Fundamentals of AI in Radiology
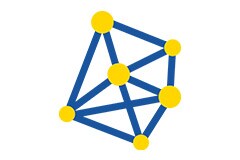
Artificial Intelligence (AI) is revolutionizing various fields, particularly healthcare, by enabling computer systems to perform tasks in a way that simulates human reasoning.
At its core, AI utilizes algorithms - sets of rules or instructions programmed to solve specific problems. These algorithms can analyze vast amounts of data, uncover patterns, and adapt their solutions based on changing circumstances.1
Machine Learning (ML), a type of AI, empowers algorithms to learn from data, improving their performance as they are exposed to more information. One of the most common uses of machine learning is classification - assigning a particular label to a piece of data. ML can be classified into two main categories: supervised learning, where the algorithm is trained on labeled data, and unsupervised learning, where it identifies patterns without explicit instructions. Neural networks, particularly deep neural networks (DNNs), a type of ML, take this a step further by employing multiple layers of interconnected nodes to process complex data, making them particularly effective in the field of radiology.1
Understanding AI Validation Data
For radiologists considering the deployment of AI in their practice, understanding AI performance metrics and validation data is crucial. Several performance metrics exist for assessing how well a model performs certain tasks. Validation data helps assess the performance and reliability of radiology AI solutions. It is essential to ensure that these algorithms can not only analyze existing data but also generalize to new, unseen cases. 1
Performance Metrics
Understanding performance metrics is vital for interpreting AI literature. Multiple performance metrics assess model effectiveness, and no single metric is perfect. Using a combination offers a more comprehensive view of performance.
- For classification tasks, metrics like accuracy, sensitivity, and specificity are often utilized.
- The area under the receiver operating characteristic curve (AUC) is a threshold-independent metric, indicating the likelihood that a random positive example is ranked higher than a random negative example by the algorithm.
- Metrics such as mean absolute error (MAE) and root mean square error (RMSE) are often used to quantitatively describe how well models perform regression tasks.
- In image segmentation tasks, metrics like the Dice similarity coefficient and Hausdorff distance help assess the overlap and distance respectively between predicted and actual regions of interest, which is particularly relevant for AI in medical imaging.1
Internal vs. External Validity
AI models should ideally demonstrate both internal and external validity. Internal validity refers to how well a model performs on the data it was trained on. Performance metrics such as accuracy, sensitivity, specificity, and the area under the receiver operating characteristic curve (AUC) are commonly used to evaluate this aspect. High internal validity indicates that the model can effectively classify or predict outcomes based on its training data.
Conversely, external validity assesses how well the model performs on new data. This is critical in clinical settings, where patient demographics and disease prevalence may vary significantly from the training dataset. The better the model performs on data that differs from data the models were trained and validated on, the higher the external validity. A model that performs well on internal data but poorly on external data may lead to misleading results and potentially harmful patient outcomes.1
Clinical Validation of Algorithms
Clinical validation of AI solutions can be performed retrospectively or prospectively. The prospective approach offers the advantage of assessing the AI's direct impact on radiologists' workflows and user interfaces. However, it is essential to select test cases that reflect the actual patient population, ensuring that the radiology AI model is evaluated under realistic conditions.
Ground truth must be established for each test case, ideally through multi-rater consensus or reference standards from clinical data. Furthermore, the size of the test set should be adequate to ensure robust validation, with recommendations varying based on the specific AI application and AI technique.2
How to choose the right AI App
In the fast-evolving landscape of Radiology Artificial Intelligence, the sheer volume of FDA-cleared healthcare AI applications can be overwhelming. A significant challenge is the lack of transparency regarding performance and validation.
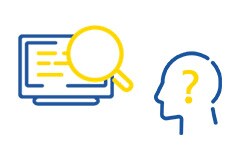
A study revealed that 64% of CE-approved apps were released without peer-reviewed evidence, and those that did often showed only low efficacy.3
Consequently, purchasers must allocate clinical resources to validate performance after implementation. Additionally, an AI algorithm’s return on investment is often unknown or difficult to determine, as improvements in patient outcomes and clinical efficiency are highly dependent on specific use cases.
Curation of AI solutions is one option to navigate this complexity and manage scalable deployment of radiology AI apps.
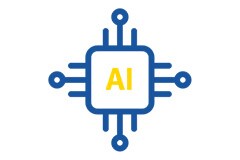
An AI platform can help radiologists identify the most effective algorithms tailored to their specific needs.
Bayer's Calantic AI platform curates applications by first identifying unmet needs through pathology prioritization, mapping patient imaging workflows, and prioritizing pain points. Individual solutions are then assessed for clinical utility. AI solutions that perform well on these criteria are then reviewed by a multidisciplinary team of health economists, radiologists, IT personnel, and business experts for further evaluation.
