Le cancer est l'une des principales causes de morbidité et de mortalité à l'échelle mondiale, avec une personne sur cinq en moyenne développant un cancer au cours de sa vie (The Burden of Cancer, n.d.). Le dépistage du cancer cible les individus asymptomatiques et vise à identifier soit un cancer à un stade précoce, soit des affections précancéreuses. Dans de nombreux cas, cela permet une intervention rapide et de meilleurs résultats du traitement. En général, le dépistage peut être considéré comme jouant un rôle préventif ou de détection précoce. Le dépistage préventif vise à détecter les affections bénignes pouvant devenir cancéreuses, ce qui n'est possible que pour certains cancers, tandis que les approches de détection précoce visent à détecter un cancer à un stade précoce. Il est important de noter que le dépistage ne doit pas être considéré comme un test unique, mais comme un processus qui comprend l'identification de la population cible, la réalisation de tests de diagnostic et la planification d'un examen plus approfondi, y compris un traitement si nécessaire (Organisation mondiale de la santé. Bureau régional pour l’Europe, 2022).
La radiologie joue depuis longtemps un rôle essentiel dans la détermination de l’étendue de la propagation locale et à distance des tumeurs après l’établissement d’un diagnostic de cancer. Cependant, elle est également indispensable dans le cadre du dépistage de plusieurs cancers courants. Dans ces cas, les examens d’imagerie médicale constituent soit le principal outil de dépistage, soit sont utilisées pour décider d’un examen plus approfondi après le dépistage à l’aide d’autres méthodes, telles que des analyses de sang. Selon le type de cancer, le dépistage peut faire appel à des techniques d'imagerie médicale telles que la mammographie, la tomodensitométrie (TDM), l'imagerie par résonance magnétique (IRM) ou l'échographie. Des programmes nationaux de dépistage utilisant l’imagerie médicale ont été mis en place pour certains des cancers les plus courants. La plupart de ces programmes ciblent des populations spécifiques à risque du cancer spécifique en question, identifiées à l'aide de facteurs de risque modifiables ou non modifiables.
Étant donné que le dépistage du cancer cible les personnes en bonne santé, il est particulièrement essentiel que les avantages d'un programme de dépistage l'emportent sur ses inconvénients. Cela doit être soigneusement établi pour chaque programme et est parfois controversé (Lam et al., 2014). Cependant, certains avantages et inconvénients du dépistage du cancer s'appliquent à toutes les techniques de dépistage et à tous les cancers (Kramer, 2004 ; Organisation mondiale de la santé. Bureau régional pour l’Europe, 2022). Le dépistage peut réduire les coûts des soins de santé et améliorer la qualité de vie des patients. Cela améliore également souvent le pronostic et les résultats du traitement des personnes diagnostiquées comme atteintes d'un cancer et peut rassurer les personnes chez lesquelles le cancer n'est pas détecté. Cependant, parfois, une détection précoce ne modifie pas le pronostic et, chez ces personnes, le dépistage peut donner lieu à un traitement inutile, entraînant une diminution de la santé ou de la qualité de vie. En fait, le dépistage permet parfois de détecter des cancers qui n'auraient jamais entraîné de problèmes de santé ou la mort d'une personne. De plus, les faux positifs et les faux négatifs sont inévitables avec tout test de dépistage. Les premiers conduisent à un surtraitement, avec les effets secondaires psychosociaux et physiques qui en résultent, et les deuxièmes à une fausse assurance et à un retard du traitement.
Cancer du sein
Le cancer du sein est la deuxième cause de décès par cancer chez les femmes (Bray et al., 2018) et l'un des cancers les plus courants dans le monde (Sung et al., 2021). La détection et le traitement précoces peuvent améliorer les résultats, et des études ont révélé une mortalité jusqu'à 20 % inférieure dans les populations dépistées par rapport aux populations auxquelles le dépistage n’est pas proposé. Des études estiment qu’un décès par cancer du sein est évité en moyenne pour 250 à 414 femmes dépistées (Marmot et al., 2013 ; Tabár et al., 2011). Plus de 100 pays dans le monde ont mis en œuvre des programmes de dépistage du cancer du sein à grande échelle (Existence of National Screening Program for Breast Cancer, n.d.). Le début du dépistage est recommandé entre 40 et 50 ans (Ren et al., 2022) et se fait presque exclusivement par mammographie, qui utilise des rayons X à faible dose pour obtenir une image des seins, ou par tomosynthèse mammaire numérique, une technique similaire qui utilise plusieurs projections pour créer une série d’images empilées du sein.
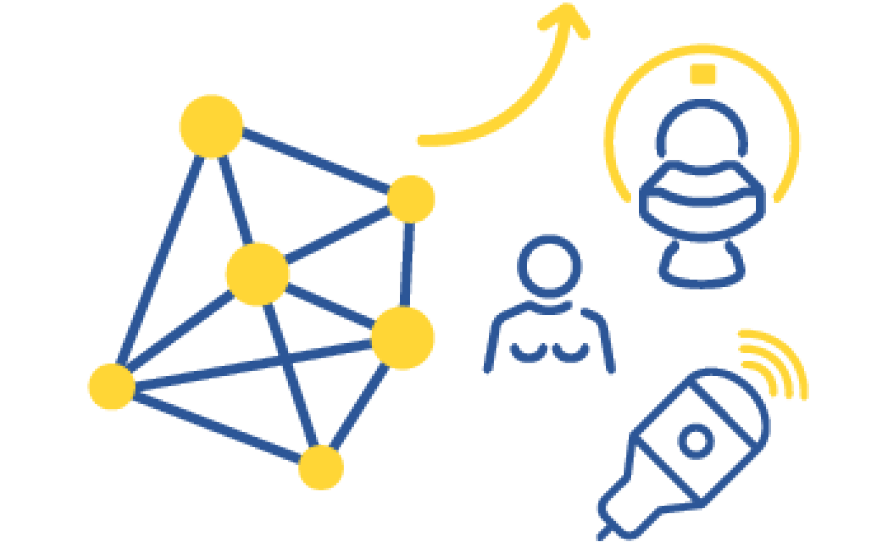
L’algorithme a augmenté la détection du cancer du sein de 12 à 27 % en triant les mammographies qui ont été évaluées comme négatives après une double lecture mais qui ont été considérées comme suspectes par l’algorithme pour une évaluation plus approfondie par IRM ou échographie.
La précision de la mammographie varie considérablement et même les lectures des radiologues les plus expérimentés présentent des taux élevés de faux positifs et de faux négatifs (Elmore et al., 2009 ; Lehman et al., 2015). On estime qu’au moins une femme dépistée sur trois aura un résultat faussement positif à la mammographie au cours de sa vie (Castells et al., 2006). La mammographie est particulièrement difficile dans le cas des seins denses (Boyd et al., 2007) et chez les femmes sous traitement hormonal substitutif (Banks et al., 2006). Le dépistage par mammographie est également un processus à forte intensité de main-d'oeuvre. Dans de nombreux pays européens, la norme de soins est la double lecture consensuelle, dans laquelle deux radiologues lisent consécutivement chaque cas et résolvent les désaccords par consensus (Giordano et al., 2012). Il existe malheureusement également une pénurie de radiologues et de manipulateurs en radiologie spécifiquement formés à la mammographie dans de nombreux pays (Moran & Warren-Forward, 2012 ; Rimmer, 2017 ; Wing & Langelier, 2009).
Des systèmes basés sur l’intelligence artificielle (IA) ont été intégrés à différentes étapes du processus de dépistage du cancer du sein. Dans une étude portant sur près de 30 000 femmes aux États-Unis et au Royaume-Uni ayant subi des mammographies de dépistage à des intervalles de 1 à 3 ans et une période de suivi allant jusqu'à 39 mois, un ensemble de trois modèles d'apprentissage profond a été comparé à l'histopathologie et aux interprétations de radiologues certifiés (McKinney et al., 2020). L'algorithme avait une spécificité 1,2 à 5,7 % plus élevée et une sensibilité 2,7 à 9,4 % plus élevée que celles des radiologues qui ont effectué la première lecture. Les auteurs ont estimé que l’utilisation de l’algorithme pourrait rendre inutiles les secondes lectures dans jusqu’à 88 % des cas de dépistage tout en maintenant la précision, libérant ainsi des ressources indispensables.
Des résultats toujours prometteurs ont été rapportés dans des études utilisant des systèmes basés sur l’IA en collaboration avec des radiologues. Une étude portant sur près de 16 000 femmes subissant une mammographie numérique ou une tomosynthèse mammaire numérique en Espagne a estimé que l'utilisation d'un algorithme basé sur l'apprentissage profond entraînerait une charge de travail inférieure de 72,5 % par rapport à la double lecture tout en maintenant la sensibilité (Raya-Povedano et al., 2021). Dans ce modèle, les examens les moins suspects seraient uniquement lus par l'algorithme et les 2 % d'examens les plus suspects, tels que jugés par l'algorithme, seraient signalés pour un examen plus approfondi, quelle que soit l'interprétation des radiologues. De même, une étude portant sur 7 354 femmes en Suède a révélé qu’un algorithme d’apprentissage profond disponible sur le marché classifiait avec précision les mammographies les moins suspectes, et que ces femmes n’avaient subi aucun examen supplémentaire (Dembrower et al., 2020). Ceci a été réalisé avec un taux de faux négatifs de 0 à 2,6 %. L'algorithme a également augmenté la détection du cancer du sein de 12 à 27 % en triant les mammographies qui ont été évaluées comme négatives après une double lecture, mais qui ont été considérées comme suspectes par l'algorithme pour une évaluation plus approfondie par IRM ou échographie.
D’autres études ont utilisé des systèmes basés sur l’IA comme étape d’orientation décisionnelle. Dans une étude portant sur plus d’un million de mammographies en Allemagne, un réseau neuronal convolutif profond (CNN) a attribué un score de confiance à chaque mammographie (Leibig et al., 2022). Les évaluations réalisées avec un niveau de confiance élevé par l'algorithme n'ont fait l'objet d'aucun traitement supplémentaire, tandis que les évaluations à faible niveau de confiance ont été renvoyées au radiologue. Cette approche était associée à une augmentation de 4 % de la sensibilité et de 0,5 % de la spécificité par rapport à l'évaluation d'un seul radiologue sans l'aide de l'algorithme. Dans ce scénario, 63 % des mammographies ont été automatiquement triées par l'algorithme, et l'amélioration des performances par rapport à la lecture d'un seul radiologue était cohérente sur huit sites de dépistage et trois fabricants de dispositifs.
