This article summarizes the findings of the scientific paper titled “Unlocking the Value: Quantifying the ROI of Hospital AI” published in the Journal of the American College of Radiology on March 24, 2024.
Key Learnings
- A comprehensive and multi-dimensional return-on-investment (ROI) calculator was designed to assess both monetary and non-monetary benefits of a platform for AI-powered digital imaging applications.
- Relative to current practices, the calculator showed a reduction of 16 days of waiting time and 78 days of triage time (through scans reprioritization), 10 days of reading time, and 41 days of reporting time, totalling 145 days saved overall if the platform was to be used over a time period of five years.
- The calculator showed potential revenue benefits for hospitals that could bring in patient for clinically beneficial follow up scans, hospitalizations, and treatment procedures.
The Impact of AI in Radiology
Artificial intelligence (AI) and machine learning algorithms have demonstrated the potential to transform the radiology workflow by helping to reduce image acquisition time, enhance diagnostic confidence, and support precision medicine.1,2,3,4
AI algorithms are increasingly enhancing or, in some cases, outperforming human experts in certain tasks and this can lead to higher accuracy in radiologist assessments when augmented by AI.5,6,7,8,9,10
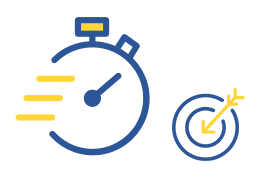
These advancements have the potential to translate into patient-centred benefits, such as shorter waiting times, quicker access to results, earlier disease detection, and improved prognoses.11
Healthcare decision-makers are increasingly keen to take advantage of the benefits offered by AI. In a recent survey of 201 US healthcare provider executives, 56% indicated that software and technology were among their top three strategic priorities and 75% expected to increase spending on software and technology in the subsequent year.12 To date, however, AI research has focused primarily on the accuracy of algorithms, with little evidence measuring the impact of these innovations on patient- and physician-relevant outcomes or the broader benefits to the setting within which they are deployed.13,14,15
There is an urgent need for calculators which capture and clearly communicate the benefits that radiology AI solutions bring to the various stakeholders within the healthcare delivery environment.16
The Need for Comprehensive Evaluation Tools
The development of a multi-dimensional return-on-investment (ROI) calculator for a platform of AI-powered radiology digital imaging applications addresses the urgent need for tools that capture and communicate the benefits of radiology AI solutions to various stakeholders within the healthcare delivery environment.
This comprehensive ROI calculator aims to assess both the financial and non-financial benefits of integrating a platform for AI applications into radiology practices, providing decision-makers with valuable insights to guide resource allocation and help ensure financial sustainability.
The ROI Calculator
A central focus of the study was the development of a comprehensive ROI calculator. This tool evaluates the financial and clinical gains potentially achievable through an AI-powered radiology platform. Let’s delve into the key components:
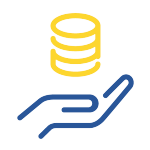
1. Comparative Costs
The calculator considers the costs associated with adoption of the AI platform versus traditional radiology workflows. It accounts for expenses related to AI implementation, training, maintenance, and infrastructure. By juxtaposing these costs, decision-makers gain a clear understanding of the financial implications.
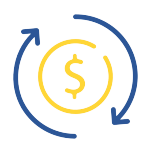
2. Estimated Revenues
The economic impact of AI extends beyond cost savings. The study meticulously examines potential revenue streams resulting from improved diagnostic accuracy, faster turnaround times, and enhanced patient care. These estimated revenues contribute significantly to the overall ROI.
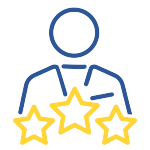
3. Clinical Value
Beyond financial gains, the clinical value of AI cannot be overlooked. AI streamlines workflow, allowing radiologists to focus on complex cases, potentially leading to better patient outcomes.
Scope | Details |
Perspective | US health care provider (including radiologists, referring physicians, IT team, and hospital administrators) from a hospital or diagnostic center, payers, and patients |
Intervention | The Calantic platform (including two service lines and five individual packages) integrated in the radiology workflow. |
Comparator | Current workflow without the use of AI |
Outcomes |
|
Time horizon | 1 and 5 years |
Figure: Scope of ROI Calculator
Findings and Implications
The study shows:
- Monetary ROI: The calculator demonstrates substantial cost savings over a five-year horizon. Based on this paper, hospitals investing in an AI platform may see a significant positive financial impact.
- Non-Monetary ROI: The calculator also identifies the potential for improving patient care, reducing diagnostic errors and enhancing radiologist efficiency contributing to the non-monetary ROI. These non-monetary benefits are also important.
In this paper, the use of an AI platform was found to contribute to time savings in procurement, installation, and maintenance compared to the installation of single digital applications.
