- CCTA
- CAD
- Coronary Stenosis
- Cardiac
Improving Coronary Stenosis Assessment with DL-FFRCT
Conventional diagnostic coronary angiography is inaccurate in assessing the functional significance of a coronary stenosis when compared with the FFR, not only in the 50% to 70% category but also in the 70% to 90% angiographic severity category.1
FFRCT can reduce the need for diagnostic coronary angiography when identifying patients suitable for coronary intervention.2
Supporting CAD Functional Evaluation
DEEPVESSEL FFR is a coronary physiological simulation software for the clinical quantitative and qualitative analysis of previously acquired Computed Tomography DICOM data for clinically stable symptomatic patients with coronary artery disease. It provides DVFFR (a CT-derived FFR measurement) computed from static coronary CTA images using deep learning neural networks. Deep Vessel FFR analysis is intended to support the functional evaluation of CAD.
Accuracy. Speed of Computational Results.
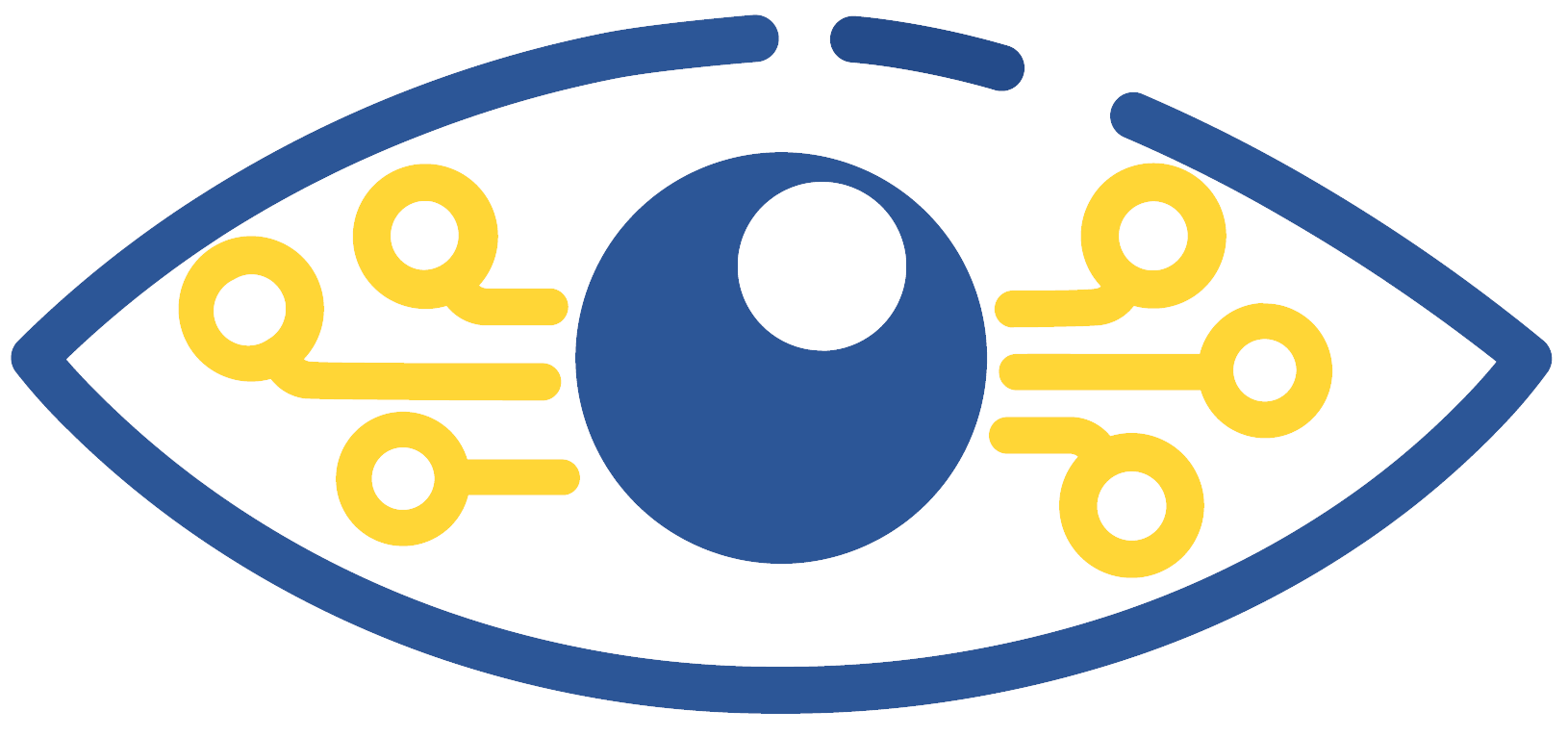
- DVFFR can potentially reduce the false positive rate for myocardial ischemia caused by CTA classification alone.5
- Positive Predictive Value and Negative Predictive Values for ischemia above 95% at the per patient level and 85% at the per vessel level.6
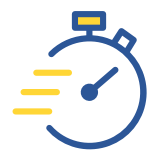
- The computation time for the CT-FFR step is still very fast, with the maximum time of 27.9 s on a GPU workstation (Nvidia GTX1080 GPU).5
1 Tonino PA, Fearon WF, De Bruyne B, Oldroyd KG, Leesar MA, Ver Lee PN, Maccarthy PA, Van't Veer M, Pijls NH. Angiographic versus functional severity of coronary artery stenoses in the FAME study fractional flow reserve versus angiography in multivessel evaluation. J Am Coll Cardiol. 2010 Jun 22;55(25):2816-21. doi: 10.1016/j.jacc.2009.11.096. PMID: 20579537.
2 Liu, X., Mo, X., Zhang, H. et al. A 2-year investigation of the impact of the computed tomography–derived fractional flow reserve calculated using a deep learning algorithm on routine decision-making for coronary artery disease management. Eur Radiol 31, 7039–7046 (2021). https://doi.org/10.1007/s00330-021- 07771-7