Cancer du poumon
Le cancer du poumon est la principale cause de décès par cancer dans le monde, causant près de 1,8 million de décès en 2020 (Sung et al., 2021). Un exemple d’approche de dépistage ciblé, le dépistage du cancer du poumon, est recommandé en fonction du risque individuel. Le dépistage des adultes âgés de 50 à 80 ans ayant fumé pendant 20 paquets-années par tomodensitométrie à faible dose (LDCT) est recommandé aux États-Unis depuis 2013, après que des études initiales ont révélé une réduction relative de 20 % de la mortalité par la cancer du poumon (Lung Cancer: Screening, 2021 ; National Lung Screening Trial Research Team et al., 2011). Un programme de dépistage similaire est en cours de déploiement au Royaume-Uni (NHS England, 2022). Chez les patients qui qui bénéficient d’un dépistage du cancer du poumon par la LDCT, l’IA s’est révélée prometteuse pour la détection automatique des nodules pulmonaires susceptibles de représenter une tumeur maligne. Ceci est important car la détection des nodules pulmonaires par les radiologues est fastidieuse, prend du temps et est sujette aux erreurs (Al Mohammad et al., 2019 ; Armato et al., 2009 ; Gierada et al., 2017 ; Leader et al., 2005). Dans une étude portant sur près de deux mille patients, un algorithme basé sur le CNN conçu pour détecter automatiquement les nodules pulmonaires a été testé comme deuxième lecteur (Katase et al., 2022). Les cas témoins étaient constituée de nodules identifiés par deux radiologues expérimentés comme étant à haut risque selon l'histoire clinique ainsi que la morphologie du nodule. L'étude a révélé que l'utilisation de l'algorithme était associée à une sensibilité améliorée aux nodules à différents niveaux d'expérience du premier lecteur. Bien que la sensibilité globale soit plus faible pour les nodules en verre dépoli et les nodules de moins de 1 cm de diamètre, la sensibilité de ceux-ci était beaucoup plus élevée lorsque l'algorithme était utilisé que lorsque le radiologue interprétait seul les images. Les faux positifs incluaient des zones d'inflammation pleurale ou des vaisseaux périphériques, tandis que les faux négatifs étaient souvent des nodules en verre dépoli faibles ou mal délimités ou des nodules proches du diaphragme. Il est important de noter que les auteurs ont constaté des performances de modèle cohérentes sur une gamme de doses de rayonnement TDM dans une étude fantôme, ce qui indique que leurs résultats pourraient être généralisables à d’autres protocoles de TDM thoracique (Katase et al., 2022). Une autre étude a trouvé une sensibilité de 93 % et une spécificité de 96 % d'un algorithme basé sur le CNN pour la détection des nodules pulmonaires sur la LDCT par rapport au consensus de deux radiologues (Chamberlin et al., 2021). Les faux positifs dans cette étude comprenaient des zones d'atélectasie, des modifications parenchymateuses associées à une infection et des ostéophytes faisant saillie dans les champs pulmonaires à partir des vertèbres thoraciques.
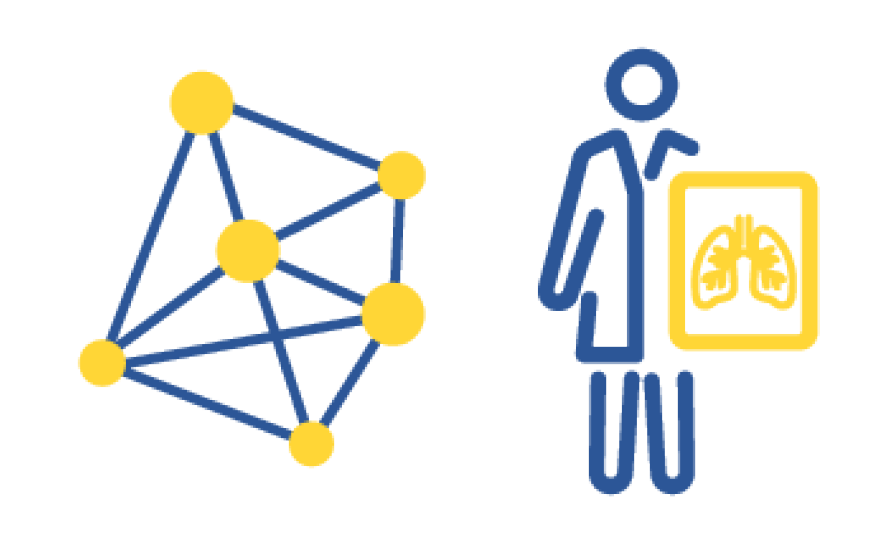
L’étude a révélé que l’utilisation de l’algorithme était associée à une sensibilité améliorée aux nodules à différents niveaux d’expérience du premier lecteur.
Au-delà de la simple identification des nodules pulmonaires, certaines études ont tenté de classer le risque de malignité des nodules identifiés. Un algorithme à plusieurs composants comprenant des modèles de segmentation pulmonaire, de détection des régions cancéreuses et de prédiction du cancer a été testé sur 6 716 LDCT et validé sur un ensemble de données indépendant de 1 139 LDCT (Ardila et al., 2019). L'algorithme génère une probabilité de malignité basée soit sur des LDCT uniques, soit, lorsqu'elles sont disponibles, sur des LDCT antérieures du même patient. En utilisant des cas de cancer du poumon prouvé par biopsie, l’algorithme a fonctionné aussi bien que six radiologues lorsque des LDCT antérieures étaient disponibles. Dans les cas sans LDCT préalable, l’algorithme avait un taux de faux positifs inférieur de 11 % et un taux de faux négatifs inférieur de 5 % à celui des radiologues.
Une évaluation du parenchyme pulmonaire sur la LDCT au-delà de la présence de nodules pulmonaires est une approche récente et prometteuse pour identifier le risque futur de cancer du poumon. Une étude a révélé qu'un algorithme 3D-CNN, testé sur plus de 15 000 LDCT, présentait une aire sous la courbe caractéristique de fonctionnement du récepteur (ASC) de 0,86 à 0,94 (selon l'ensemble de données) pour prédire le cancer du poumon à un an (Mikhael et al., 2023). Il est intéressant de noter que l’ASC de l’algorithme, après exclusion des cas où des nodules visibles étaient présents à la référence au même endroit que les futurs cancers, était de 0,82. L'algorithme a également montré un taux de faux positifs inférieur aux scores de malignité établis sur la base de la morphologie des nodules lorsque le volume total de la LDCT a été évalué par l'algorithme. Ces résultats suggèrent que d'autres caractéristiques au-delà des nodules suspects contribuaient à la prédiction de l'algorithme. Surtout, cela signifie que l’algorithme détecte des caractéristiques de la LDCT au-delà de ce que les radiologues considèrent généralement comme pertinents pour prédire le risque de cancer du poumon.
Aux États-Unis, les critères d'éligibilité au dépistage du cancer du poumon, qui émanent des Centers for Medicare et Medicaid Services (CMS), négligent plus de la moitié des cas de cancer du poumon (Y. Wang et al., 2015). Bien qu’il existe d’autres outils de « pré-dépistage » plus complexes, basés sur des scores, les informations qu’ils nécessitent, comme le nombre de paquets-années, sont souvent inexactes ou indisponibles (Kinsinger et al., 2017). L’IA a ainsi été utilisée pour identifier davantage de personnes à haut risque de cancer du poumon afin de les inclure dans les programmes de dépistage. Une étude portant sur 5 615 personnes a révélé qu'une combinaison de radiographies thoraciques simples, d'âge, de sexe et de statut tabagique actuel permet une sélection plus ciblée de patients pour le dépistage par la LDCT (Lu et al., 2020). Le modèle dans cette étude avait une ASC de 0,7 pour prédire un cancer du poumon incident sur 12 ans, contre une ASC de 0,63 pour les critères CMS, ce qui se traduit par 30,7 % de cas de cancer du poumon en moins manqués avec l'algorithme. Le modèle prédit également une mortalité par le cancer du poumon sur 12 ans avec une ASC de 0,76. Les auteurs ne recommandent pas les radiographies thoraciques de routine à des fins de pré-dépistage, mais préconisent l'utilisation de ce modèle chez les patients subissant des radiographies thoraciques pour d'autres indications cliniques.
Cancer colorectal
Le cancer colorectal est le troisième cancer le plus répandu chez les femmes et les hommes et constitue une cause majeure de décès par le cancer dans le monde (Sung et al., 2021). Il se développe comme une cascade d’événements à mesure que les cellules de la muqueuse intestinale accumulent des mutations génétiques, se transformant d’abord en muqueuse hyperproliférative, puis en adénome bénin et, dans certains cas, en adénocarcinome (Kuipers et al., 2015). Le dépistage du cancer colorectal est avant tout préventif : il vise à détecter les adénomes potentiellement cancéreux afin de pouvoir les éliminer, une approche qui réduit la mortalité de la maladie (Zauber et al., 2012).
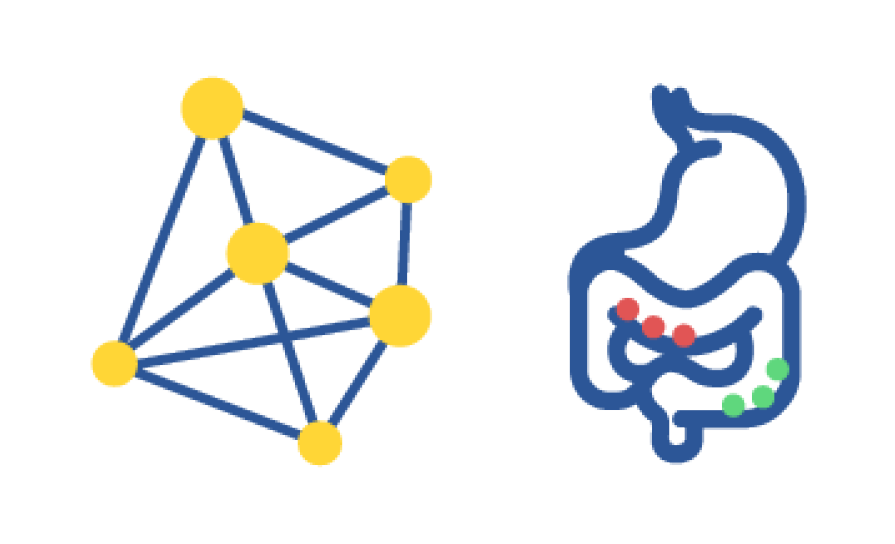
Une récente étude de validation de principe a utilisé une approche entièrement automatisée utilisant les CNN pour la segmentation des polypes et la distinction entre les polypes bénins et précancéreux.
Le dépistage du cancer colorectal est régulièrement effectué soit en recherchant du sang dans les selles à l'aide de tests très sensibles, soit en visualisant la lumière de l'intestin par coloscopie optique (Helsingen Lise M. & Kalager Mette, 2022). La coloscopie optique est une méthode établie et fiable pour identifier les adénomes colorectaux et permet de les éliminer immédiatement. Cependant, ses principaux inconvénients sont la faible observance du patient et la nécessité d'une sédation (Inadomi et al., 2012 ; Joseph et al., 2012 ; OECD, 2012 ; Stock et al., 2011 ; Use of Colorectal Cancer Screening Tests, 2023).
Une alternative émergente et prometteuse à la coloscopie optique est le coloscanner. Cette technique a une précision diagnostique similaire à celle de la coloscopie optique (Pickhardt et al., 2003, 2011, 2018), est préférée par les patients (Ristvedt et al., 2003) et offre une meilleure observance (Moawad et al., 2010). Elle ne nécessite pas non plus de sédation et peut détecter des résultats cliniquement pertinents en dehors de l'intestin qui sont invisibles à la coloscopie optique (Smyth et al., 2013). En revanche, la colonographie TDM nécessite une préparation intestinale (comme la coloscopie optique), expose le patient à certains rayonnements ionisants et ne permet pas la résection simultanée des polypes. Malgré ces inconvénients, l'American College of Radiology recommande la colonographie TDM pour le dépistage des patients présentant un risque moyen ou modéré de cancer colorectal (Expert Panel on Gastrointestinal Imaging: et al., 2018).