The introduction of an AI platform into the hospital radiology workflow resulted in labor time reductions and delivery of an ROI of 451% over a five-year period compared to a workflow without the use of AI. The calculator showed potential revenue benefits for hospitals that could result in additional clinically beneficial follow-up scans, hospitalizations, and treatment procedures.
Conclusion and Future Implications
The study provided quantitative evidence of the financial and non-financial benefits that AI integration in radiology can potentially generate, while offering a comprehensive and evidence-based calculator for assessing the financial viability and value proposition of integrating AI platform technology into radiology practices. Each hospital's results will differ based on their input data.
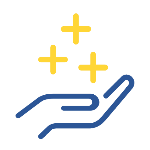
The ROI calculator serves as a valuable tool to assist healthcare decision-making in terms of AI purchasing and investment, providing insights into potential financial advantages specific to each organization.
References
- Yu K-H, Beam AL, Kohane IS. Artificial intelligence in healthcare. Nat Biomed Eng. 2018;2(10):719–731. doi: 10.1038/s41551-018-0305-z.
- Davenport T, Kalakota R. The potential for artificial intelligence in healthcare. Future Healthc J. 2019;6(2):94–98. doi: 10.7861/futurehosp.6-2-94.
- Hosny A, Parmar C, Quackenbush J, Schwartz LH, Aerts HJWL. Artificial intelligence in radiology. Nat Rev Cancer. 2018;18(8):500–510. doi: 10.1038/s41568-018-0016-5.
- Aung YYM, Wong DCS, Ting DSW. The promise of artificial intelligence: A review of the opportunities and challenges of artificial intelligence in healthcare. Br Med Bull. 2021;139(1):4–15. doi: 10.1093/bmb/ldab016.
- Langlotz CP. Will artificial intelligence replace radiologists? Radiol Artif Intell.
2019;1(3):e190058. doi: 10.1148/ryai.2019190058. - Reverberi C, Rigon T, Solari A, et al. Experimental evidence of effective human-AI collaboration in medical decision-making. Sci Rep. 2022;12(1):14952. doi:10.1038/s41598-022-18751-2.
- Liu X, Faes L, Kale AU, et al. A comparison of deep learning performance against healthcare professionals in detecting diseases from medical imaging: A systematic review and meta-analysis. Lancet Digit Health. 2019;1(6):e271 e297. doi: 10.1016/S2589-7500(19)30123-2.
- Seah JCY, Tang CHM, Buchlak QD, et al. Effect of a comprehensive deep learning model on the accuracy of chest X-ray interpretation by radiologists: A retrospective, multireader multicase study. Lancet Digit Health. 2021;3(8):e496–e506. doi: 10.1016/S2589-7500(21)00106-0.
- Ahn JS, Ebrahimian S, McDermott S, et al. Association of artificial intelligence aided chest radiograph interpretation with reader performance and efficiency. JAMA Netw Open. 2022;5(8):e2229289. doi:10.1001/jamanetworkopen.2022.29289.
- Sim Y, Chung MJ, Kotter E, et al. Deep convolutional neural network-based software improves radiologist detection of malignant lung nodules on chest radiographs. Radiology. 2020;294(1):199–209. doi:10.1148/radiol.2019182465.
- Choy G, Khalilzadeh O, Michalski M, et al. Current applications and future impact of machine learning in radiology. Radiology. 2018;288(2):318–328. doi: 10.1148/radiol.2018171820
- Feinberg A, Berger E, Hammond R. 2023 Healthcare Provider IT Report: Doubling Down on Innovation. Bain & Company; 2023 Sep. https://www.bain.com/insights/2023-healthcare-provider-it-report-doubling-down-on-innovation/. Accessed November 2024
- van Leeuwen KG, Schalekamp S, Rutten MJCM, van Ginneken B, de Rooij M. Artificial intelligence in radiology: 100 commercially available products and their scientific evidence. Eur Radiol. 2021;31(6):3797–3804. doi: 10.1007/s00330-021-07892-z.
- Wolff J, Pauling J, Keck A, Baumbach J. The economic impact of artificial intelligence in health care: Systematic review. J Med Internet Res. 2020;22(2):e16866. doi: 10.2196/16866.
- Voets MM, Veltman J, Slump CH, Siesling S, Koffijberg H. Systematic review of health economic evaluations focused on artificial intelligence in healthcare: The tortoise and the cheetah. Value Health J Int Soc Pharmacoeconomics Outcomes Res. 2022;25(3):340–349. doi: 10.1016/j.jval.2021.11.1362.
- Sima DM, Esposito G, Van Hecke W, Ribbens A, Nagels G, Smeets D. Health economic impact of software-assisted brain MRI on therapeutic decision-making and outcomes of relapsing-remitting multiple sclerosis patients—A microsimulation study. Brain Sci. 2021;11(12):1570. doi: 10.3390/brainsci11121570.
- Bharadwaj, P. et al (2024). Unlocking the Value: Quantifying the ROI of Hospital AI, JACR