kell, D. B. (2017). What do we need to build explainable AI systems for the medical domain? In arXiv [cs.AI]. arXiv. http://arxiv.org/ abs/1712.09923
Hötker, A. M., Da Mutten, R., Tiessen, A., Konukoglu, E., & Donati, O. F. (2021). Improving workflow in prostate MRI: AI- based decision-making on biparametric or multiparametric MRI. Insights into Imaging, 12(1), 112.
Huang, S.-C., Pareek, A., Seyyedi, S., Banerjee, I., & Lungren, M. P. (2020). Fusion of medical imaging and electronic health records using deep learning: a systematic review and implementation guidelines. NPJ Digital Medicine, 3, 136.
Kaissis, G. A., Makowski, M. R., Rückert, D., & Braren, R. F. (2020). Secure, privacy-preserving and federated machine learning in medical imaging. Nature Machine Intelligence, 2(6), 305–311.
Kaissis, G., Ziller, A., Passerat-Palmbach, J., Ryffel, T., Usynin, D., Trask, A., Lima, I., Mancuso, J., Jungmann, F., Steinborn, M.-M., Saleh, A., Makowski, M., Rueckert, D., & Braren, R. (2021). End-to-end privacy preserving deep learning on multi-institutional medical imaging. Nature Machine Intelligence, 3(6), 473–484.
Kansal, R., Bawa, A., Bansal, A., Trehan, S., Goyal, K., Goyal, N., & Malhotra, K. (2022). Differences in Knowledge and Perspectives on the Usage of Artificial Intelligence Among Doctors and Medical Students of a Developing Country: A Cross- Sectional Study. Cureus, 14(1), e21434.
Katsari, K., Penna, D., Arena, V., Polverari, G., Ianniello, A., Italiano, D., Milani, R., Roncacci, A., Illing, R. O., & Pelosi, E. (2021). Artificial intelligence for reduced dose 18F-FDG PET examinations: a real-world deployment through a standardized framework and business case assessment. EJNMMI Physics, 8(1), 25.
Kerpel, A., Marom, E. M., Green, M., Eifer, M., Konen, E., Mayer, A., & Betancourt Cuellar, S. L. (2021). Ultra-Low Dose Chest CT with Denoising for Lung Nodule Detection. The Israel Medical Association Journal: IMAJ, 23(9), 550–555.
Kim, D. W., Jang, H. Y., Kim, K. W., Shin, Y., & Park, S. H. (2019).
Design Characteristics of Studies Reporting the Performance of Artificial Intelligence Algorithms for Diagnostic Analysis of
Medical Images: Results from Recently Published Papers. Korean Journal of Radiology: Official Journal of the Korean Radiological Society, 20(3), 405–410.
Kim, K. H., & Park, S.-H. (2017). Artificial neural network for suppression of banding artifacts in balanced steady-state free precession MRI. Magnetic Resonance Imaging, 37, 139–146.
Kolyshkina, I., & Simoff, S. (2021). Interpretability of Machine Learning Solutions in Public Healthcare: The CRISP-ML Approach. Frontiers in Big Data, 4, 660206.
Komorowski, M., Celi, L. A., Badawi, O., Gordon, A. C., & Faisal, A. A. (2018). The Artificial Intelligence Clinician learns optimal treatment strategies for sepsis in intensive care. Nature Medicine, 24(11), 1716–1720.
Kritikos, M. (2020). What if artificial intelligence in medical imaging could accelerate Covid-19 treatment? Think Tank
- European Parliament. https://www.europarl.europa.eu/ thinktank/en/document/EPRS_ATA(2020)656333
Kurasawa, H., Hayashi, K., Fujino, A., Takasugi, K., Haga, T., Waki, K., Noguchi, T., & Ohe, K. (2016). Machine-Learning- Based Prediction of a Missed Scheduled Clinical Appointment by Patients With Diabetes. Journal of Diabetes Science and Technology, 10(3), 730–736.
Larrazabal, A. J., Nieto, N., Peterson, V., Milone, D. H., & Ferrante, E. (2020). Gender imbalance in medical imaging datasets produces biased classifiers for computer-aided diagnosis. Proceedings of the National Academy of Sciences of the United States of America, 117(23), 12592–12594.
Larson, D. B., Johnson, L. W., Schnell, B. M., Salisbury, S. R., & Forman, H. P. (2011). National trends in CT use in the emergency department: 1995-2007. Radiology, 258(1), 164–173.