Les images de colonographie TDM subissent une série d’étapes de préparation avant d’être interprétées. Celles-ci incluent un prétraitement pour éliminer les artefacts, l’extraction du côlon du reste des structures abdominales, la reconstruction 3D du côlon et la visualisation de la lumière du côlon. Une étude récente a combiné une nouvelle méthode de segmentation et de reconstruction du côlon avec la détection des polypes à l'aide d'un CNN (Alkabbany et al., 2022). La segmentation automatisée du côlon a montré un chevauchement de plus de 90 % avec la segmentation manuelle experte dans 70 % des cas et des polypes du côlon ont été détectés avec une ASC de 0,93, une sensibilité de 97 % et une spécificité de 79 %.
La différenciation entre les polypes bénins et ceux potentiellement malins est un défi à la fois en coloscopie optique et en colonographie TDM et a fait l'objet de plusieurs études utilisant l'IA. Les approches basées sur la radiomique pour classer les polypes bénins et précancéreux sur la colonographie TDM ont montré des ASC allant jusqu'à 0,91, mais nécessitent une segmentation manuelle des polypes (Grosu et al., 2021 ; Song et al., 2014). Une récente étude de validation de principe a utilisé une approche entièrement automatisée utilisant les CNN pour la segmentation des polypes et la distinction entre les polypes bénins et précancéreux (Wesp et al., 2022). Les auteurs ont formé le CNN sur les données de 63 patients et l'ont testé sur un ensemble de données indépendant de 59 patients, montrant une ASC allant jusqu'à 0,83 et une sensibilité et une spécificité allant jusqu'à 80 % et 69 % respectivement. De telles approches basées sur l’IA peuvent potentiellement être utilisées comme deuxième lecteur pour aider à orienter la décision concernant l’élimination des polypes.
Carcinome hépatocellulaire
Le carcinome hépatocellulaire (CHC) est l’une des causes les plus courantes de décès par cancer dans le monde (Sung et al., 2021). Les personnes atteintes d’une cirrhose du foie ou d’une infection chronique par le virus de l’hépatite B ou C courent un risque élevé de développer un CHC (Vogel et al., 2022). Le dépistage de ces patients est associé à une réduction de la mortalité par CHC (Singal et al., 2022 ; Zhang et al., 2004). Le dépistage est généralement réalisé par échographie abdominale tous les six mois (Association européenne pour l'étude du foie, 2018 ; Frenette et al., 2019 ; Marrero et al., 2018) avec ou sans mesure des taux d'alpha-foetoprotéine dans le sang (Colli et al., 2006 ; Tzartzeva et al., 2018). Les lésions suspectes identifiées par échographie sont ensuite caractérisées par TDM, IRM ou les deux. La pathogenèse du CHC implique une interaction complexe entre les nodules hépatiques qui existent à différents stades de lésions hépatiques chroniques. Des nodules régénératifs se forment en réponse à des lésions hépatocytaires et sont fréquemment observés dans les foies cirrhotiques. Les mutations génétiques peuvent s'accumuler au fil du temps dans ces nodules régénératifs, les convertissant en nodules dysplasiques avec un risque élevé d'évolution vers un CHC à mesure que davantage de mutations s'accumulent (Kudo, 2009). Faire la différence entre les nodules dysplasiques et malins à l’aide de l’imagerie est difficile (Park et al., 2017). En outre, les caractéristiques d'imagerie du CHC se confondent parfois avec celles d'autres lésions hépatiques, notamment les hémangiomes, les kystes hépatiques simples et l'hyperplasie nodulaire focale (Heiken, 2007).
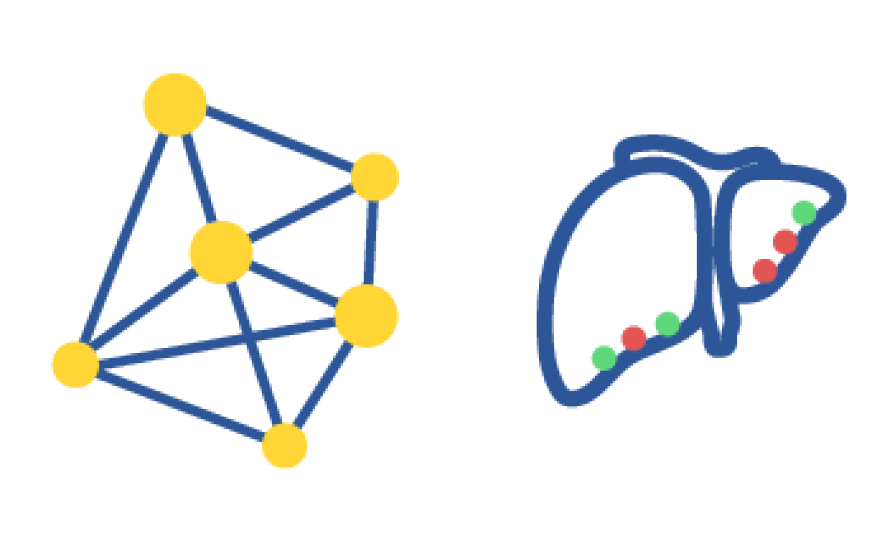
Les techniques d’apprentissage profond ont également été largement appliquées à l’imagerie hépatique à l’aide de l’échographie en mode B, montrant des résultats prometteurs pour détecter et classer les lésions focales du foie comme bénignes ou malignes.
En utilisant une approche radiomique combinant des informations de perfusion et une analyse de texture en échographie avec contraste amélioré, une étude portant sur 72 patients a trouvé une précision équilibrée de 0,84 pour distinguer les lésions hépatiques bénignes et malignes (Turco et al., 2022). Une autre étude utilisant l'échographie avec contraste amélioré a révélé une sensibilité de 94,8 % et une spécificité de 93,6 % pour distinguer le CHC de l'hyperplasie nodulaire focale à l'aide d'une approche d'apprentissage automatique à vecteurs de support (Huang et al., 2020), d'autres études trouvant des résultats similaires (Gatos et al., 2015 ; Kondo et al., 2017). Dans une étude multicentrique examinant la différenciation de 11 types différents de lésions focales du foie en utilisant l'échographie avec contraste amélioré et l'histopathologie comme référence, l'apprentissage automatique des vecteurs de support (ASC = 0,883) a surpassé un réseau neuronal artificiel (ASC = 0,829) et les deux approches ont surpassé un radiologue expérimenté (ASC = 0,702) (Ta et al., 2018).
Les techniques d’apprentissage profond ont également été largement appliquées à l’imagerie hépatique utilisant l’échographie en mode B. Ces études ont montré des résultats prometteurs pour détecter (Brehar et al., 2020 ; Schmauch et al., 2019 ; Tiyarattanachai et al., 2022) et classer les lésions focales hépatiques comme bénignes ou malignes (Schmauch et al., 2019) ou les classer en entités spécifiques (Hassan et al., 2017 ; Virmani et al., 2014). En utilisant une approche d'apprentissage profond, une étude a révélé que la combinaison d'informations sur les données démographiques des patients et les résultats de laboratoire avec des images échographiques en mode B améliorait l'ASC pour classer les lésions hépatiques comme bénignes ou malignes de 0,721 (en utilisant uniquement l'échographie) à 0,994 (Sato et al., 2022). Une autre étude portant sur 334 patients a révélé que le taux de détection des lésions focales du foie par échographie en mode B utilisant un CNN était plus élevé pour le CHC que pour les autres lésions focales du foie et que le CNN surpassait les experts humains (avec un taux de détection d'algorithme de 100 % contre 39,1 % pour les non-radiologues et 69,6 % pour les radiologues) (Tiyarattanachai et al., 2022).
Cancer de la prostate
Le cancer de la prostate est le cancer le plus répandu chez les hommes en Europe et aux États-Unis (Ferlay et al., 2018 ; Siegel et al., 2021) et le troisième cancer le plus répandu dans le monde (Sung et al., 2021). Dans les pays où des programmes existent, le dépistage repose généralement sur la mesure des taux sériques d’antigène spécifique de la prostate (PSA). Le PSA sérique a une sensibilité élevée mais une faible spécificité pour le cancer de la prostate (Merriel et al., 2022). Le dépistage basé sur le PSA seul conduit ainsi à de nombreuses biopsies inutiles, avec jusqu'à 75 % des biopsies systématiques de la prostate - celles réalisées sans cibler un endroit spécifique de la prostate, mais en prenant plusieurs biopsies dans différentes parties de la glande - étant négatives (Ahmed et al., 2017). De plus, le dépistage du PSA a tendance à détecter un cancer à faible risque et à croissance plus lente, considéré comme cliniquement insignifiant car il ne menace pas la survie du patient (US Preventive Services Task Force et al., 2018 ; Welch & Albertsen, 2020). Un dépistage basé sur le taux sérique de PSA suivi d'une biopsie systématique présente donc un bénéfice globalement discutable. L’approche idéale consisterait plutôt à détecter le cancer et à caractériser simultanément sa signification clinique.
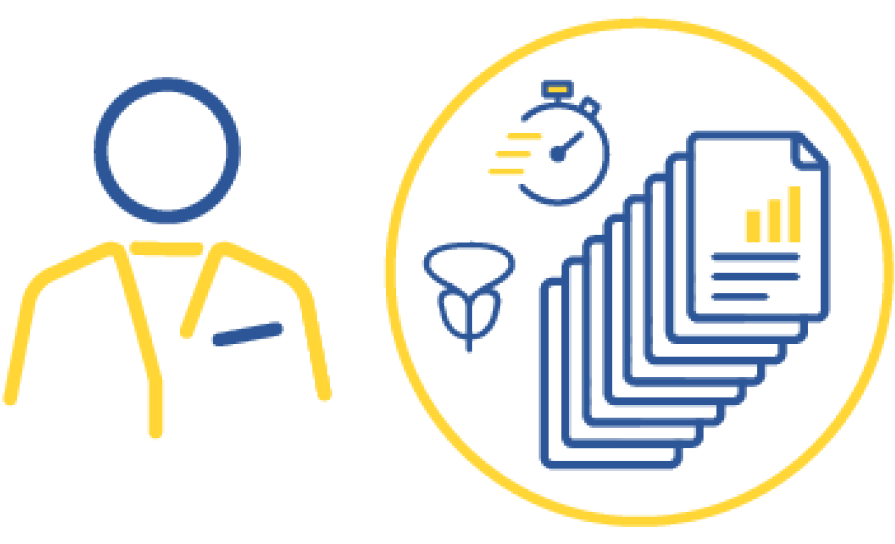
Une étude utilisant un classificateur basé sur les forêts aléatoires pour détecter les zones suspectes sur l’IRM multiparamétrique de la prostate a été associée à des temps de lecture plus courts et à une spécificité améliorée.