3 Li Y, Qiu H, Hou Z, et al. Additional value of deep learning computed tomographic angiography-based fractional flow reserve in detecting coronary stenosis and predicting outcomes. Acta Radiologica. 2022;63(1):133-140. doi:10.1177/0284185120983977
4 Liu, X., Mo, X., Zhang, H. et al. A 2-year investigation of the impact of the computed tomography–derived fractional flow reserve calculated using a deep learning algorithm on routine decision-making for coronary artery disease management. Eur Radiol 31, 7039–7046 (2021).
5 Li Y, Qiu H, Hou Z, Zheng J, Li J, Yin Y, Gao R. Additional value of deep learning computed tomographic angiography-based fractional flow reserve in detecting coronary stenosis and predicting outcomes. Acta Radiol. 2022 Jan;63(1):133-140. doi: 10.1177/0284185120983977. Epub 2021 Jan 10. PMID: 33423530.
6 Wang ZQ, Zhou YJ, Zhao YX, Shi DM, Liu YY, Liu W, Liu XL, Li YP. Diagnostic accuracy of a deep learning approach to calculate FFR from coronary CT angiography. J Geriatr Cardiol. 2019 Jan;16(1):42-48. doi: 10.11909/j.issn.1671-5411.2019.01.010. PMID: 30800150; PMCID: PMC6379239.
7 Gulati M, Levy PD, Mukherjee D, Amsterdam E, Bhatt DL, Birtcher KK, Blankstein R, Boyd J, Bullock-Palmer RP, Conejo T, Diercks DB, Gentile F, Greenwood JP, Hess EP, Hollenberg SM, Jaber WA, Jneid H, Joglar JA, Morrow DA, O'Connor RE, Ross MA, Shaw LJ. 2021 AHA/ACC/ASE/CHEST/SAEM/SCCT/SCM R Guideline for the Evaluation and Diagnosis of Chest Pain: A Report of the American College of Cardiology/American Heart Association Joint Committee on Clinical Practice Guidelines. Circulation. 2021 Nov 30;144(22):e368-e454. doi: 10.1161/CIR.0000000000001029. Epub 2021 Oct 28. Erratum in: Circulation. 2021 Nov 0;144(22):e455. Erratum in: Circulation. 2023 Dec 12;148(24):e281. PMID: 34709879.
Currently not available within the Calantic Viewer.