- MSK
- Fractures
- Dislocations
Challenges in Detecting Subtle Fractures on Radiograph
Radiographic diagnosis of most fractures and dislocations poses little difficulty to radiologists: however, occasionally these injuries are quite subtle or even impossible to detect on radiographs.1
Missed diagnoses of fracture potentially have important consequences for patients, clinicians, and radiologists.1
Spectrum of fractures in adults potentially missed on plain radiographs: Cervical spine fractures, Thoraco-lumbar spine fractures, Upper extremity fractures, Pelvic ring and lower extremity fractures.1
Fracture Identification Across Age Groups with BoneView 1.1-US
BoneView 1.1-US is intended to analyze radiographs using machine learning techniques to identify and highlight fractures during the review of radiographs of:
Adults (over 21 years) and Children/Adolescents (ages 2 to 21 years):
- Ankle
- Foot
- Knee
- Tibia/Fibula
- Wrist
- Hand
- Elbow
- Forearm
- Humerus
- Shoulder
- Clavicle
Adults (over 21 years) only:
- Pelvis
- Hip
- Femur
- Ribs
- Thoracic Spine
- Lumbosacral Spine
Clinical Impact according to a study5
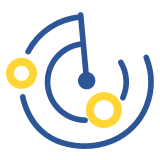
A 20% improvement in per patient fracture detection sensitivity & 60% decrease in patients with false negative findings of fractures in adults and children in a trauma care setting.
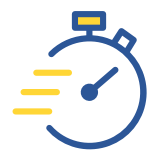
A mean gain in reading time from 10-16 seconds per patient leading to a saving of 16.7 - 26.7 minutes per 100 patients in a trauma care setting.
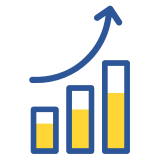
A 10% improvement in NPV value with algorithm use in comparison to an unaided radiologist in a trauma care setting.
1 Pinto A, Berritto D, Russo A, Riccitiello F, Caruso M, Belfiore MP, Papapietro VR, Carotti M, Pinto F, Giovagnoni A, Romano L, Grassi R. Traumatic fractures in adults: missed diagnosis on plain radiographs in the Emergency Department. Acta Biomed. 2018 Jan 19;89(1-S):111-123. doi: 10.23750/abm.v89i1-S.7015. PMID: 29350641; PMCID: PMC6179080.
2 Tveiten L. Implementation of an AI application for fracture detection: Major benefits for patients and healthcare workers. Abstract ECR 2024. Available at: https://dx.doi.org/10.26044/ecr2024/C-23581
3 Regnard NE, Lanseur B, Ventre J, Ducarouge A, Clovis L, Lassalle L et al. Assessment of performances of a deep learning algorithm for the detection of limbs and pelvic fractures, dislocations, focal bone lesions, and elbow effusions on trauma X-rays. European Journal of Radiology 2022; 154:110447. doi: 10.1016/j.ejrad.2022.110447.
4 Hayashi D, Kompel AJ, Ventre J, Ducarouge A, Nguyen T, Regnard NE, Guermazi A. Automated detection of acute appendicular skeletal fractures in pediatric patients using deep learning. Skeletal Radiology. 2022; 51(11):2129-2139. doi: 10.1007/s00256-022-04070-0.
5 Canoni-Meynet L, Verdot P, Danner A,Calame P, Aubry S. Added value of an artificial intelligence solution for fracture detection in the radiologist's daily trauma emergencies workflow. Diagnostic and Interventional Imaging 2022; 103(12):594-600. doi: 10.1016/j.diii.2022.06.004.
Currently not available within the Calantic Viewer.