L’IRM multiparamétrique joue un rôle de plus en plus important dans le traitement des cas de cancer de la prostate dépistés et comprend des séquences pondérées en diffusion et pondérées T2, avec ou sans séquence dynamique à contraste amélioré pondérée T1 (Walker et al., 2020). Les faux positifs et la détection d’un cancer de la prostate cliniquement insignifiant peuvent être réduits grâce à l’IRM, ce qui peut contribuer à réduire le surtraitement (Drost et al., 2019). Des études suggèrent que l'IRM avant la biopsie peut réduire d'un tiers le nombre de biopsies inutiles (Elwenspoek et al., 2019), et cette approche a été incluse dans plusieurs lignes directrices sur la prise en charge du cancer de la prostate (Leitlinienprogramm Onkologie: Prostatakarzinom, n.d., Overview | Prostate Cancer: Diagnosis and Management | Guidance | NICE, n.d. ; Mottet et al., 2017). L’IRM peut également aider à orienter les biopsies ciblées chez les patients présentant des biopsies systématiques négatives de la prostate (Hoeks et al., 2012 ; Hugosson et al., 2022 ; Penzkofer et al., 2015 ; Siddiqui et al., 2015 ; Sonn et al., 2014). . Chez les patients présentant un risque de cancer de la prostate à très faible ou faible risque, l’IRM peut être utile pour surveiller activement la maladie, une approche associée à de bons résultats à long terme (Klotz et al., 2015). La lecture des IRM de la prostate est cependant difficile et même les systèmes de rapports standardisés ont une courbe d'apprentissage abrupte, et les performances diagnostiques varient considérablement entre les radiologues et les établissements (Kohestani et al., 2019 ; Muller et al., 2015 ; Rosenkrantz et al., 2017 ; Smith et al., 2019 ; Westphalen et al., 2020).
La segmentation de l'ensemble de la prostate permet de déterminer le volume de la glande qui est utilisé pour calculer la densité de PSA (une mesure qui permet de différencier l'hypertrophie bénigne de la prostate et le cancer de la prostate) et pour planifier la radiothérapie. La segmentation manuelle de la prostate par les radiologues prend cependant du temps et est sujette à des erreurs (Garvey et al., 2014). La segmentation automatisée de la prostate à l'aide d'outils basés sur l'IA est réalisable et précise, et plusieurs outils commerciaux sont actuellement disponibles à cet effet (AI for Radiology, n.d. ; Bardis et al., 2021 ; Belue & Turkbey, 2022 ; Sanford et al., 2020 ; Sunoqrot et al., 2022 ; Turkbey & Haider, 2022 ; Ushinsky et al., 2021 ; van Leeuwen et al., 2021 ; B. Wang et al., 2019).
Plusieurs études utilisant des approches d'apprentissage profond ont atteint des ASC allant jusqu'à 0,89 pour la détection du cancer de la prostate par IRM multiparamétrique (Arif et al., 2020 ; Saha et al., 2021). Un algorithme basé sur l'apprentissage profond disponible dans le commerce a amélioré la détection par les radiologues du cancer de la prostate cliniquement significatif (en utilisant le consensus de trois radiologues expérimentés comme référence), a augmenté la fiabilité entre les lecteurs et a réduit le temps de lecture médian (Winkel et al., 2021). Comme dans le cas du cancer du sein, la précision du diagnostic est plus élevée lorsque les outils basés sur l'IA et les interprétations des radiologues sont considérés ensemble plutôt que de s'appuyer sur l'évaluation de l'un ou de l'autre (Cacciamani et al., 2023).
L’IA a également été utilisée pour classer l’agressivité du cancer de la prostate. Dans une étude radiomique basée sur l’IRM, un classificateur de machine à vecteurs de support a été utilisé pour segmenter les zones de cancer de la prostate, suivi d’une analyse de texture et d’une extraction de caractéristiques quantitatives (Giannini et al., 2021). Dans la même étude, un autre classificateur de machine à vecteurs de support a utilisé les caractéristiques extraites pour classer l’agressivité des tumeurs en utilisant le classement histopathologique comme référence. Basée sur les données de 72 patients, l'étude a trouvé une ASC de 0,81 dans un ensemble de données de validation de 59 patients (valeur prédictive positive = 81 %, valeur prédictive négative = 71 %). Dans une autre étude portant sur les IRM multiparamétriques de la prostate de 107 patients, les classifications PI-RADS des radiologues ont été combinées avec un score de vraisemblance dérivé d'un classificateur en forêts aléatoires, et toutes les régions suspectes ainsi identifiées ont été biopsiées (Litjens et al., 2015). L'inclusion du score de l'algorithme était associée à une probabilité plus élevée de détecter un cancer de la prostate (ASC = 0,88 avec et 0,81 sans l'algorithme) et de détecter des cancers plus agressifs (ASC = 0,87 avec et 0,78 sans l'algorithme). Dans une étude portant sur 417 patients, un CNN a atteint une ASC de 0,81 pour classer le cancer de la prostate cliniquement significatif à l'aide d'une IRM multiparamétrique avec une sensibilité légèrement inférieure à celle des radiologues hautement expérimentés (Cao et al., 2019).
Comme pour de nombreuses autres applications de l’IA en radiologie, le manque d’interprétabilité des modèles d’apprentissage profond de l’IRM de la prostate entrave et retarde leur mise en oeuvre dans la pratique clinique (Aristidou et al., 2022 ; Reddy et al., 2020 ; Reyes et al., 2020 ; Vayena et al., 2018). Une étude utilisant un CNN sur l'IRM de la prostate de 1 224 patients et l'histopathologie comme référence a trouvé une ASC de 0,89 pour distinguer le cancer de la prostate cliniquement significatif des autres modifications de la prostate (Hamm et al., 2023). En outre, ils comprenaient une carte thermique voxelwise des zones suspectes de cancer de la prostate cliniquement significatif et des explications descriptives inspirées de PI-RADS sur la façon dont le CNN est arrivé à sa conclusion. L'algorithme a été associé à une réduction du temps de lecture de 85 secondes à 47 secondes et à une augmentation de la confiance en lecture chez les lecteurs non experts.
Conclusion
L’imagerie médicale joue un rôle central dans le dépistage de plusieurs des cancers les plus courants. La lecture des examens de dépistage nécessite des compétences et une expérience considérables, et la demande actuelle dépasse de loin l'offre de radiologues qualifiés (AAMC Report Reinforces Mounting Physician Shortage, 2021, Clinical Radiology UK Workforce Census 2019 Report, 2019). L’utilisation d’outils basés sur l’IA pour le dépistage du cancer est extrêmement prometteuse pour atténuer ces problèmes. Les avantages de ces approches comprennent une meilleure identification des personnes éligibles au dépistage, une meilleure précision du diagnostic, des délais de rapports réduits et une confiance accrue des radiologues dans leurs propres décisions diagnostiques. Les résultats les plus prometteurs ont été obtenus lorsque les systèmes basés sur l’IA et les radiologues ont pris ensemble des décisions concernant les examens de dépistage. La prise de décision collaborative entre les outils basés sur l’IA et les radiologues peut ainsi ouvrir la voie à une ère de transformation dans le dépistage du cancer.
Références
AAMC Report Reinforces Mounting Physician Shortage. (2021). AAMC. https://www.aamc.org/news-insights/press-releases/aamc-report-reinforces-mounting-physician-shortage
Aggarwal, R., Sounderajah, V., Martin, G., Ting, D. S. W., Karthikesalingam, A., King, D., Ashrafian, H., & Darzi, A. (2021). Diagnostic accuracy of deep learning in medical imaging: a systematic review and meta-analysis. NPJ Digital Medicine, 4(1), 65. https://doi.org/10.1038/s41746-021-00438-z
Ahmed, H. U., El-Shater Bosaily, A., Brown, L. C., Gabe, R., Kaplan, R., Parmar, M. K., Collaco-Moraes, Y., Ward, K., Hindley, R. G., Freeman, A., Kirkham, A. P., Oldroyd, R., Parker, C., Emberton, M., & PROMIS study group. (2017). Diagnostic accuracy of multi-parametric MRI and TRUS biopsy in prostate cancer (PROMIS): a paired validating confirmatory study. The Lancet, 389(10071), 815–822. https://doi.org/10.1016/ S0140-6736(16)32401-1
AI for radiology. (n.d.). Retrieved June 4, 2023, from https://grand-challenge.org/aiforradiology/?subspeciality=All& modality=All&ce_under=All&ce_%20class=All&fda_ class=All&sort_by=ce+certification&search=prostate
Alkabbany, I., Ali, A. M., Mohamed, M., Elshazly, S. M., & Farag, A. (2022). An AI-Based Colonic Polyp Classifier for Colorectal Cancer Screening Using Low-Dose Abdominal CT. Sensors, 22, (24). https://doi.org/10.3390/s22249761
Al Mohammad, B., Hillis, S. L., Reed, W., Alakhras, M., & Brennan, P. C. (2019). Radiologist performance in the detection of lung cancer using CT. Clinical Radiology, 74(1), 67–75. https://doi.org/10.1016/j.crad.2018.10.008
Ardila, D., Kiraly, A. P., Bharadwaj, S., Choi, B., Reicher, J. J., Peng, L., Tse, D., Etemadi, M., Ye, W., Corrado, G., Naidich, D. P., & Shetty, S. (2019). End-to-end lung cancer screening with three-dimensional deep learning on low-dose chest computed tomography. Nature Medicine, 25(6), 954–961. https://doi. org/10.1038/s41591-019-0447-x
Arif, M., Schoots, I. G., Castillo Tovar, J., Bangma, C. H., Krestin, G. P., Roobol, M. J., Niessen, W., & Veenland, J. F. (2020). Clinically significant prostate cancer detection and segmentation in low-risk patients using a convolutional neural network on multi-parametric MRI. European Radiology, 30(12), 6582–6592. https://doi.org/10.1007/s00330-020-07008-z