Le, V., Frye, S., Botkin, C., Christopher, K., Gulaka, P., Sterkel, B., Frye, R., Muzaffar, R., & Osman, M. (2020). Effect of PET Scan with Count Reduction Using AI-Based Processing Techniques on Image Quality. Journal of Nuclear Medicine: Official Publication, Society of Nuclear Medicine, 61(supplement 1), 3095–3095.
Levin, D. C., Parker, L., & Rao, V. M. (2017). Recent Trends in Imaging Use in Hospital Settings: Implications for Future Planning. Journal of the American College of Radiology: JACR, 14(3), 331–336.
Liu, X., Faes, L., Kale, A. U., Wagner, S. K., Fu, D. J., Bruynseels, A., Mahendiran, T., Moraes, G., Shamdas, M., Kern, C., Ledsam, J. R., Schmid, M. K., Balaskas, K., Topol, E.J., Bachmann, L. M., Keane, P. A., & Denniston, A. K. (2019). A comparison of deep learning performance against health-care professionals in detecting diseases from medical imaging: a systematic review and meta-analysis. The Lancet. Digital Health, 1(6), e271–e297.
Lotan, E., Tschider, C., Sodickson, D. K., Caplan, A. L., Bruno, M., Zhang, B., & Lui, Y. W. (2020). Medical Imaging and Privacy in the Era of Artificial Intelligence: Myth, Fallacy, and the Future. Journal of the American College of Radiology: JACR, 17(9), 1159–1162.
Mairhöfer, D., Laufer, M., Simon, P. M., Sieren, M., Bischof, A., Käster, T., Barth, E., Barkhausen, J., & Martinetz, T. (2021). An AI-based Framework for Diagnostic Quality Assessment of Ankle Radiographs. https://openreview.net/pdf?id=bj04hJss_xZ
Makeeva, V., Gichoya, J., Hawkins, C. M., Towbin, A. J., Heilbrun, M., & Prater, A. (2019). The Application of Machine Learning to Quality Improvement Through the Lens of the Radiology Value Network. Journal of the American College of Radiology: JACR, 16(9 Pt B), 1254–1258.
McLeavy, C. M., Chunara, M. H., Gravell, R. J., Rauf, A., Cushnie, A., Staley Talbot, C., & Hawkins, R. M. (2021). The future of CT: deep learning reconstruction. Clinical Radiology, 76(6), 407–415.
Medical AI Evaluation. (n.d.). Retrieved February 23, 2022, from https://ericwu09.github.io/medical-ai-evaluation/
Michoud, L., Tschudi, Y., & Villien, Y. (2019). Artificial Intelligence for Medical Imaging: Market and Technology Report 2020. Yole Développement. https://s3.i-micronews.com/ uploads/2020/01/YDR20059-AI-for-Medical-Imaging_Yole_ sample.pdf
Murdoch, B. (2021). Privacy and artificial intelligence: challenges for protecting health information in a new era. BMC Medical Ethics, 22(1), 122.
Nagendran, M., Chen, Y., Lovejoy, C. A., Gordon, A. C., Komorowski, M., Harvey, H., Topol, E. J., Ioannidis, J. P. A., Collins, G. S., & Maruthappu, M. (2020). Artificial intelligence versus clinicians: systematic review of design, reporting standards, and claims of deep learning studies. BMJ, 368. https://doi.org/10.1136/bmj.m689
Nelson, A., Herron, D., Rees, G., & Nachev, P. (2019). Predicting scheduled hospital attendance with artificial intelligence. Npj Digital Medicine, 2(1), 26.
Obermeyer, Z., Powers, B., Vogeli, C., & Mullainathan, S. (2019). Dissecting racial bias in an algorithm used to manage the health of populations. Science, 366(6464), 447–453.
Ooi, S. K. G., Makmur, A., Soon, A. Y. Q., Fook-Chong, S., Liew, C., Sia, S. Y., Ting, Y. H., & Lim, C. Y. (2021). Attitudes toward artificial intelligence in radiology with learner needs assessment within radiology residency programmes: a national multi-programme survey. Singapore Medical Journal, 62(3), 126–134.
Paranjape, K., Schinkel, M., Nannan Panday, R., Car, J., & Nanayakkara, P. (2019). Introducing Artificial Intelligence Training in Medical Education. JMIR Medical Education, 5(2), e16048.
Park, J., Hwang, D., Kim, K. Y., Kang, S. K., Kim, Y. K., & Lee, J. S. (2018). Computed tomography super-resolution using deep convolutional neural network. Physics in Medicine and Biology, 63(14), 145011.