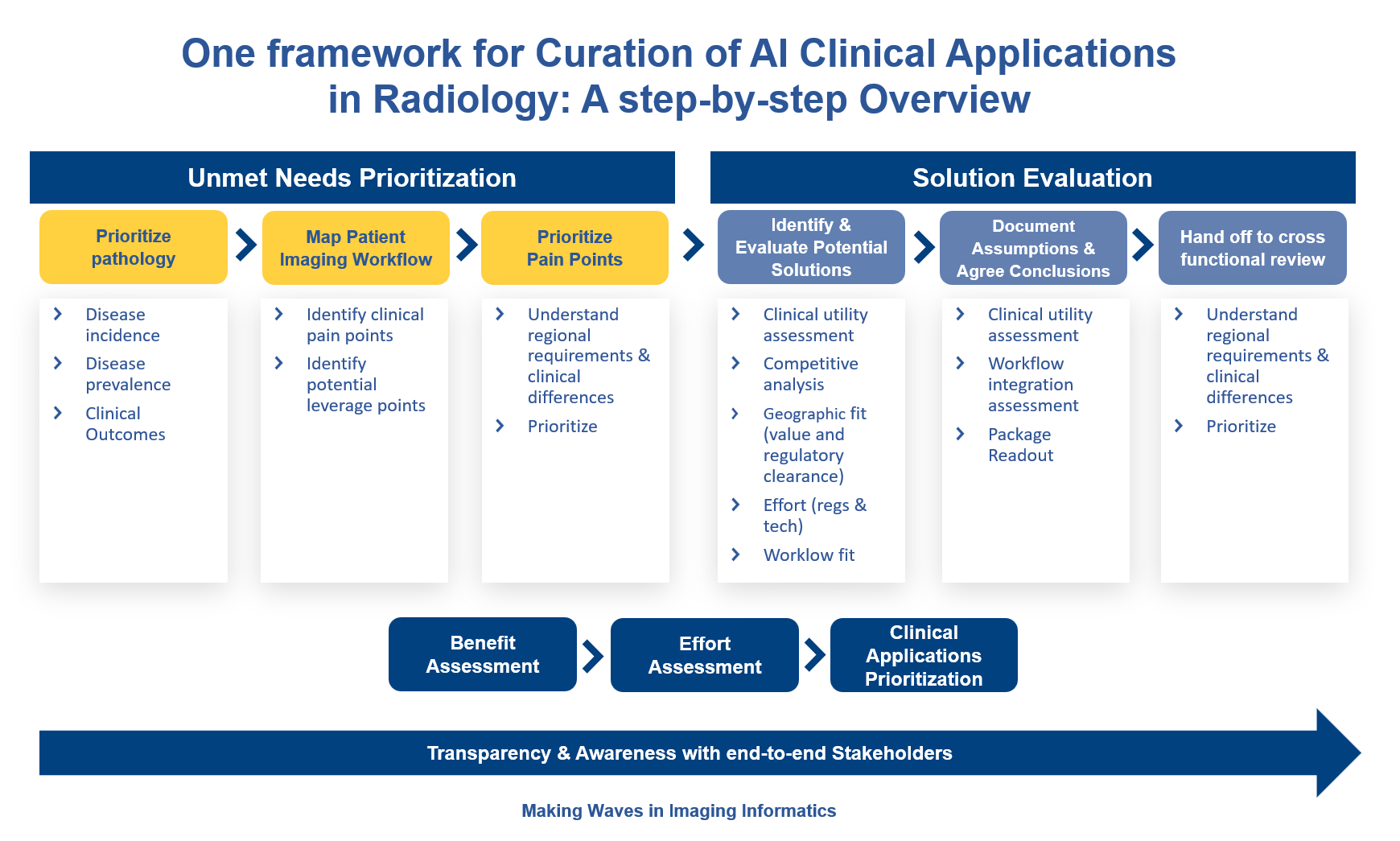
Framework for curation of AI Clinical Application in Radiology
Calantic evaluates an AI application's clinical utility by assessing the disease's clinical burden - considering epidemiology, disease characteristics like duration and severity – along with the frequency of misdiagnosis, the application’s relevance to use cases, its impact on clinical decision-making and healthcare resource utilization.
References
- The Complete Guide to Artificial Intelligence in Radiology, Bayer, 2022
- Tanguay W, Acar P, Fine B et al. Assessment of Radiology Artificial Intelligence Software: A Validation and Evaluation Framework. Can Assoc Radiol J. 2023 May;74(2):326-333.
- Van Leeuwen KG, Schalekamp S, Rutten MJCM et al. Eur Radiol. 2021 Jun;31(6):3797-3804.