Aristidou, A., Jena, R., & Topol, E. J. (2022). Bridging the chasm between AI and clinical implementation. The Lancet, 399(10325), 620. https://doi.org/10.1016/S0140-6736(22)00235-5
Armato, S. G., 3rd, Roberts, R. Y., Kocherginsky, M., Aberle, D. R., Kazerooni, E. A., Macmahon, H., van Beek, E. J. R., Yankelevitz, D., McLennan, G., McNitt-Gray, M. F., Meyer, C. R., Reeves, A. P., Caligiuri, P., Quint, L. E., Sundaram, B., Croft, B. Y., & Clarke, L. P. (2009). Assessment of radiologist performance in the detection of lung nodules: dependence on the definition of "truth." Academic Radiology, 16(1), 28–38. https://doi.org/10.1016/j.acra.2008.05.022
Banks, E., Reeves, G., Beral, V., Bull, D., Crossley, B., Simmonds, M., Hilton, E., Bailey, S., Barrett, N., Briers, P., English, R., Jackson, A., Kutt, E., Lavelle, J., Rockall, L., Wallis, M. G., Wilson, M., & Patnick, J. (2006). Hormone replacement therapy and false positive recall in the Million Women Study: patterns of use, hormonal constituents and consistency of effect. Breast Cancer Research: BCR, 8(1), R8. https://doi.org/10.1186/bcr1364
Bardis, M., Houshyar, R., Chantaduly, C., Tran-Harding, K., Ushinsky, A., Chahine, C., Rupasinghe, M., Chow, D., & Chang, P. (2021). Segmentation of the Prostate Transition Zone and Peripheral Zone on MR Images with Deep Learning. Radiology. Imaging Cancer, 3(3), e200024. https://doi.org/10.1148/rycan.2021200024
Belue, M. J., & Turkbey, B. (2022). Tasks for artificial intelligence in prostate MRI. European Radiology Experimental, 6(1), 33. https://doi.org/10.1186/s41747-022-00287-9
Boyd, N. F., Guo, H., Martin, L. J., Sun, L., Stone, J., Fishell, E., Jong, R. A., Hislop, G., Chiarelli, A., Minkin, S., & Yaffe, M. J. (2007). Mammographic density and the risk and detection of breast cancer. The New England Journal of Medicine, 356(3), 227–236. https://doi.org/10.1056/NEJMoa062790
Bray, F., Ferlay, J., Soerjomataram, I., Siegel, R. L., Torre, L. A., & Jemal, A.(2018). Global cancer statistics 2018: GLOBOCAN estimates of incidence and mortality worldwide for 36 cancers in 185 countries. CA: A Cancer Journal for Clinicians, 68(6), 394–424. https://doi.org/10.3322/caac.21492
Brehar, R., Mitrea, D.-A., Vancea, F., Marita, T., Nedevschi, S., Lupsor-Platon, M., Rotaru, M., & Badea, R. I. (2020). Comparison of Deep-Learning and Conventional Machine- Learning Methods for the Automatic Recognition of the Hepatocellular Carcinoma Areas from Ultrasound Images. Sensors, 20(11). https://doi.org/10.3390/s20113085
Cacciamani, G. E., Sanford, D. I., Chu, T. N., Kaneko, M., De Castro Abreu, A. L., Duddalwar, V., & Gill, I. S. (2023). Is Artificial Intelligence Replacing Our Radiology Stars? Not Yet! European Urology Open Science, 48, 14–16. https://doi.org/10.1016/j.euros.2022.09.024
Cao, R., Mohammadian Bajgiran, A., Afshari Mirak, S., Shakeri, S., Zhong, X., Enzmann, D., Raman, S., & Sung, K. (2019). Joint Prostate Cancer Detection and Gleason Score Prediction in mp-MRI via FocalNet. IEEE Transactions on Medical Imaging, 38 (11), 2496–2506. https://doi.org/10.1109/ TMI.2019.2901928
Castells, X., Molins, E., & Macià, F. (2006). Cumulative false positive recall rate and association with participant related factors in a population based breast cancer screening programme. Journal of Epidemiology and Community Health, 60(4), 316–321. https://doi.org/10.1136/jech.2005.042119
Chamberlin, J., Kocher, M. R., Waltz, J., Snoddy, M., Stringer, N. F. C., Stephenson, J., Sahbaee, P., Sharma, P., Rapaka, S., Schoepf, U. J., Abadia, A. F., Sperl, J., Hoelzer, P., Mercer, M., Somayaji, N., Aquino, G., & Burt, J. R. (2021). Automated detection of lung nodules and coronary artery calcium using artificial intelligence on low-dose CT scans for lung cancer screening: accuracy and prognostic value. BMC Medicine, 19(1), 55. https://doi.org/10.1186/s12916-021-01928-3
Clinical radiology UK workforce census 2019 report. (2019). https://www.rcr.ac.uk/publication/clinical-radiology-uk- workforce-census-2019-report
Colli, A., Fraquelli, M., Casazza, G., Massironi, S., Colucci, A., Conte, D., & Duca, P. (2006). Accuracy of ultrasonography, spiral CT, magnetic resonance, and alpha-fetoprotein in diagnosing hepatocellular carcinoma: a systematic review. The American Journal of Gastroenterology, 101(3), 513–523. https://doi.org/10.1111/j.1572-0241.2006.00467
Dembrower, K., Wåhlin, E., Liu, Y., Salim, M., Smith, K., Lindholm, P., Eklund, M., & Strand, F. (2020). Effect of artificial intelligence-based triaging of breast cancer screening mammograms on cancer detection and radiologist workload: a retrospective simulation study. The Lancet. Digital Health, 2(9), e468–e474. https://doi.org/10.1016/S2589-7500(20)30185-0
Drost, F.-J. H., Osses, D. F., Nieboer, D., Steyerberg, E. W., Bangma, C. H., Roobol, M. J., & Schoots, I. G.(2019). Prostate MRI, with or without MRI-targeted biopsy, and systematic biopsy for detecting prostate cancer. Cochrane Database of Systematic Reviews, 4(4), CD012663. https://doi.org/10.1002/14651858.CD012663.pub2
Elmore, J. G., Jackson, S. L., Abraham, L., Miglioretti, D. L., Carney, P. A., Geller, B. M., Yankaskas, B. C., Kerlikowske, K., Onega, T., Rosenberg, R. D., Sickles, E. A., & Buist, D. S. M. (2009). Variability in interpretive performance at screening mammography and radiologists’ characteristics associated with accuracy. Radiology, 253(3), 641–651. https://doi.org/10.1148/radiol.2533082308
Elwenspoek, M. M. C., Sheppard, A. L., McInnes, M. D. F., Merriel, S. W. D., Rowe, E. W. J., Bryant, R. J., Donovan, J. L., & Whiting, P. (2019). Comparison of Multiparametric Magnetic Resonance Imaging and Targeted Biopsy With Systematic Biopsy Alone for the Diagnosis of Prostate Cancer: A Systematic Review and Meta-analysis. JAMA Network Open, 2(8), e198427. https://doi.org/10.1001/jamanetworkopen.2019.8427
European Association for the Study of the Liver. (2018). EASL Clinical Practice Guidelines: Management of hepatocellular carcinoma. Journal of Hepatology, 69(1), 182–236. https://doi.org/10.1016/j.jhep.2018.03.019
Existence of national screening program for breast cancer. (n.d.). Retrieved April 2, 2023, from https://www.who.int/data/gho/data/indicators/indicator-details/GHO/existence-ofnational-screening-program-for-breast-cancer
Expert Panel on Gastrointestinal Imaging:, Moreno, C., Kim, D. H., Bartel, T. B., Cash, B. D., Chang, K. J., Feig, B. W., Fowler, K. J., Garcia, E. M., Kambadakone, A. R., Lambert, D. L., Levy, A. D., Marin, D., Peterson, C. M., Scheirey, C. D., Smith, M. P., Weinstein, S., & Carucci, L. R. (2018). ACR Appropriateness Criteria® Colorectal Cancer Screening. Journal of the American College of Radiology: JACR, 15(5S), S56–S68. https://doi.org/10.1016/j.jacr.2018.03.014
Ferlay, J., Colombet, M., Soerjomataram, I., Dyba, T., Randi,G., Bettio, M., Gavin, A., Visser, O., & Bray, F.(2018). Cancer incidence and mortality patterns in Europe: Estimates for 40 countries and 25 major cancers in 2018. European Journal of Cancer, 103, 356–387. https://doi.org/10.1016/j.ejca.2018.07.005
Freeman, K., Geppert, J., Stinton, C., Todkill, D., Johnson, S., Clarke, A., & Taylor-Phillips, S. (2021). Use of artificial intelligence for image analysis in breast cancer screening programmes: systematic review of test accuracy.BMJ, 374, n1872.https://doi.org/10.1136/bmj.n1872
Frenette, C. T., Isaacson, A. J., Bargellini, I., Saab, S., & Singal, A. G. (2019). A Practical Guideline for Hepatocellular Carcinoma Screening in Patients at Risk. Mayo Clinic Proceedings. Innovations, Quality & Outcomes, 3(3), 302–310. https://doi.org/10.1016/j.mayocpiqo.2019.04.005
Garvey, B., Türkbey, B., Truong, H., Bernardo, M., Periaswamy, S., & Choyke, P. L. (2014). Clinical value of prostate segmentation and volume determination on MRI in benign prostatic hyperplasia. Diagnostic and Interventional Radiology, 20(3), 229–233. https://doi.org/10.5152/dir.2014.13322
Gatos, I., Tsantis, S., Spiliopoulos, S., Skouroliakou, A., Theotokas, I., Zoumpoulis, P., Hazle, J. D., & Kagadis, G. C. (2015). A new automated quantification algorithm for the detection and evaluation of focal liver lesions with contrastenhanced ultrasound. Medical Physics, 42(7), 3948–3959. https://doi.org/10.1118/1.4921753
Gaur, S., Lay, N., Harmon, S. A., Doddakashi, S., Mehralivand, S., Argun, B., Barrett, T., Bednarova, S., Girometti, R., Karaarslan, E., Kural, A. R., Oto, A., Purysko, A. S., Antic, T., Magi-Galluzzi, C., Saglican, Y., Sioletic, S., Warren, A. Y., Bittencourt, L., … Turkbey, B. (2018). Can computeraided diagnosis assist in the identification of prostate cancer on prostate MRI? a multi-center, multi-reader investigation. Oncotarget, 9(73), 33804–33817. https://doi.org/10.18632/oncotarget.26100
Giannini, V., Mazzetti, S., Defeudis, A., Stranieri, G., Calandri, M., Bollito, E., Bosco, M., Porpiglia, F., Manfredi, M., De Pascale, A., Veltri, A., Russo, F., & Regge, D. (2021). A Fully Automatic Artificial Intelligence System Able to Detect and Characterize Prostate Cancer Using Multiparametric MRI: Multicenter and Multi-Scanner Validation. Frontiers in Oncology, 11, 718155. https://doi.org/10.3389/fonc.2021.718155
Gierada, D. S., Pinsky, P. F., Duan, F., Garg, K., Hart, E. M., Kazerooni, E. A., Nath, H., Watts, J. R., Jr, & Aberle, D. R. (2017). Interval lung cancer after a negative CT screening examination: CT findings and outcomes in National Lung Screening Trial participants. European Radiology, 27(8), 3249–3256. https://doi.org/10.1007/s00330-016-4705-8