Pinto Dos Santos, D., Giese, D., Brodehl, S., Chon, S. H., Staab, W., Kleinert, R., Maintz, D., & Baeßler, B. (2019). Medical students’ attitude towards artificial intelligence: a multicentre survey. European Radiology, 29(4), 1640–1646.
Population ages 65 and above. (n.d.). The World Bank. Retrieved February 23, 2022, from https://data.worldbank.org/ indicator/SP.POP.65UP.TO.ZS
Prevedello, L. M., Erdal, B. S., Ryu, J. L., Little, K. J., Demirer, M., Qian, S., & White, R. D. (2017). Automated Critical Test Findings Identification and Online Notification System Using Artificial Intelligence in Imaging. Radiology, 285(3), 923–931.
Rezazade Mehrizi, M. H., van Ooijen, P., & Homan, M. (2021). Applications of artificial intelligence (AI) in diagnostic radiology: a technography study. European Radiology, 31(4), 1805–1811.
Rockenbach, M. A. B. (2021, June 13). Multimodal AI in healthcare: Closing the gaps. CodeX. https://medium.com/codex/ multimodal-ai-in-healthcare-1f5152e83be2
Rudie, J. D., Gleason, T., Barkovich, M. J., Wilson, D. M., Shankaranarayanan, A., Zhang, T., Wang, L., Gong, E., Zaharchuk, G., & Villanueva-Meyer, J. E. (2022). Clinical Assessment of Deep Learning–based Super-Resolution for 3D Volumetric Brain MRI. Radiology: Artificial Intelligence, e210059.
Schreiber-Zinaman, J., & Rosenkrantz, A. B. (2017). Frequency and reasons for extra sequences in clinical abdominal MRI examinations. Abdominal Radiology (New York), 42(1), 306–311.
Seyyed-Kalantari, L., Zhang, H., McDermott, M. B. A., Chen, I. Y., & Ghassemi, M. (2021). Underdiagnosis bias of artificial intelligence algorithms applied to chest radiographs in under-served patient populations. Nature Medicine, 27(12), 2176–2182.
Shelmerdine, S. C., Arthurs, O. J., Denniston, A., & Sebire, N. J. (2021). Review of study reporting guidelines for clinical studies using artificial intelligence in healthcare. BMJ Health & Care Informatics, 28(1). https://doi.org/10.1136/ bmjhci-2021-100385
Shinagare, A. B., Ip, I. K., Abbett, S. K., Hanson, R., Seltzer, S. E., & Khorasani, R. (2014). Inpatient imaging utilization: trends of the past decade. AJR. American Journal of Roentgenology, 202(3), W277–W283.
Singh, R., Digumarthy, S. R., Muse, V. V., Kambadakone, A. R., Blake, M. A., Tabari, A., Hoi, Y., Akino, N., Angel, E., Madan, R., & Kalra, M. K. (2020). Image Quality and Lesion Detection on Deep Learning Reconstruction and Iterative Reconstruction of Submillisievert Chest and Abdominal CT. AJR. American Journal of Roentgenology, 214(3), 566–573.
Sit, C., Srinivasan, R., Amlani, A., Muthuswamy, K., Azam, A., Monzon, L., & Poon, D. S. (2020). Attitudes and perceptions of UK medical students towards artificial intelligence and radiology: a multicentre survey. Insights into Imaging, 11(1), 14.
Smith-Bindman, R., Miglioretti, D. L., Johnson, E., Lee, C., Feigelson, H. S., Flynn, M., Greenlee, R. T., Kruger, R. L., Hornbrook, M. C., Roblin, D., Solberg, L. I., Vanneman, N., Weinmann, S., & Williams, A. E. (2012). Use of diagnostic imaging studies and associated radiation exposure for patients enrolled in large integrated health care systems, 1996-2010. JAMA: The Journal of the American Medical Association, 307(22), 2400–2409.
Smith-Bindman, R., Miglioretti, D. L., & Larson, E. B. (2008). Rising use of diagnostic medical imaging in a large integrated health system. Health Affairs, 27(6), 1491–1502.
The Medical Futurist. (n.d.). The Medical Futurist. Retrieved February 23, 2022, from https://medicalfuturist.com/fda- approved-ai-based-algorithms/
Towards trustable machine learning. (2018). Nature Biomedical Engineering, 2(10), 709–710.
Trivedi, H., Mesterhazy, J., Laguna, B., Vu, T., & Sohn, J. H. (2018). Automatic Determination of the Need for Intravenous Contrast in Musculoskeletal MRI Examinations Using IBM Watson’s Natural Language Processing Algorithm. Journal of Digital Imaging, 31(2), 245–251.