Giordano, L., von Karsa, L., Tomatis, M., Majek, O., de Wolf, C., Lancucki, L., Hofvind, S., Nyström, L., Segnan, N., Ponti, A., Eunice Working Group, Van Hal, G., Martens, P., Májek, O., Danes, J., von Euler-Chelpin, M., Aasmaa, A., Anttila, A., Becker, N., … Suonio, E. (2012). Mammographic screening programmes in Europe: organization, coverage and participation. Journal of Medical Screening, 19 Suppl 1, 72–82. https://doi.org/10.1258/jms.2012.012085
Grosu, S., Wesp, P., Graser, A., Maurus, S., Schulz, C., Knösel, T., Cyran, C. C., Ricke, J., Ingrisch, M., & Kazmierczak, P. M. (2021). Machine Learning-based Differentiation of Benign and Premalignant Colorectal Polyps Detected with CT Colonography in an Asymptomatic Screening Population: A Proof-of-Concept Study. Radiology, 299(2), 326–335. https://doi.org/10.1148/radiol.2021202363
Hamm, C. A., Baumgärtner, G. L., Biessmann, F., Beetz, N. L., Hartenstein, A., Savic, L. J., Froböse, K., Dräger, F., Schallenberg, S., Rudolph, M., Baur, A. D. J., Hamm, B., Haas, M., Hofbauer, S., Cash, H., & Penzkofer, T.(2023). Interactive Explainable Deep Learning Model Informs Prostate Cancer Diagnosis at MRI. Radiology, 307(4), e222276. https://doi.org/10.1148/radiol.222276
Hassan, T. M., Elmogy, M., & Sallam, E.-S. (2017). Diagnosis of Focal Liver Diseases Based on Deep Learning Technique for Ultrasound Images. Arabian Journal for Science and Engineering, 42(8), 3127–3140. https://doi.org/10.1007/s13369-016-2387-9
Heiken, J. P. (2007). Distinguishing benign from malignant liver tumours. Cancer Imaging: The Official Publication of the International Cancer Imaging Society, 7 Spec No A(Special issue A), S1–S14. https://doi.org/10.1102/1470-7330.2007.9084
Helsingen Lise M., & Kalager Mette. (2022). Colorectal Cancer Screening — Approach, Evidence, and Future Directions. NEJM Evidence, 1(1), EVIDra2100035. https://doi.org/10.1056/EVIDra2100035
Hoeks, C. M. A., Schouten, M. G., Bomers, J. G. R., Hoogendoorn, S. P., Hulsbergen-van de Kaa, C. A., Hambrock, T., Vergunst, H., Sedelaar, J. P. M., Fütterer, J. J., & Barentsz, J. O. (2012). Three-Tesla magnetic resonance-guided prostate biopsy in men with increased prostate-specific antigen and repeated, negative, random, systematic, transrectal ultrasound biopsies: detection of clinically significant prostate cancers. European Urology, 62(5), 902–909. https://doi.org/10.1016/j.eururo.2012.01.047
Huang, Q., Pan, F., Li, W., Yuan, F., Hu, H., Huang, J., Yu, J., & Wang, W. (2020). Differential Diagnosis of Atypical Hepatocellular Carcinoma in Contrast-Enhanced Ultrasound Using Spatio-Temporal Diagnostic Semantics. IEEE Journal of Biomedical and Health Informatics, 24(10), 2860–2869. https://doi.org/10.1109/JBHI.2020.2977937
Hugosson, J., Månsson, M., Wallström, J., Axcrona, U., Carlsson, S. V., Egevad, L., Geterud, K., Khatami, A., Kohestani, K., Pihl, C.-G., Socratous, A., Stranne, J., Godtman, R. A., Hellström, M., & GÖTEBORG-2 Trial Investigators. (2022). Prostate Cancer Screening with PSA and MRI Followed by Targeted Biopsy Only.The New England Journal of Medicine, 387(23), 2126–2137. https://doi.org/10.1056/NEJMoa2209454
Inadomi, J. M., Vijan, S., Janz, N. K., Fagerlin, A., Thomas, J. P., Lin, Y. V., Muñoz, R., Lau, C., Somsouk, M., El-Nachef, N., & Hayward, R. A. (2012). Adherence to colorectal cancer screening: a randomized clinical trial of competing strategies. Archives of Internal Medicine, 172(7), 575–582. https://doi. org/10.1001/archinternmed. 2012.332
Joseph, D. A., Jessica B. King, M. P. H., Miller, J. W., & Richardson, L. C. (2012, June 15).Prevalence of Colorectal Cancer Screening Among Adults — Behavioral Risk Factor Surveillance System, United States, 2010. https://www.cdc.gov/MMWr/preview/mmwrhtml/su6102a9.htm
Katase, S., Ichinose, A., Hayashi, M., Watanabe, M., Chin,K., Takeshita, Y., Shiga, H., Tateishi, H., Onozawa, S., Shirakawa, Y., Yamashita, K., Shudo, J., Nakamura, K., Nakanishi, A., Kuroki, K., & Yokoyama, K. (2022). Development and performance evaluation of a deep learning lung nodule detection system. BMC Medical Imaging. 22(1), 203. https://doi.org/10.1186/s12880-022-00938-8
Kinsinger, L. S., Anderson, C., Kim, J., Larson, M., Chan, S. H., King, H. A., Rice, K. L., Slatore, C. G., Tanner, N. T., Pittman, K., Monte, R. J., McNeil, R. B., Grubber, J. M., Kelley, M. J., Provenzale, D., Datta, S. K., Sperber, N. S., Barnes, L. K., Abbott, D. H., … Jackson, G. L. (2017). Implementation of Lung Cancer Screening in the Veterans Health Administration. JAMA Internal Medicine, 177(3), 399–406. https://doi.org/10.1001/jamainternmed.2016.9022
Klotz, L., Vesprini, D., Sethukavalan, P., Jethava, V., Zhang, L., Jain, S., Yamamoto, T., Mamedov, A., & Loblaw, A. (2015). Long-term follow-up of a large active surveillance cohort of patients with prostate cancer.Journal of Clinical Oncology: Official Journal of the American Society of Clinical Oncology, 33(3), 272–277.https://doi.org/10.1200/JCO.2014.55.1192
Kohestani, K., Wallström, J., Dehlfors, N., Sponga, O. M., Månsson, M., Josefsson, A., Carlsson, S., Hellström, M., & Hugosson, J. (2019). Performance and inter-observer variability of prostate MRI (PI-RADS version 2) outside high-volume centres. Scandinavian Journal of Urology, 53(5), 304–311. https://doi.org/10.1080/21681805.2019.1675757
Kondo, S., Takagi, K., Nishida, M., Iwai, T., Kudo, Y., Ogawa, K., Kamiyama, T., Shibuya, H., Kahata, K., & Shimizu, C. (2017). Computer-Aided Diagnosis of Focal Liver Lesions Using Contrast-Enhanced Ultrasonography With Perflubutane Microbubbles. IEEE Transactions on Medical Imaging, 36(7),1427–1437. https://doi.org/10.1109/TMI.2017.2659734
Kramer, B. S. (2004). The science of early detection. Urologic Oncology, 22(4), 344–347. https://doi.org/10.1016/j.urolonc.2003.04.001
Kudo, M. (2009). Multistep human hepatocarcinogenesis: correlation of imaging with pathology. Journal of Gastroenterology, 44 Suppl 19, 112–118. https://doi.org/10.1007/s00535-008-2274-6
Kuipers, E. J., Grady, W. M., Lieberman, D., Seufferlein, T., Sung, J. J., Boelens, P. G., van de Velde, C. J. H., & Watanabe, T. (2015) Colorectal cancer.Nature Reviews. Disease Primers, 1, 15065. https://doi.org/10.1038/nrdp.2015.65
Lam, D. L., Pandharipande, P. V., Lee, J. M., Lehman, C. D., & Lee, C. I. (2014). Imaging-based screening: understanding the controversies.AJR. American Journal of Roentgenology, 203(5), 952–956. https://doi.org/10.2214/AJR.14.13049
Lauritzen, A. D., Rodríguez-Ruiz, A., von Euler-Chelpin, M. C., Lynge, E., Vejborg, I., Nielsen, M., Karssemeijer, N., & Lillholm, M. (2022). An Artificial Intelligence-based Mammography Screening Protocol for Breast Cancer: Outcome and Radiologist Workload. Radiology, 304(1), 41–49. https://doi.org/10.1148/radiol.210948
Leader, J. K., Warfel, T. E., Fuhrman, C. R., Golla, S. K., Weissfeld, J. L., Avila, R. S., Turner, W. D., & Zheng, B. (2005). Pulmonary nodule detection with low-dose CT of the lung: agreement among radiologists. AJR. American Journal of Roentgenology, 185(4), 973–978. https://doi.org/10.2214/AJR.04.1225
Lehman, C. D., Wellman, R. D., Buist, D. S. M., Kerlikowske, K., Tosteson, A. N. A., Miglioretti, D. L., & Breast Cancer Surveillance Consortium. (2015). Diagnostic Accuracy of Digital Screening Mammography With and Without Computer-Aided Detection. JAMA Internal Medicine, 175(11), 1828–1837. https://doi.org/10.1001/jamainternmed.2015.5231
Leibig, C., Brehmer, M., Bunk, S., Byng, D., Pinker, K., & Umutlu, L. (2022). Combining the strengths of radiologists and AI for breast cancer screening: a retrospective analysis. The Lancet. Digital Health, 4(7), e507–e519. https://doi.org/10.1016/ S2589-7500(22)00070-X
Leitlinienprogramm Onkologie: Prostatakarzinom. (n.d.). Retrieved July 8, 2023, from https://www.leitlinienprogrammonkologie. de/index.php?id=58&type=0
Litjens, G. J. S., Barentsz, J. O., Karssemeijer, N., & Huisman, H. J. (2015). Clinical evaluation of a computer-aided diagnosis system for determining cancer aggressiveness in prostate MRI. European Radiology, 25(11), 3187–3199. https://doi.org/10.1007/ s00330-015-3743-y
Lu, M. T., Raghu, V. K., Mayrhofer, T., Aerts, H. J. W. L., & Hoffmann, U. (2020). Deep Learning Using Chest Radiographs to Identify High-Risk Smokers for Lung Cancer Screening Computed Tomography: Development and Validation of a Prediction Model. Annals of Internal Medicine, 173(9), 704–713. https://doi.org/10.7326/M20-1868
Lung cancer: Screening. (2021, March 9). US Preventive Services Taskforce. https://www.uspreventiveservicestaskforce.org/uspstf/recommendation/lung-cancer-screening
Marmot, M. G., Altman, D. G., Cameron, D. A., Dewar, J. A., Thompson, S. G., & Wilcox, M. (2013). The benefits and harms of breast cancer screening: an independent review. British Journal of Cancer, 108(11), 2205–2240. https://doi.org/10.1038/ bjc.2013.177
Marrero, J. A., Kulik, L. M., Sirlin, C. B., Zhu, A. X., Finn, R. S., Abecassis, M. M., Roberts, L. R., & Heimbach, J. K. (2018). Diagnosis, Staging, and Management of Hepatocellular Carcinoma: 2018 Practice Guidance by the American Association for the Study of Liver Diseases. Hepatology, 68(2), 723–750. https://doi.org/10.1002/hep.29913
McKinney, S. M., Sieniek, M., Godbole, V., Godwin, J., Antropova, N., Ashrafian, H., Back, T., Chesus, M., Corrado, G. S., Darzi, A., Etemadi, M., Garcia-Vicente, F., Gilbert, F. J., Halling-Brown, M., Hassabis, D., Jansen, S., Karthikesalingam, A., Kelly, C. J., King, D., … Shetty, S. (2020). International evaluation of an AI system for breast cancer screening. Nature, 577(7788), 89–94. https://doi.org/10.1038/s41586-019-1799-6
Merriel, S. W. D., Pocock, L., Gilbert, E., Creavin, S., Walter, F. M., Spencer, A., & Hamilton, W. (2022). Systematic review and meta-analysis of the diagnostic accuracy of prostatespecific antigen (PSA) for the detection of prostate cancer in symptomatic patients. BMC Medicine, 20(1), 54. https://doi.org/10.1186/s12916-021-02230-y
Mikhael, P. G., Wohlwend, J., Yala, A., Karstens, L., Xiang, J., Takigami, A. K., Bourgouin, P. P., Chan, P., Mrah, S., Amayri, W., Juan, Y.-H., Yang, C.-T., Wan, Y.-L., Lin, G., Sequist, L. V., Fintelmann, F. J., & Barzilay, R. (2023). Sybil: A Validated Deep Learning Model to Predict Future Lung Cancer Risk From a Single Low-Dose Chest Computed Tomography.Journal of Clinical Oncology: Official Journal of the American Society of Clinical Oncology, 41(12), 2191–2200. https://doi.org/10.1200/ JCO.22.01345
Moawad, F. J., Maydonovitch, C. L., Cullen, P. A., Barlow, D.S., Jenson, D. W., & Cash, B. D. 2010). CT colonography may improve colorectal cancer screening compliance. AJR. American Journal of Roentgenology, 195(5), 1118–1123. https://doi.org/10.2214/AJR.10.4921
Moran, S., & Warren-Forward, H. (2012). The Australian BreastScreen workforce: a snapshot. The Radiographer, 59(1), 26–30. https://doi.org/10.1002/j.2051-3909.2012.tb00169.x
Mottet, N., Bellmunt, J., Bolla, M., Briers, E., Cumberbatch, M. G., De Santis, M., Fossati, N., Gross, T., Henry, A. M., Joniau, S., Lam, T. B., Mason, M. D., Matveev, V. B., Moldovan, P. C., van den Bergh, R. C. N., Van den Broeck, T., van der Poel, H. G., van der Kwast, T. H., Rouvière, O., … Cornford, P. (2017). EAU-ESTRO-SIOG Guidelines on Prostate Cancer. Part 1: Screening, Diagnosis, and Local Treatment with Curative Intent. European Urology 71(4), 618–629. https://doi.org/10.1016/j.eururo.2016.08.003
Muller, B. G., Shih, J. H., Sankineni, S., Marko, J., Rais-Bahrami, S., George, A. K., de la Rosette, J. J. M. C. H., Merino, M. J., Wood, B. J., Pinto, P., Choyke, P. L., & Turkbey, B. (2015). Prostate Cancer: Interobserver Agreement and Accuracy with the Revised Prostate Imaging Reporting and Data System at Multiparametric MR Imaging. Radiology, 277(3), 741–750. https://doi.org/10.1148/radiol.2015142818
National Lung Screening Trial Research Team, Aberle, D. R., Adams, A. M., Berg, C. D., Black, W. C., Clapp, J. D., Fagerstrom, R. M., Gareen, I. F., Gatsonis, C., Marcus, P.M., & Sicks, J. D. (2011). Reduced lung-cancer mortality with low-dose computed tomographic screening. The New England Journal of Medicine, 365(5), 395–409. https://doi.org/10.1056/ NEJMoa1102873
NHS England. (2022). Targeted screening for lung cancer with low radiation dose computed tomography Standard protocol prepared for the Targeted Lung Health Checks Programme. https://www.england.nhs.uk/publication/targeted-screening-for-lung-cancer
OECD. (2012). Screening, survival and mortality for colorectal cancer.In Health at a Glance: Europe 2012 (pp. 110–111). OECD. https://doi.org/10.1787/9789264183896-48-en
Overview | Prostate cancer: diagnosis and management | Guidance | NICE. (n.d.). Retrieved July 8, 2023, from https://www.nice.org.uk/guidance/ng131
Park, H. J., Choi, B. I., Lee, E. S., Park, S. B., & Lee, J. B. (2017). How to Differentiate Borderline Hepatic Nodules in Hepatocarcinogenesis: Emphasis on Imaging Diagnosis. Liver Cancer, 6(3), 189–203. https://doi.org/10.1159/000455949
Penzkofer, T., Tuncali, K., Fedorov, A., Song, S.-E., Tokuda, J., Fennessy, F. M., Vangel, M. G., Kibel, A. S., Mulkern, R. V., Wells, W. M., Hata, N., & Tempany, C. M. C. (2015). Transperineal in-bore 3-T MR imaging-guided prostate biopsy: a prospective clinical observational study.Radiology, 274(1), 170–180. https://doi.org/10.1148/radiol.14140221
Pickhardt, P. J., Choi, J. R., Hwang, I., Butler, J. A., Puckett, M.L., Hildebrandt, H. A., Wong, R. K., Nugent, P. A., Mysliwiec, P. A., & Schindler, W. R. (2003). Computed tomographic virtual colonoscopy to screen for colorectal neoplasia in asymptomatic adults.The New England Journal of Medicine, 349(23), 2191–2200. https://doi.org/10.1056/NEJMoa031618
Pickhardt, P. J., Correale, L., Delsanto, S., Regge, D., & Hassan, C. (2018). CT Colonography Performance for the Detection of Polyps and Cancer in Adults ≥ 65 Years Old: Systematic Review and Meta-Analysis.AJR. American Journal of Roentgenology, 211(1), 40–51. https://doi.org/10.2214/AJR.18.19515
Pickhardt, P. J., Hassan, C., Halligan, S., & Marmo, R. (2011). Colorectal cancer: CT colonography and colonoscopy for detection-systematic review and meta-analysis.Radiology, 259(2), 393–405. https://doi.org/10.1148/radiol.11101887
Raya-Povedano, J. L., Romero-Martín, S., Elías-Cabot, E., Gubern-Mérida, A., Rodríguez-Ruiz, A., & Álvarez-Benito, M. (2021). AI-based Strategies to Reduce Workload in Breast Cancer Screening with Mammography and Tomosynthesis: A Retrospective Evaluation. Radiology, 300(1), 57–65. https://doi. org/10.1148/radiol.2021203555
Reddy, S., Allan, S., Coghlan, S., & Cooper, P. (2020). A governance model for the application of AI in health care. Journal of the American Medical Informatics Association: JAMIA, 27(3), 491–497. https://doi.org/10.1093/jamia/ocz192
Ren, W., Chen, M., Qiao, Y., & Zhao, F. (2022). Global guidelines for breast cancer screening: A systematic review.Breast, 64,85–99. https://doi.org/10.1016/j.breast.2022.04.003
Reyes, M., Meier, R., Pereira, S., Silva, C. A., Dahlweid, F.-M., von Tengg-Kobligk, H., Summers, R. M., & Wiest, R. (2020). On the Interpretability of Artificial Intelligence in Radiology: Challenges and Opportunities. Radiology.Artificial Intelligence, 2(3), e190043. https://doi.org/10.1148/ryai.2020190043
Rimmer, A. (2017). Radiologist shortage leaves patient care at risk, warns royal college.BMJ, 359, j4683. https://doi.org/10.1136/bmj.j4683
Ristvedt, S. L., McFarland, E. G., Weinstock, L. B., & Thyssen, E. P. (2003). Patient preferences for CT colonography, conventional colonoscopy, and bowel preparation. The American Journal of Gastroenterology, 98(3), 578–585. https://doi.org/10.1016/S0002-9270(02)06024-0
Rosenkrantz, A. B., Ayoola, A., Hoffman, D., Khasgiwala, A., Prabhu, V., Smereka, P., Somberg, M., & Taneja, S. S. (2017). The Learning Curve in Prostate MRI Interpretation: Self-Directed Learning Versus Continual Reader Feedback. AJR. American Journal of Roentgenology, 208(3), W92–W100. https://doi.org/10.2214/AJR.16.16876
Saha, A., Hosseinzadeh, M., & Huisman, H. (2021). End-to-end prostate cancer detection in bpMRI via 3D CNNs: Effects of attention mechanisms, clinical priori and decoupled false positive reduction. Medical Image Analysis, 73, 102155. https://doi.org/10.1016/j.media.2021.102155
Sanford, T. H., Zhang, L., Harmon, S. A., Sackett, J., Yang, D., Roth, H., Xu, Z., Kesani, D., Mehralivand, S., Baroni, R.H., Barrett, T., Girometti, R., Oto, A., Purysko, A. S., Xu, S., Pinto, P. A., Xu, D., Wood, B. J., Choyke, P. L., & Turkbey, B. (2020). Data Augmentation and Transfer Learning to Improve Generalizability of an Automated Prostate Segmentation Model. AJR. American Journal of Roentgenology, 215(6), 1403–1410. https://doi.org/10.2214/AJR.19.22347
Sato, M., Kobayashi, T., Soroida, Y., Tanaka, T., Nakatsuka,T., Nakagawa, H., Nakamura, A., Kurihara, M., Endo, M., Hikita, H., Sato, M., Gotoh, H., Iwai, T., Tateishi, R., Koike,K., & Yatomi, Y. (2022). Development of novel deep multimodal representation learning-based model for the differentiation of liver tumors on B-mode ultrasound images. Journal of Gastroenterology and Hepatology, 37(4), 678–684, https://doi.org/10.1111/jgh.15763
Schmauch, B., Herent, P., Jehanno, P., Dehaene, O., Saillard, C., Aubé, C., Luciani, A., Lassau, N., & Jégou, S. (2019). Diagnosis of focal liver lesions from ultrasound using deep learning.Diagnostic and Interventional Imaging, 100(4), 227–233. https://doi.org/10.1016/j.diii.2019.02.009
Siddiqui, M. M., Rais-Bahrami, S., Turkbey, B., George, A. K., Rothwax, J., Shakir, N., Okoro, C., Raskolnikov, D., Parnes, H.L., Linehan, W. M., Merino, M. J., Simon, R. M., Choyke, P. L., Wood, B. J., & Pinto, P. A. (2015). Comparison of MR/ultrasound fusion-guided biopsy with ultrasound-guided biopsy for the diagnosis of prostate cancer. JAMA: The Journal of the American Medical Association, 313(4), 390–397. https://doi.org/10.1001/jama.2014.17942
Siegel, R. L., Miller, K. D., Fuchs, H. E., & Jemal, A. (2021). Cancer Statistics, 2021. CA: A Cancer Journal for Clinicians, 71(1), 7–33. https://doi.org/10.3322/caac.21654
Singal, A. G., Zhang, E., Narasimman, M., Rich, N. E., Waljee, A. K., Hoshida, Y., Yang, J. D., Reig, M., Cabibbo, G., Nahon, P., Parikh, N. D., & Marrero, J.A. (2022). HCC surveillance improves early detection, curative treatment receipt, and survival in patients with cirrhosis: A meta-analysis Journal of Hepatology, 77(1), 128–139. https://doi.org/10.1016/j.jhep.2022.01.023
Smith, C. P., Harmon, S. A., Barrett, T., Bittencourt, L. K., Law, Y. M., Shebel, H., An, J. Y., Czarniecki, M., Mehralivand, S.,Coskun, M., Wood, B. J., Pinto, P. A., Shih, J. H., Choyke, P. L., & Turkbey, B. (2019). Intra- and interreader reproducibility of PI-RADSv2: A multireader study. Journal of Magnetic Resonance Imaging: JMRI, 49(6), 1694–1703. https://doi.org/10.1002/jmri.26555