Tsao, D. N. (2020, July 27). AI in medical diagnostics 2020- 2030: Image recognition, players, clinical applications, forecasts: IDTechEx. https://www.idtechex.com/en/research- report/ai-in-medical-diagnostics-2020-2030-image- recognition-players-clinical-applications-forecasts/766
van Duffelen, J. (2021, February 22). Making a case for buying medical imaging AI: How to define the return on investment.
Aidence. https://www.aidence.com/articles/medical-imaging- ai-roi/
van Leeuwen, K. G., Schalekamp, S., Rutten, M. J. C. M., van Ginneken, B., & de Rooij, M. (2021). Artificial intelligence in radiology: 100 commercially available products and their scientific evidence. European Radiology, 31(6), 3797–3804.
Vokinger, K. N., Feuerriegel, S., & Kesselheim, A. S. (2021). Mitigating bias in machine learning for medicine. Communication & Medicine, 1, 25.
Wang, S., Cao, G., Wang, Y., Liao, S., Wang, Q., Shi, J., Li, C., & Shen, D. (2021). Review and Prospect: Artificial Intelligence in Advanced Medical Imaging. Frontiers in Radiology, 1. https://doi.org/10.3389/fradi.2021.781868
What is personal data? (2021, January 1). ICO - Information Commissioner’s Office; ICO. https://ico.org.uk/for-organisations/ guide-to-data-protection/guide-to-the-general-data-protection- regulation-gdpr/what-is-personal-data/what-is-personal-data
WHO. (n.d.-a). Ageing and health. Retrieved February 23, 2022, from https://www.who.int/news-room/fact-sheets/detail/ ageing-and-health
WHO. (n.d.-b). Noncommunicable diseases. Retrieved March 6, 2022, from https://www.who.int/news-room/fact-sheets/detail/ noncommunicable-diseases
Winder, M., Owczarek, A. J., Chudek, J., Pilch-Kowalczyk, J., & Baron, J. (2021). Are We Overdoing It? Changes in Diagnostic Imaging Workload during the Years 2010-2020 including the Impact of the SARS-CoV-2 Pandemic. Healthcare (Basel, Switzerland), 9(11). https://doi.org/10.3390/healthcare9111557
Winkel, D. J., Heye, T., Weikert, T. J., Boll, D. T., & Stieltjes, B. (2019). Evaluation of an AI-Based Detection Software for Acute Findings in Abdominal Computed Tomography Scans: Toward an Automated Work List Prioritization of Routine CT Examinations. Investigative Radiology, 54(1), 55–59.
Wong, T. T., Kazam, J. K., & Rasiej, M. J. (2019). Effect of Analytics-Driven Worklists on Musculoskeletal MRI
Interpretation Times in an Academic Setting. AJR. American Journal of Roentgenology, 1–5.
Xu, F., Pan, B., Zhu, X., Gulaka, P., Xiang, L., Gong, E., Zhang, T., Wang, J., Lin, L., Ma, Y., & Gong, N.-J. (2020). Evaluation of Deep Learning Based PET Image Enhancement Method in Diagnosis of Lymphoma. Journal of Nuclear Medicine: Official Publication, Society of Nuclear Medicine, 61(supplement 1), 431–431.
Yoon, C. H., Torrance, R., & Scheinerman, N. (2021). Machine learning in medicine: should the pursuit of enhanced interpretability be abandoned? Journal of Medical Ethics. https://doi.org/10.1136/medethics-2020-107102
Yusuf, M., Atal, I., Li, J., Smith, P., Ravaud, P., Fergie, M., Callaghan, M., & Selfe, J. (2020). Reporting quality of studies using machine learning models for medical diagnosis: a systematic review. BMJ Open, 10(3), e034568.
Zhang, D., Mishra, S., Brynjolfsson, E., Etchemendy, J., Ganguli, D., Grosz, B., Lyons, T., Manyika, J., Niebles, J. C., Sellitto, M., Shoham, Y., Clark, J., & Perrault, R. (2021). The AI Index 2021 Annual Report. In arXiv [cs.AI]. arXiv. http://arxiv.org/ abs/2103.06312
Zhang, Y., & Yu, H. (2018). Convolutional Neural Network Based Metal Artifact Reduction in X-Ray Computed Tomography. IEEE Transactions on Medical Imaging, 37(6), 1370–1381.
Zhu, B., Liu, J. Z., Cauley, S. F., Rosen, B. R., & Rosen, M. S. (2018). Image reconstruction by domain-transform manifold learning. Nature, 555(7697), 487–492.