Smyth, A. E., Healy, C. F., MacMathuna, P., & Fenlon, H. M. (2013). REVIEW OF CT COLONOGRAPHY: REAL-LIFE EXPERIENCE OF ONE THOUSAND CASES IN A TERTIARY REFERRAL CENTRE. Gut, 62(Suppl 2), A15–A15. https://doi.org/10.1136/gutjnl-2013-305143.36
Song, B., Zhang, G., Lu, H., Wang, H., Zhu, W., J Pickhardt, P., & Liang, Z. (2014). Volumetric texture features from higher-order images for diagnosis of colon lesions via CT colonography. International Journal of Computer Assisted Radiology and Surgery, 9(6), 1021–1031. https://doi.org/10.1007/s11548-014-0991-2
Sonn, G. A., Chang, E., Natarajan, S., Margolis, D. J., Macairan, M., Lieu, P., Huang, J., Dorey, F. J., Reiter, R.E., & Marks, L. S. (2014). Value of targeted prostate biopsy using magnetic resonance-ultrasound fusion in men with prior negative biopsy and elevated prostate-specific antigen. European Urology, 65(4), 809–815. https://doi.org/10.1016/j.eururo.2013.03.025
Stock, C., Ihle, P., Schubert, I., & Brenner, H. (2011). Colonoscopy and fecal occult blood test use in Germany: results from a large insurance-based cohort. Endoscopy, 43(9), 771–781. https://doi.org/10.1055/s-0030-1256504
Sung, H., Ferlay, J., Siegel, R. L., Laversanne, M., Soerjomataram, I., Jemal, A., & Bray, F. (2021). Global Cancer Statistics 2020: GLOBOCAN Estimates of Incidence and Mortality Worldwide for 36 Cancers in 185 Countries. CA: A Cancer Journal for Clinicians, 71(3), 209–249. https://doi.org/10.3322/caac.21660
Sunoqrot, M. R. S., Saha, A., Hosseinzadeh, M., Elschot, M., & Huisman, H. (2022). Artificial intelligence for prostate MRI: open datasets, available applications, and grand challenges.European Radiology Experimental, 6(1), 35. https://doi.org/10.1186/s41747-022-00288-8
Tabár, L., Vitak, B., Chen, T. H.-H., Yen, A. M.-F., Cohen, A., Tot, T., Chiu, S. Y.-H., Chen, S. L.-S., Fann, J. C.-Y., Rosell, J., Fohlin, H., Smith, R. A., & Duffy, S. W. (2011). Swedish two-county trial: impact of mammographic screening on breast cancer mortality during 3 decades. Radiology, 260(3), 658–663. https://doi.org/10.1148/radiol.11110469
Ta, C. N., Kono, Y., Eghtedari, M., Oh, Y. T., Robbin, M. L., Barr, R. G., Kummel, A. C., & Mattrey, R. F. (2018). Focal Liver Lesions: Computer-aided Diagnosis by Using Contrast-enhanced US Cine Recordings. Radiology, 286(3), 1062–1071. https://doi.org/10.1148/radiol.2017170365
The burden of cancer. (n.d.). The Cancer Atlas. Retrieved July 8, 2023, from https://canceratlas.cancer.org/the-burden/theburden-of-cancer/
Tiyarattanachai, T., Apiparakoon, T., Marukatat, S., Sukcharoen, S., Yimsawad, S., Chaichuen, O., Bhumiwat, S., Tanpowpong, N., Pinjaroen, N., Rerknimitr, R., & Chaiteerakij, R. (2022). The feasibility to use artificial intelligence to aid detecting focal liver lesions in real-time ultrasound: a preliminary study based on videos. Scientific Reports 12(1), 7749. https://doi.org/10.1038/s41598-022-11506-z
Turco, S., Tiyarattanachai, T., Ebrahimkheil, K., Eisenbrey, J., Kamaya, A., Mischi, M., Lyshchik, A., & Kaffas, A. E. (2022). Interpretable Machine Learning for Characterization of Focal Liver Lesions by Contrast-Enhanced Ultrasound. IEEE Transactions on Ultrasonics, Ferroelectrics, and Frequency Control, 69(5), 1670–1681. https://doi.org/10.1109/TUFFC.2022.3161719
Turkbey, B., & Haider, M. A. (2022). Deep learning-based artificial intelligence applications in prostate MRI: brief summary. The British Journal of Radiology, 95(1131), 20210563. https://doi.org/10.1259/bjr.20210563
Twilt, J. J., van Leeuwen, K. G., Huisman, H. J., Fütterer, J. J., & de Rooij, M. (2021). Artificial Intelligence Based Algorithms for Prostate Cancer Classification and Detection on Magnetic Resonance Imaging: A Narrative Review.Diagnostics (Basel, Switzerland), 11(6). https://doi.org/10.3390/diagnostics11060959
Tzartzeva, K., Obi, J., Rich, N. E., Parikh, N. D., Marrero, J.A., Yopp, A., Waljee, A. K., & Singal, A. G. (2018). Surveillance Imaging and Alpha Fetoprotein for Early Detection of Hepatocellular Carcinoma in Patients With Cirrhosis: A Meta-analysis. Gastroenterology, 154(6), 1706–1718.e1. https://doi.org/10.1053/j.gastro.2018.01.064
Use of colorectal cancer screening tests. (2023, March 31). https://www.cdc.gov/cancer/colorectal/statistics/use-screeningtests-BRFSS.htm
Ushinsky, A., Bardis, M., Glavis-Bloom, J., Uchio, E., Chantaduly, C., Nguyentat, M., Chow, D., Chang, P. D., & Houshyar, R. (2021). A 3D-2D Hybrid U-Net Convolutional Neural Network Approach to Prostate Organ Segmentation of Multiparametric MRI.AJR. American Journal of Roentgenology, 216(1), 111–116. https://doi.org/10.2214/AJR.19.22168
US Preventive Services Task Force, Grossman, D. C., Curry, S. J., Owens, D. K., Bibbins-Domingo, K., Caughey, A. B., Davidson, K. W., Doubeni, C. A., Ebell, M., Epling, J. W., Jr, Kemper, A. R., Krist, A. H., Kubik, M., Landefeld, C. S., Mangione, C. M., Silverstein, M., Simon, M. A., Siu, A. L., & Tseng, C.-W. (2018). Screening for Prostate Cancer: US Preventive Services Task Force Recommendation Statement. JAMA: The Journal of the American Medical Association, 319(18), 1901–1913. https://doi.org/10.1001/jama.2018.3710
van Leeuwen, K. G., Schalekamp, S., Rutten, M. J. C. M., van Ginneken, B., & de Rooij, M. (2021). Artificial intelligence in radiology: 100 commercially available products and their scientific evidence. European Radiology, 31(6), 3797–3804. https://doi.org/10.1007/s00330-021-07892-z
Vayena, E., Blasimme, A., & Cohen, I. G. (2018). Machine learning in medicine: Addressing ethical challenges.PLoS Medicine, 15(11), e1002689. https://doi.org/10.1371/journal.pmed.1002689
Virmani, J., Kumar, V., Kalra, N., & Khandelwal, N. (2014).Neural network ensemble based CAD system for focal liver lesions from B-mode ultrasound. Journal of Digital Imaging, 27(4), 520–537. https://doi.org/10.1007/s10278-014-9685-0
Vogel, A., Meyer, T., Sapisochin, G., Salem, R., & Saborowski, A. (2022). Hepatocellular carcinoma.The Lancet, 400(10360), 1345–1362. https://doi.org/10.1016/S0140-6736(22)01200-4
Walker, S. M., Choyke, P. L., & Turkbey, B. (2020). What You Need to Know Before Reading Multiparametric MRI for Prostate Cancer. AJR. American Journal of Roentgenology, 214(6), 1211–1219. https://doi.org/10.2214/AJR.19.22751
Wang, B., Lei, Y., Tian, S., Wang, T., Liu, Y., Patel, P., Jani, A.B., Mao, H., Curran, W. J., Liu, T., & Yang, X. (2019). Deeply supervised 3D fully convolutional networks with group dilated convolution for automatic MRI prostate segmentation. Medical Physics, 46(4), 1707–1718. https://doi.org/10.1002/mp.13416
Wang, Y., Midthun, D. E., Wampfler, J. A., Deng, B., Stoddard, S. M., Zhang, S., & Yang, P. (2015). Trends in the proportion of patients with lung cancer meeting screening criteria. JAMA: The Journal of the American Medical Association, 313(8), 853–855. https://doi.org/10.1001/jama.2015.413
Welch, H. G., & Albertsen, P. C. (2020). Reconsidering Prostate Cancer Mortality - The Future of PSA Screening. The New England Journal of Medicine, 382(16), 1557–1563. https://doi.org/10.1056/NEJMms1914228
Wesp, P., Grosu, S., Graser, A., Maurus, S., Schulz, C., Knösel, T., Fabritius, M. P., Schachtner, B., Yeh, B. M., Cyran, C. C., Ricke, J., Kazmierczak, P. M., & Ingrisch, M. (2022). Deep learning in CT colonography: differentiating premalignant from benign colorectal polyps. European Radiology, 32(7), 4749–4759. https://doi.org/10.1007/s00330-021-08532-2
Westphalen, A. C., McCulloch, C. E., Anaokar, J. M., Arora, S., Barashi, N. S., Barentsz, J. O., Bathala, T. K., Bittencourt, L. K., Booker, M. T., Braxton, V. G., Carroll, P. R., Casalino, D. D., Chang, S. D., Coakley, F. V., Dhatt, R., Eberhardt, S. C., Foster, B. R., Froemming, A. T., Fütterer, J. J., … Rosenkrantz, A. B. (2020). Variability of the Positive Predictive Value of PI-RADS for Prostate MRI across 26 Centers: Experience of the Society of Abdominal Radiology Prostate Cancer Diseasefocused Panel. Radiology, 296(1), 76–84. https://doi.org/10.1148/radiol.2020190646
Wing, P., & Langelier, M. H. (2009). Workforce shortages in breast imaging: impact on mammography utilization.AJR. American Journal of Roentgenology, 192(2), 370–378. https://doi.org/10.2214/AJR.08.1665
Winkel, D. J., Tong, A., Lou, B., Kamen, A., Comaniciu, D., Disselhorst, J. A., Rodríguez-Ruiz, A., Huisman, H., Szolar, D., Shabunin, I., Choi, M. H., Xing, P., Penzkofer, T., Grimm, R., von Busch, H., & Boll, D. T. (2021). A Novel Deep Learning Based Computer-Aided Diagnosis System Improves the Accuracy and Efficiency of Radiologists in Reading Biparametric Magnetic Resonance Images of the Prostate: Results of a Multireader, Multicase Study. Investigative Radiology, 56(10), 605–613. https://doi.org/10.1097/RLI.0000000000000780
World Health Organization. Regional Office for Europe. (2022). A short guide to cancer screening: increase effectiveness, maximize benefits and minimize harm. World Health Organization. Regional Office for Europe. https://apps.who.int/iris/handle/10665/351396
Zauber, A. G., Winawer, S. J., O’Brien, M. J., Lansdorp-Vogelaar, I., van Ballegooijen, M., Hankey, B. F., Shi, W., Bond, J. H., Schapiro, M., Panish, J. F., Stewart, E. T., & Waye, J. D. (2012). Colonoscopic polypectomy and long-term prevention of colorectal-cancer deaths. The New England Journal of Medicine, 366(8), 687–696. https://doi.org/10.1056/NEJMoa1100370
Zhang, B.-H., Yang, B.-H., & Tang, Z.-Y. (2004). Randomized controlled trial of screening for hepatocellular carcinoma. Journal of Cancer Research and Clinical Oncology, 130(7), 417–422. https://doi.org/10.1007/s00432-004-0552-0